Evaluating the roughness of structure-property relationships using pretrained molecular representations
DIGITAL DISCOVERY(2023)
摘要
Quantitative structure-property relationships (QSPRs) aid in understanding molecular properties as a function of molecular structure. When the correlation between structure and property weakens, a dataset is described as "rough," but this characteristic is partly a function of the chosen representation. Among possible molecular representations are those from recently-developed "foundation models" for chemistry which learn molecular representation from unlabeled samples via self-supervision. However, the performance of these pretrained representations on property prediction benchmarks is mixed when compared to baseline approaches. We sought to understand these trends in terms of the roughness of the underlying QSPR surfaces. We introduce a reformulation of the roughness index (ROGI), ROGI-XD, to enable comparison of ROGI values across representations and evaluate various pretrained representations and those constructed by simple fingerprints and descriptors. We show that pretrained representations do not produce smoother QSPR surfaces, in agreement with previous empirical results of model accuracy. Our findings suggest that imposing stronger assumptions of smoothness with respect to molecular structure during model pretraining could aid in the downstream generation of smoother QSPR surfaces. Pretrained molecular representations are often thought to provide smooth, navigable latent spaces; analysis by ROGI-XD suggests they are no smoother than fixed descriptor/fingerprint representations.
更多查看译文
关键词
roughness,structure–property
AI 理解论文
溯源树
样例
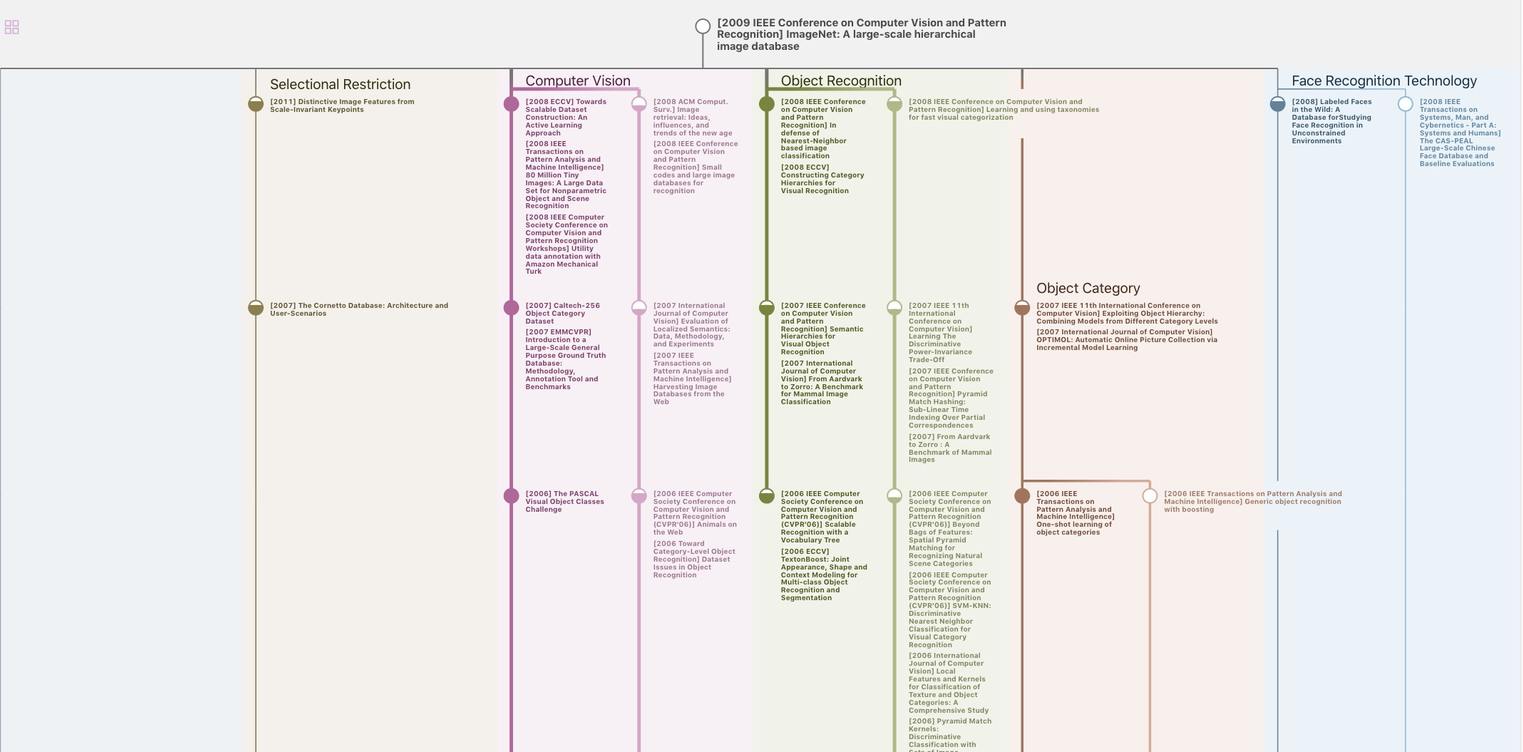
生成溯源树,研究论文发展脉络
Chat Paper
正在生成论文摘要