Confidence-based federated distillation for vision-based lane-centering
CoRR(2023)
摘要
A fundamental challenge of autonomous driving is maintaining the vehicle in the center of the lane by adjusting the steering angle. Recent advances leverage deep neural networks to predict steering decisions directly from images captured by the car cameras. Machine learning-based steering angle prediction needs to consider the vehicle's limitation in uploading large amounts of potentially private data for model training. Federated learning can address these constraints by enabling multiple vehicles to collaboratively train a global model without sharing their private data, but it is difficult to achieve good accuracy as the data distribution is often non-i.i.d. across the vehicles. This paper presents a new confidence-based federated distillation method to improve the performance of federated learning for steering angle prediction. Specifically, it proposes the novel use of entropy to determine the predictive confidence of each local model, and then selects the most confident local model as the teacher to guide the learning of the global model. A comprehensive evaluation of vision-based lane centering shows that the proposed approach can outperform FedAvg and FedDF by 11.3% and 9%, respectively.
更多查看译文
关键词
distillation,confidence-based,vision-based,lane-centering
AI 理解论文
溯源树
样例
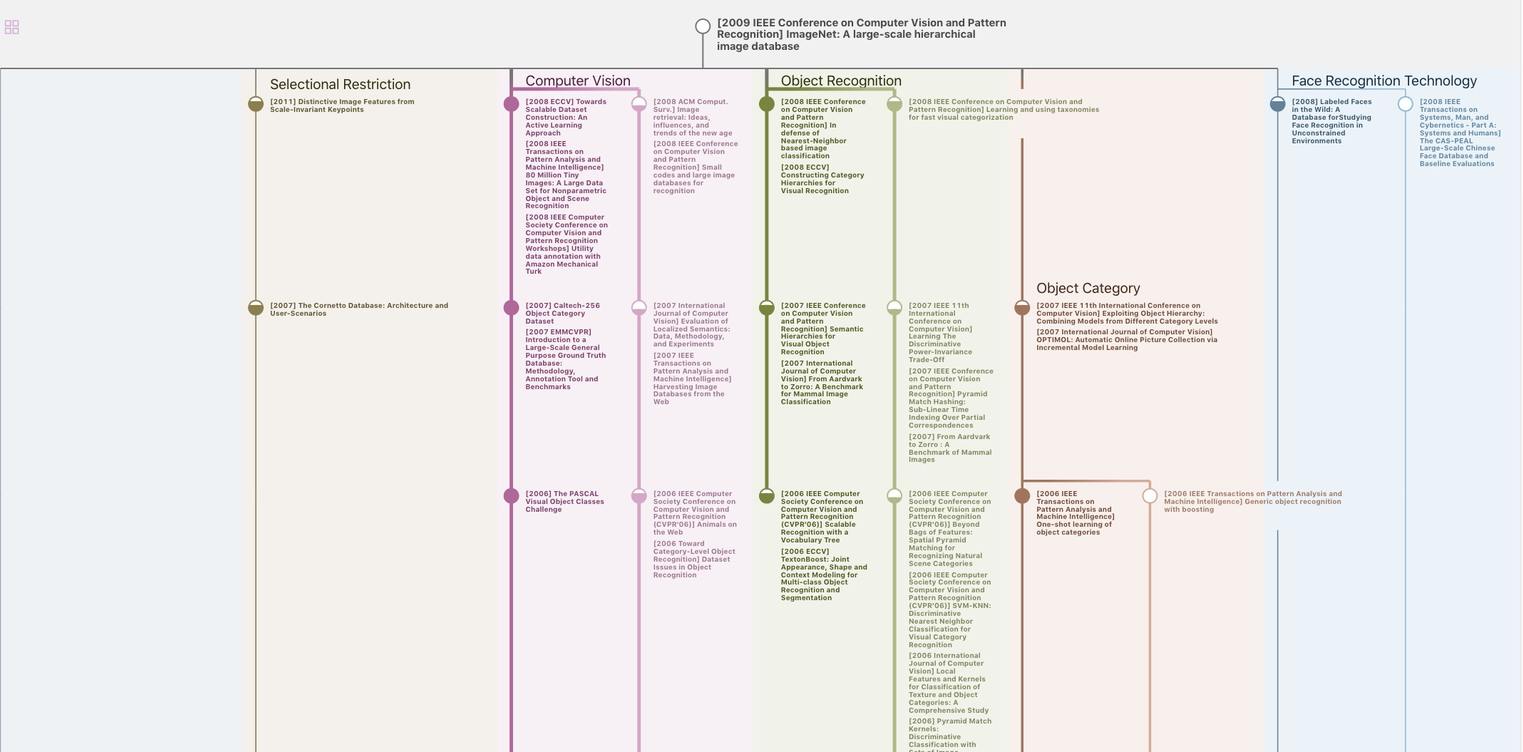
生成溯源树,研究论文发展脉络
Chat Paper
正在生成论文摘要