CT-based deep multi-label learning prediction model for outcome in patients with oropharyngeal squamous cell carcinoma
MEDICAL PHYSICS(2023)
摘要
BackgroundPersonalized treatment is increasingly required for oropharyngeal squamous cell carcinoma (OPSCC) patients due to emerging new cancer subtypes and treatment options. Outcome prediction model can help identify low or high-risk patients who may be suitable to receive de-escalation or intensified treatment approaches. PurposeTo develop a deep learning (DL)-based model for predicting multiple and associated efficacy endpoints in OPSCC patients based on computed tomography (CT). MethodsTwo patient cohorts were used in this study: a development cohort consisting of 524 OPSCC patients (70% for training and 30% for independent testing) and an external test cohort of 396 patients. Pre-treatment CT-scans with the gross primary tumor volume contours (GTVt) and clinical parameters were available to predict endpoints, including 2-year local control (LC), regional control (RC), locoregional control (LRC), distant metastasis-free survival (DMFS), disease-specific survival (DSS), overall survival (OS), and disease-free survival (DFS). We proposed DL outcome prediction models with the multi-label learning (MLL) strategy that integrates the associations of different endpoints based on clinical factors and CT-scans. ResultsThe multi-label learning models outperformed the models that were developed based on a single endpoint for all endpoints especially with high AUCs >= 0.80 for 2-year RC, DMFS, DSS, OS, and DFS in the internal independent test set and for all endpoints except 2-year LRC in the external test set. Furthermore, with the models developed, patients could be stratified into high and low-risk groups that were significantly different for all endpoints in the internal test set and for all endpoints except DMFS in the external test set. ConclusionMLL models demonstrated better discriminative ability for all 2-year efficacy endpoints than single outcome models in the internal test and for all endpoints except LRC in the external set.
更多查看译文
关键词
computed tomography,deep learning,head and neck cancer,multi-label learning,oropharyngeal squamous cell carcinoma,outcome prediction
AI 理解论文
溯源树
样例
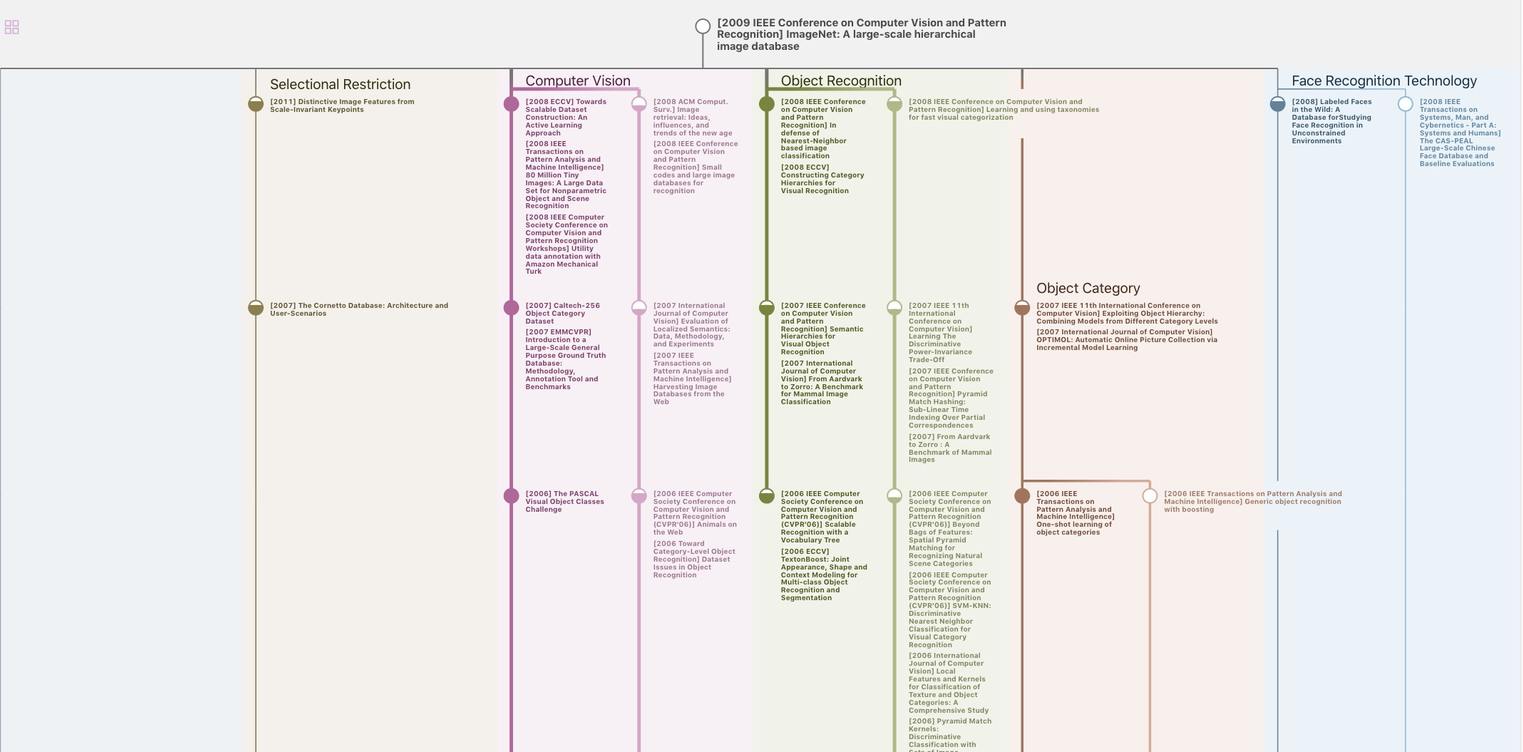
生成溯源树,研究论文发展脉络
Chat Paper
正在生成论文摘要