Resilience-Performance Tradeoff Analysis of a Deep Neural Network Accelerator
2023 26th International Symposium on Design and Diagnostics of Electronic Circuits and Systems (DDECS)(2023)
摘要
Nowadays, Deep Neural Networks (DNNs) are one of the most computationally-intensive algorithms because of the (i) huge amount of data to be transferred from/to the memory, and (ii) the huge amount of matrix multiplications to compute. These issues motivate the design of custom DNN hardware accelerators. These accelerators are widely used for low-latency safety-critical applications such as object detection in autonomous cars. Safety-critical applications have to be resilient with respect to hardware faults and Deep Learning (DL) accelerators are subjected to hardware faults that can cause functional failures, potentially leading to catastrophic consequences. Although DNNs possess a certain level of intrinsic resilience, it varies depending on the hardware on which they are run. The intent of the paper is to assess the resilience of a systolic-array-based DNN accelerator in the presence of hardware faults, in order to identify the architectural parameters that may mainly impact the DNN resilience.
更多查看译文
关键词
DNN Hardware accelerators,Fault Injection,Reliability
AI 理解论文
溯源树
样例
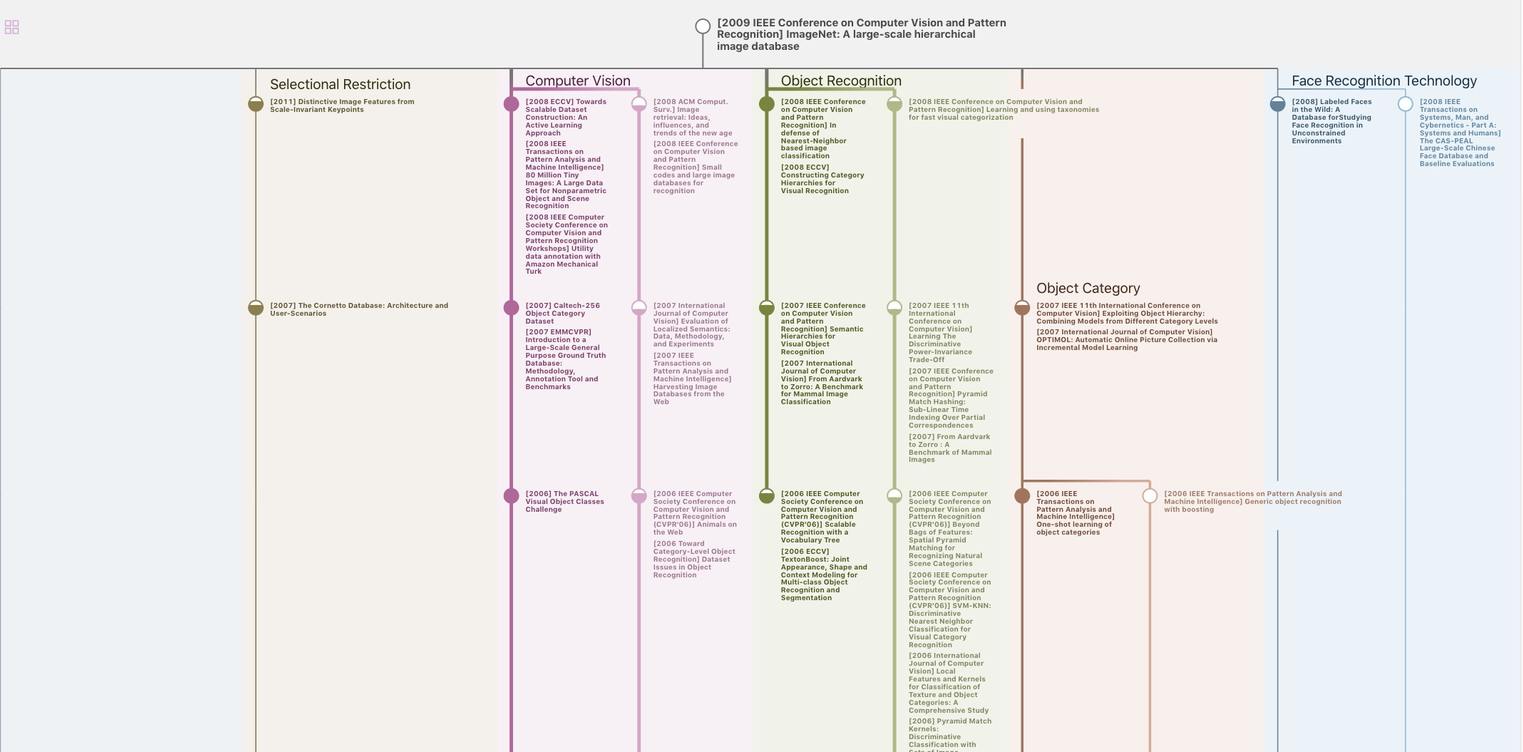
生成溯源树,研究论文发展脉络
Chat Paper
正在生成论文摘要