Offline Reinforcement Learning for Price-Based Demand Response Program Design
2023 57th Annual Conference on Information Sciences and Systems (CISS)(2023)
摘要
In this paper, price-based demand response (DR) program design by offline Reinforcement Learning (RL) with data collected from smart meters is studied. Unlike online RL approaches, offline RL does not need to interact with consumers in the real world and thus has great cost-effectiveness and safety advantages. A sequential decision-making process with a Markov Decision Process (MDP) framework is formulated. A novel data augmentation method based on bootstrapping is developed. Deep Q-network (DQN)-based offline RL and policy evaluation algorithms are developed to design high-performance DR pricing policies. The developed offline learning methods are evaluated on both a real-world data set and simulation environments. It is demonstrated that the performance of the developed offline RL methods achieve excellent performance that is very close to the ideal performance bound provided by the state-of-the-art online RL algorithms.
更多查看译文
AI 理解论文
溯源树
样例
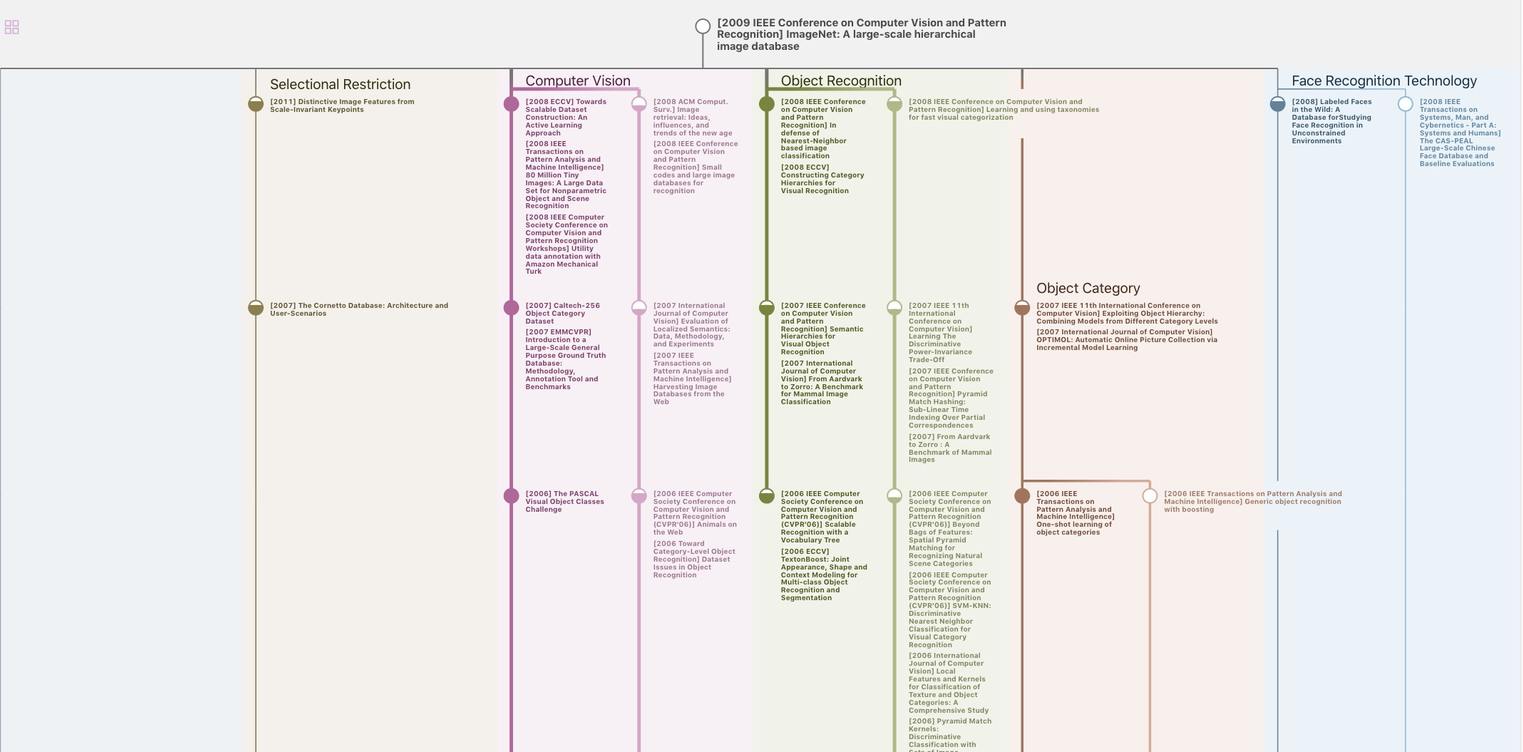
生成溯源树,研究论文发展脉络
Chat Paper
正在生成论文摘要