Porosity Prediction in Selective Laser Melting Combining Photodiode-based In-Process Monitoring and X-CT
e-Journal of Nondestructive Testing(2023)
摘要
Laser powder bed fusion (LPBF) currently faces challenges in consistency, complexity, and cost associated with quality ensurance. In-situ process monitoring is a potential approach to this problem. This work investigates the feasibility of using photodiodes for quality monitoring and correlates photodiode signals with X-ray computed tomography (X-CT) based on deep learning. A cube of Ti-6Al-4V grade 23 was produced with optimum energy density parameters. Porosity was analyzed using X-CT to help understand the size, shape, location, and the number of pores formed. The photodiode signals were recorded using the coaxial melt-pool monitoring system on an in-house developed LPBF machine. The photodiode signals collected during processing were segmented per layer accordingly using different window sizes. The classifiers were trained to predict the presence and location of porosities considering the inter-hatch effect. Moreover, different models were trained and compared, which will act as a baseline for further research. The results showed that using photodiode signals can yield a prediction sensitivity of 68% and an accuracy of 84% for pores with a cross-section larger than 100 μm2, with an area under the receiver operating characteristic curve (AUC) of 0.86. These findings suggest that photodiodes can be a promising approach to quality monitoring in LPBF with sub-layer spatial resolution.
更多查看译文
关键词
porosity prediction,laser,photodiode-based,in-process
AI 理解论文
溯源树
样例
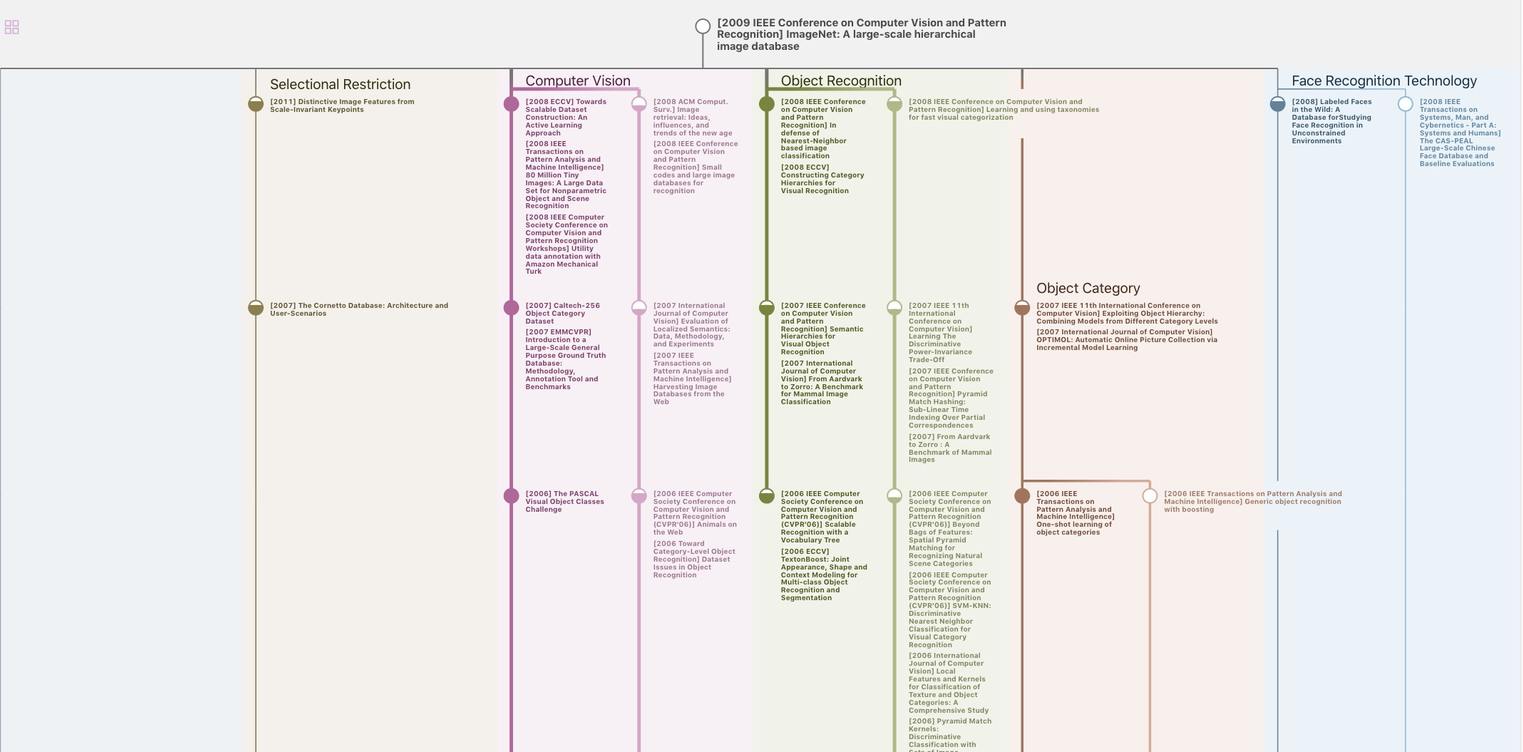
生成溯源树,研究论文发展脉络
Chat Paper
正在生成论文摘要