Dependency-aware task offloading based on deep reinforcement learning in mobile edge computing networks
WIRELESS NETWORKS(2023)
摘要
With the rapid development of innovative applications, lots of computation-intensive and delay-sensitive tasks are emerging. Task offloading, which is regarded as a key technology in the emerging mobile edge computing paradigm, aims at offloading the tasks from mobile devices (MDs) to edge servers or the remote cloud to reduce system delay and energy consumption of MDs. However, most existing task offloading studies either didn’t consider the dependencies among tasks or simply designed heuristic schemes to solve dependent task offloading problems. Different from these studies, we propose a deep reinforcement learning (DRL) based task offloading scheme to jointly offload tasks with dependencies. Specifically, we model the dependencies among tasks by directed acyclic graphs and formulate the task offloading problem as minimizing the average cost of energy and time (CET) of users. To solve this NP-hard problem, we propose a deep Q-network learning-based framework that creatively utilizes deep neural networks to extract system features. Simulation results show that our proposed scheme outperforms the existing DRL scheme and heuristic scheme in reducing the average CET of all users and can obtain near-optimal solutions.
更多查看译文
关键词
Mobile edge computing,Deep reinforcement learning,Directed acyclic graph,Deep neural network
AI 理解论文
溯源树
样例
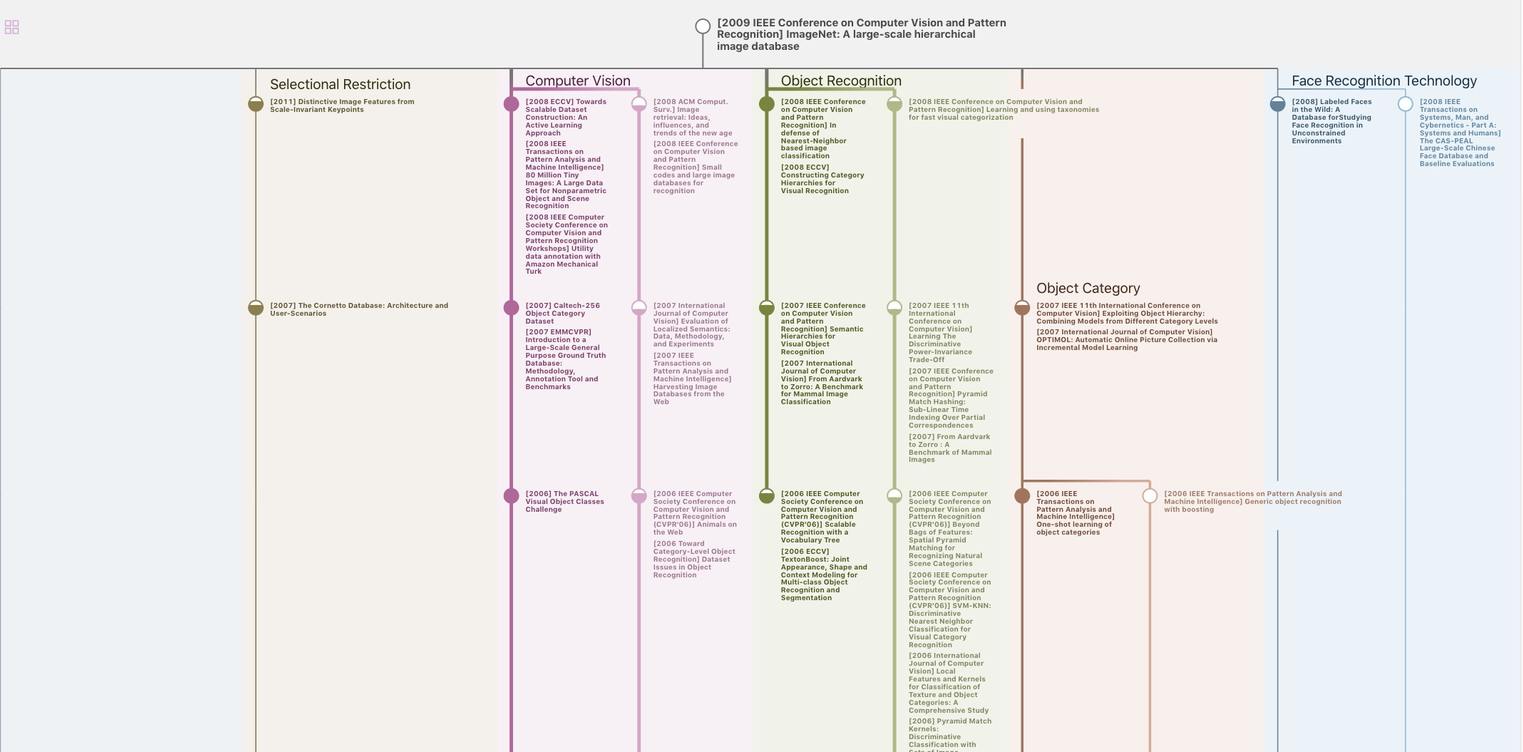
生成溯源树,研究论文发展脉络
Chat Paper
正在生成论文摘要