Highway Reinforcement Learning
ICLR 2023(2023)
摘要
Traditional Dynamic Programming (DP) approaches suffer from slow backward credit-assignment (CA): only a one-step search is performed at each update. A popular solution for multi-step CA is to use multi-step Bellman operators. Unfortunately, in the control settings, existing methods typically suffer from the large variance of multi-step off-policy corrections or are biased, preventing convergence. To overcome these problems, we introduce a novel multi-step Bellman optimality equation with adaptive lookahead steps. We first derive a new multi-step Value Iteration (VI) method that converges to the optimal Value Function (VF) with an exponential contraction rate but linear computational complexity. Given some trial, our so-called Highway RL performs rapid CA, by picking a policy and a possible lookahead (up to the trial end) that maximize the near-term reward during lookahead plus a DP-based estimate of the cumulative reward for the remaining part of the trial. Highway RL does not require off-policy corrections. Under mild assumptions, it achieves better convergence rates than the traditional one-step Bellman Optimality Operator. We then derive Highway Q-Learning, a convergent multi-step off-policy variant of Q-learning. We show that our Highway algorithms significantly outperform DP approaches on toy tasks. Finally, we propose a deep function approximation variant called Highway DQN. We evaluate it on visual MinAtar Games, outperforming similar multi-step methods.
更多查看译文
关键词
reinforcement learning,off-policy learning,credit assignment,Bellman Equation
AI 理解论文
溯源树
样例
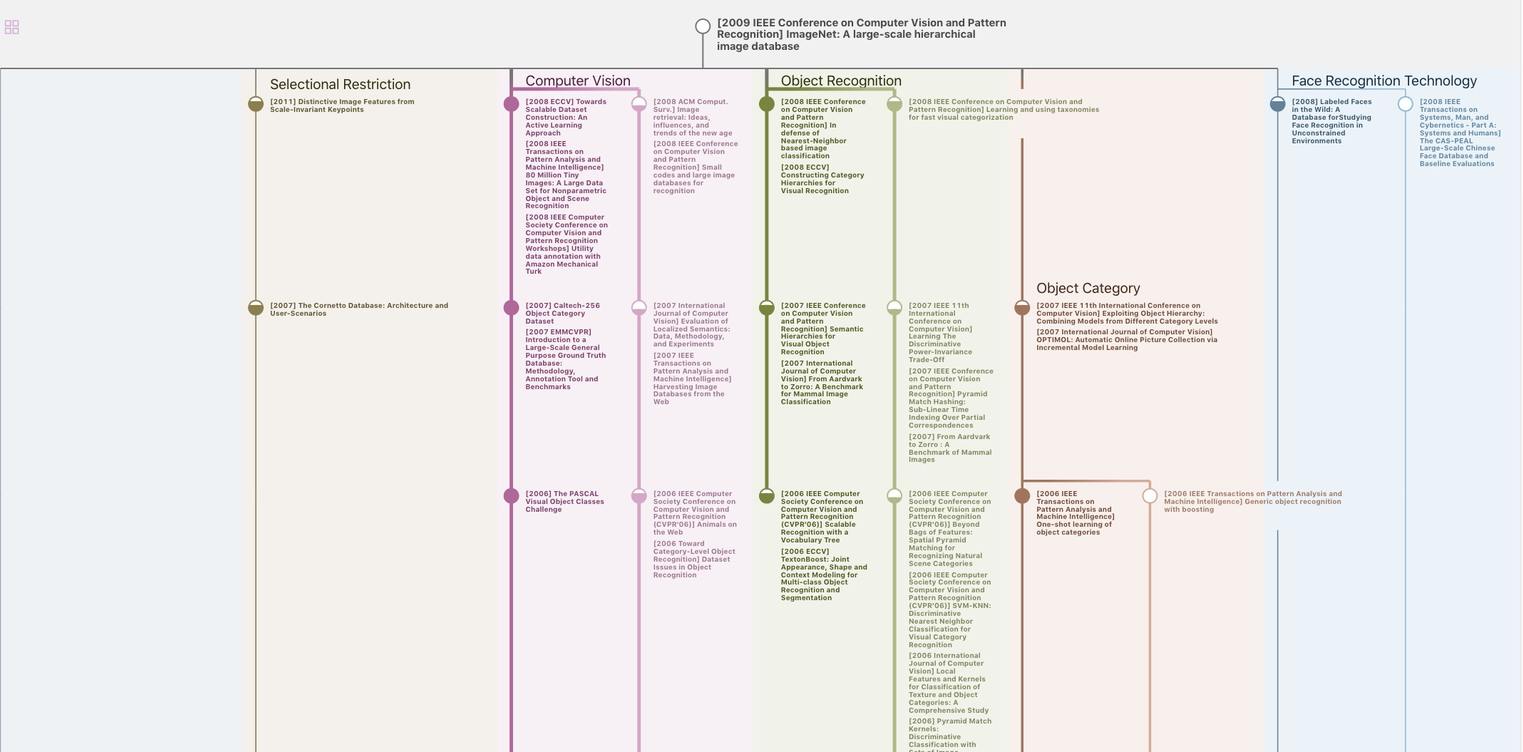
生成溯源树,研究论文发展脉络
Chat Paper
正在生成论文摘要