On the impact of activation and normalization in obtaining isometric embeddings at initialization
NeurIPS(2023)
摘要
In this paper, we explore the structure of the penultimate Gram matrix in deep neural networks, which contains the pairwise inner products of outputs corresponding to a batch of inputs. In several architectures it has been observed that this Gram matrix becomes degenerate with depth at initialization, which dramatically slows training. Normalization layers, such as batch or layer normalization, play a pivotal role in preventing the rank collapse issue. Despite promising advances, the existing theoretical results (i) do not extend to layer normalization, which is widely used in transformers, (ii) can not characterize the bias of normalization quantitatively at finite depth. To bridge this gap, we provide a proof that layer normalization, in conjunction with activation layers, biases the Gram matrix of a multilayer perceptron towards isometry at an exponential rate with depth at initialization. We quantify this rate using the Hermite expansion of the activation function, highlighting the importance of higher order ($\ge 2$) Hermite coefficients in the bias towards isometry.
更多查看译文
关键词
embeddings,normalization,initialization,activation
AI 理解论文
溯源树
样例
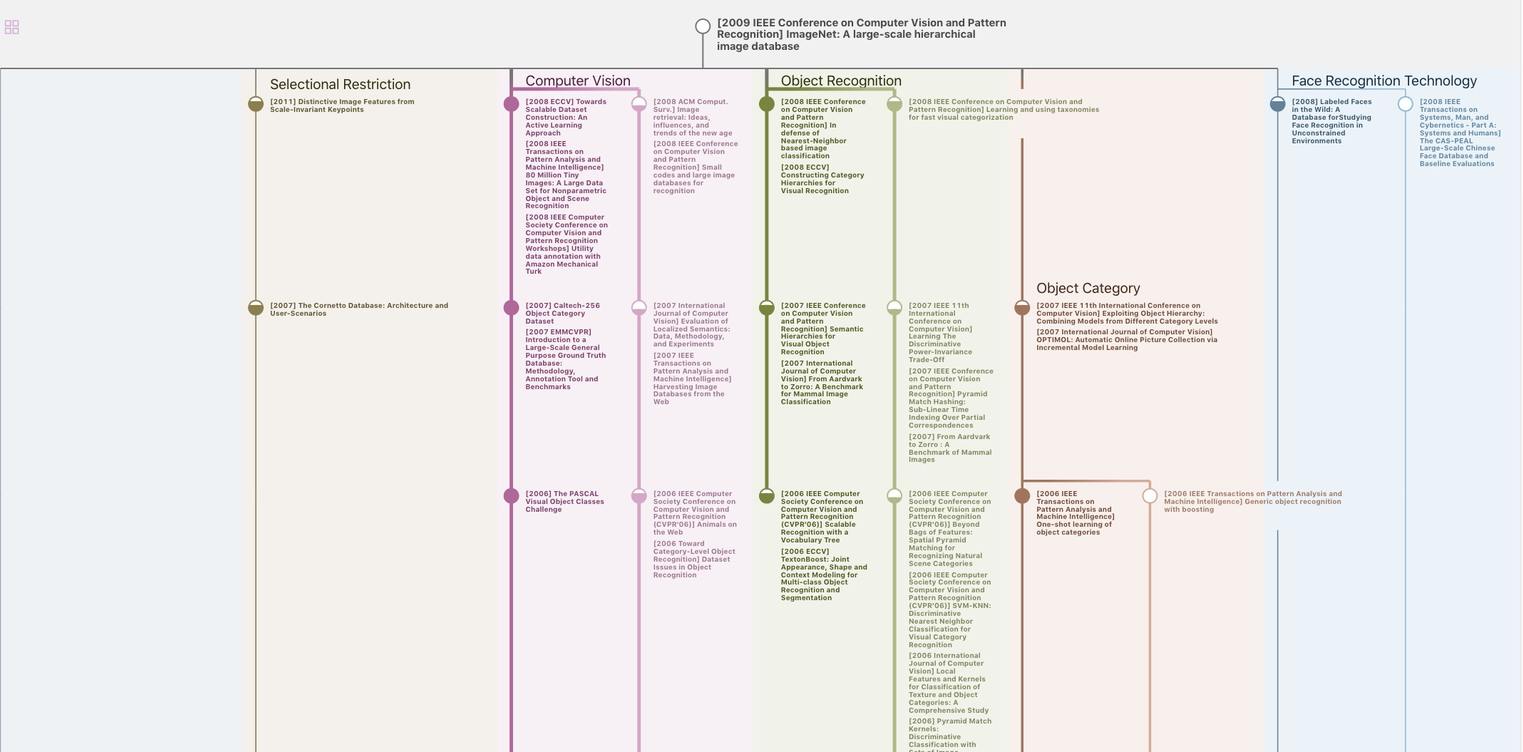
生成溯源树,研究论文发展脉络
Chat Paper
正在生成论文摘要