AutoNeRF: Training Implicit Scene Representations with Autonomous Agents
CoRR(2023)
摘要
Implicit representations such as Neural Radiance Fields (NeRF) have been shown to be very effective at novel view synthesis. However, these models typically require manual and careful human data collection for training. In this paper, we present AutoNeRF, a method to collect data required to train NeRFs using autonomous embodied agents. Our method allows an agent to explore an unseen environment efficiently and use the experience to build an implicit map representation autonomously. We compare the impact of different exploration strategies including handcrafted frontier-based exploration and modular approaches composed of trained high-level planners and classical low-level path followers. We train these models with different reward functions tailored to this problem and evaluate the quality of the learned representations on four different downstream tasks: classical viewpoint rendering, map reconstruction, planning, and pose refinement. Empirical results show that NeRFs can be trained on actively collected data using just a single episode of experience in an unseen environment, and can be used for several downstream robotic tasks, and that modular trained exploration models significantly outperform the classical baselines.
更多查看译文
关键词
implicit scene representations,agents,training
AI 理解论文
溯源树
样例
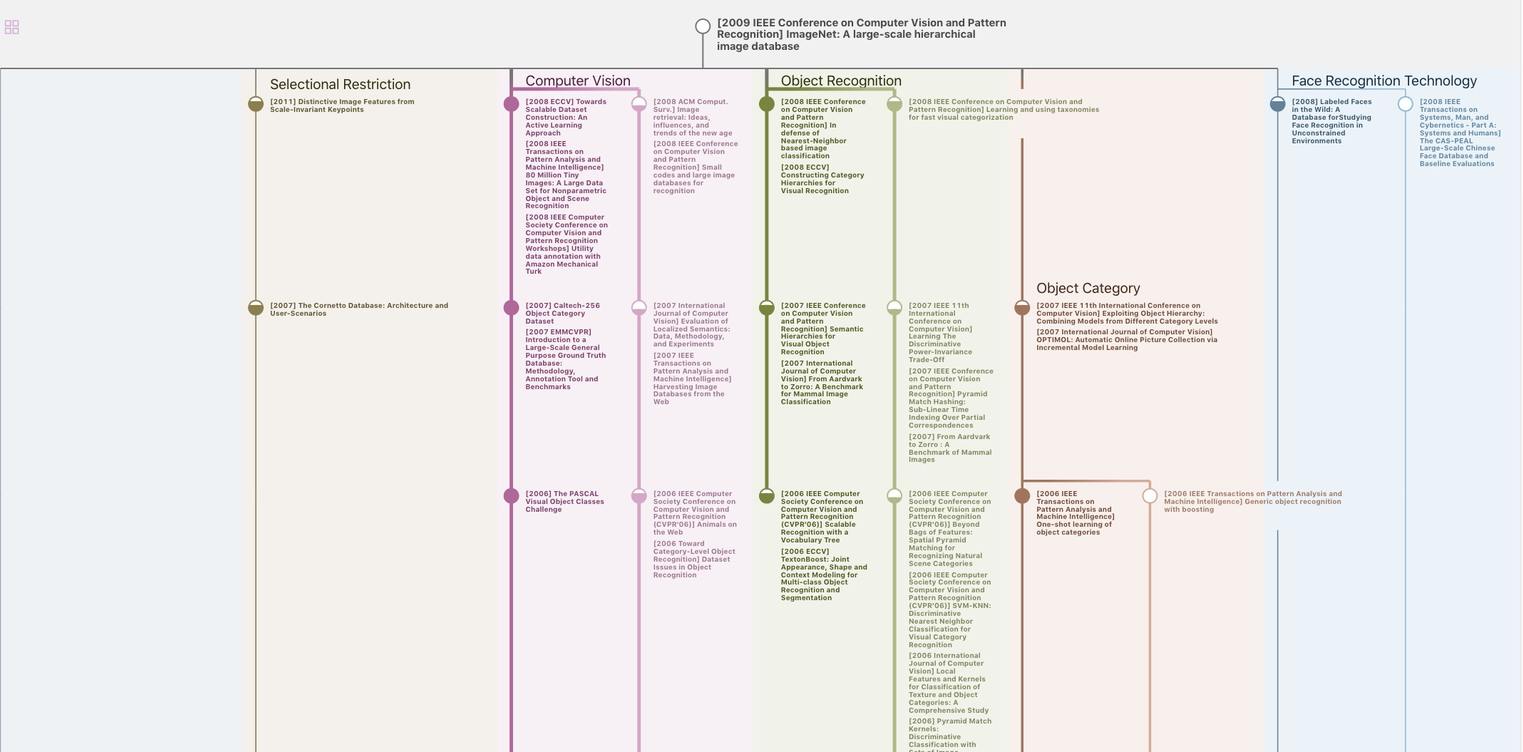
生成溯源树,研究论文发展脉络
Chat Paper
正在生成论文摘要