Knowledge-Prompted Estimator: A Novel Approach to Explainable Machine Translation Assessment
2024 26th International Conference on Advanced Communications Technology (ICACT)(2023)
摘要
Cross-lingual Machine Translation (MT) quality estimation plays a crucial role in evaluating translation performance. GEMBA, the first MT quality assessment metric based on Large Language Models (LLMs), employs one-step prompting to achieve state-of-the-art (SOTA) in system-level MT quality estimation; however, it lacks segment-level analysis. In contrast, Chain-of-Thought (CoT) prompting outperforms one-step prompting by offering improved reasoning and explainability. In this paper, we introduce Knowledge-Prompted Estimator (KPE), a CoT prompting method that combines three one-step prompting techniques, including perplexity, token-level similarity, and sentence-level similarity. This method attains enhanced performance for segment-level estimation compared with previous deep learning models and one-step prompting approaches. Furthermore, supplementary experiments on word-level visualized alignment demonstrate that our KPE method significantly improves token alignment compared with earlier models and provides better interpretability for MT quality estimation. Code will be released upon publication.
更多查看译文
关键词
Machine Translation Quality Estimation Chain-of-Thought Prompting Large Language Models
AI 理解论文
溯源树
样例
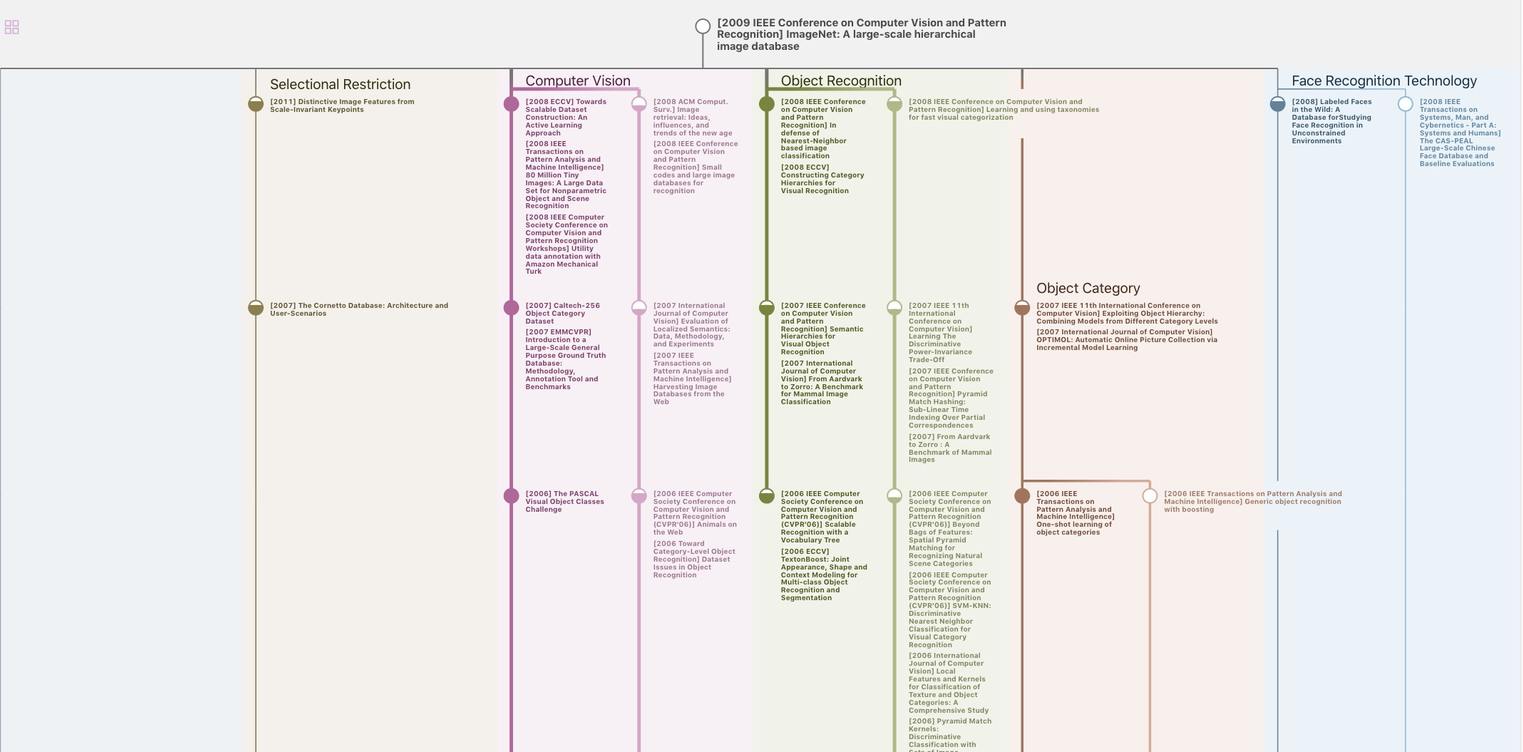
生成溯源树,研究论文发展脉络
Chat Paper
正在生成论文摘要