Characterizing the interaction conformation between T-cell receptors and epitopes with deep learning
Nature Machine Intelligence(2023)
摘要
Computational modelling of the interactions between T-cell receptors (TCRs) and epitopes is of great importance for immunotherapy and antigen discovery. However, current TCR–epitope interaction prediction tools are still in a relatively primitive stage and have limited capacity in deciphering the underlying binding mechanisms, for example, characterizing the pairwise residue interactions between TCRs and epitopes. Here we designed a new deep-learning-based framework for modelling TCR–epitope interactions, called TCR–Epitope Interaction Modelling at Residue Level (TEIM-Res), which took the sequences of TCRs and epitopes as input and predicted both pairwise residue distances and contact sites involved in the interactions. To tackle the current bottleneck of data deficiency, we applied a few-shot learning strategy by incorporating sequence-level binding information into residue-level interaction prediction. The validation experiments and analyses indicated its good prediction performance and the effectiveness of its design. We demonstrated three potential applications: revealing the subtle conformation changes of mutant TCR–epitope pairs, uncovering the key contacts based on epitope-specific TCR pools, and mining the intrinsic binding rules and patterns. In summary, our model can serve as a useful tool for comprehensively characterizing TCR–epitope interactions and understanding the molecular basis of binding mechanisms.
更多查看译文
关键词
Cellular immunity,Computational biology and bioinformatics,Machine learning,Protein function predictions,Engineering,general
AI 理解论文
溯源树
样例
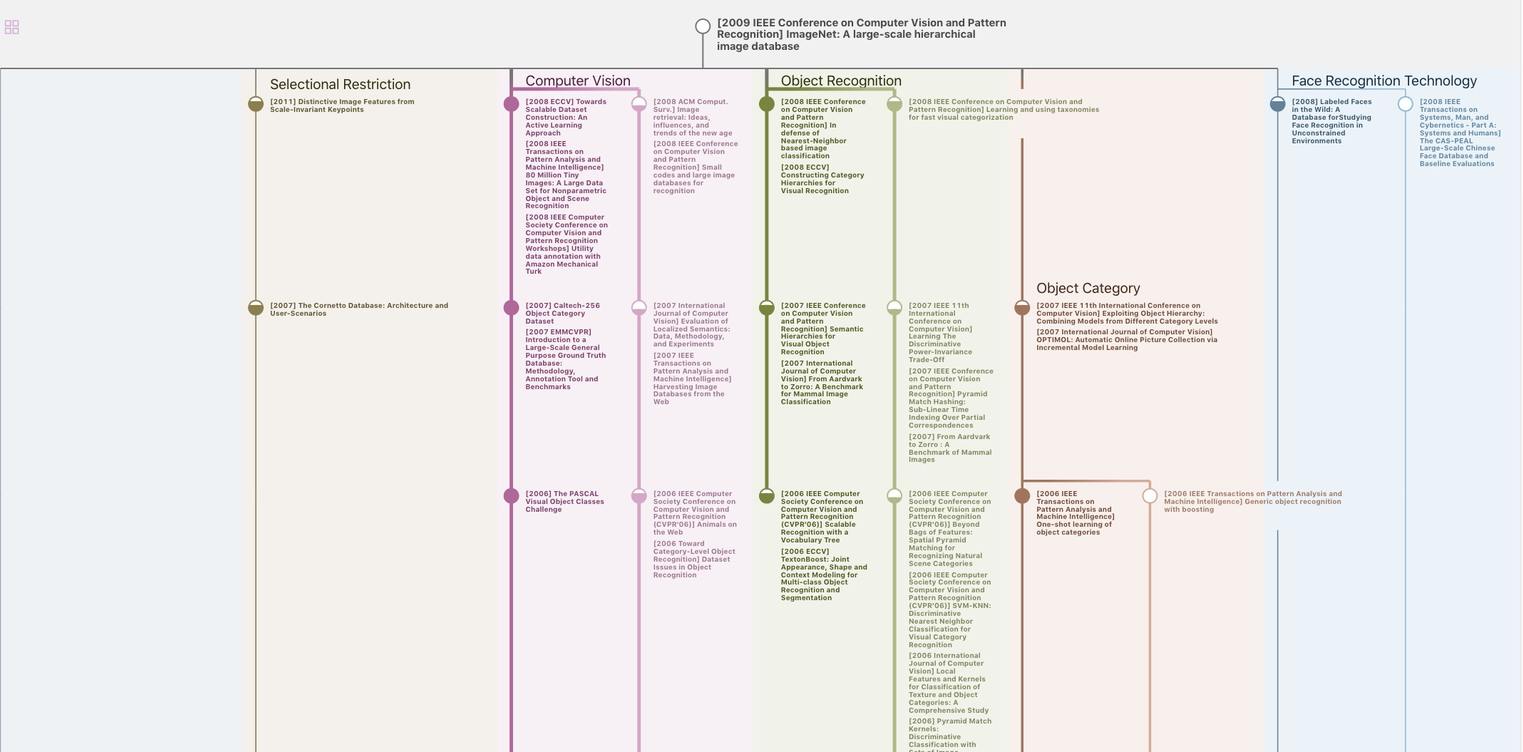
生成溯源树,研究论文发展脉络
Chat Paper
正在生成论文摘要