Counterfactual Co-occurring Learning for Bias Mitigation in Weakly-supervised Object Localization
arxiv(2023)
摘要
Contemporary weakly-supervised object localization (WSOL) methods have
primarily focused on addressing the challenge of localizing the most
discriminative region while largely overlooking the relatively less explored
issue of biased activation – incorrectly spotlighting co-occurring background
with the foreground feature. In this paper, we conduct a thorough causal
analysis to investigate the origins of biased activation. Based on our
analysis, we attribute this phenomenon to the presence of co-occurring
background confounders. Building upon this profound insight, we introduce a
pioneering paradigm known as Counterfactual Co-occurring Learning (CCL),
meticulously engendering counterfactual representations by adeptly
disentangling the foreground from the co-occurring background elements.
Furthermore, we propose an innovative network architecture known as
Counterfactual-CAM. This architecture seamlessly incorporates a perturbation
mechanism for counterfactual representations into the vanilla CAM-based model.
By training the WSOL model with these perturbed representations, we guide the
model to prioritize the consistent foreground content while concurrently
reducing the influence of distracting co-occurring backgrounds. To the best of
our knowledge, this study represents the initial exploration of this research
direction. Our extensive experiments conducted across multiple benchmarks
validate the effectiveness of the proposed Counterfactual-CAM in mitigating
biased activation.
更多查看译文
AI 理解论文
溯源树
样例
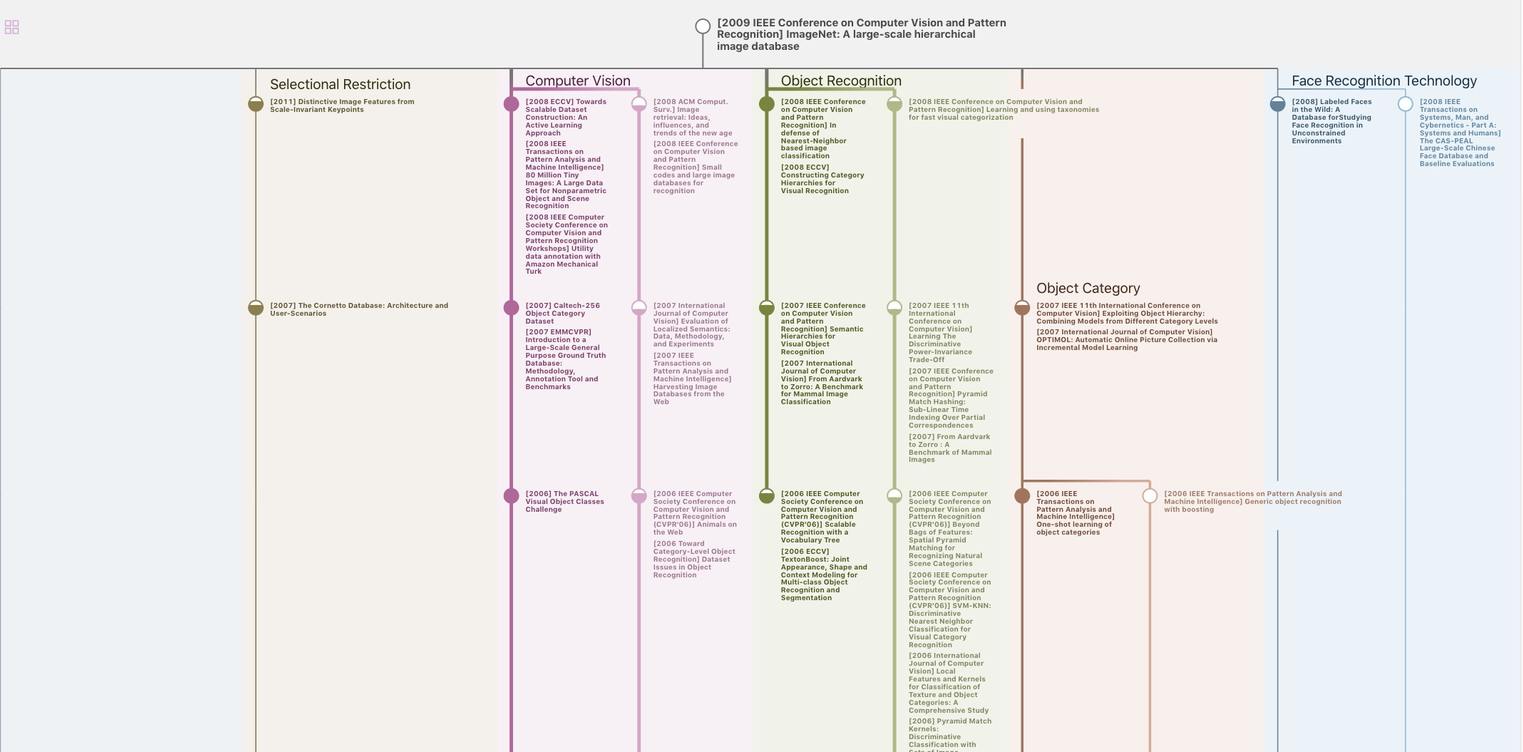
生成溯源树,研究论文发展脉络
Chat Paper
正在生成论文摘要