Gradient Deconfliction via Orthogonal Projections onto Subspaces For Multi-task Learning
ICLR 2023(2023)
摘要
Although multi-task learning (MTL) has been a preferred approach and successfully applied in many real-world scenarios, MTL models are not guaranteed to outperform single-task models on all tasks mainly due to the negative effects of conflicting gradients among the tasks. In this paper, we fully examine the influence of conflicting gradients and further emphasize the importance and advantages of achieving non-conflicting gradients which allows simple but effective trade-off strategies among the tasks with stable performance. Base on our findings, we propose the Gradient Deconfliction via Orthogonal Projections onto Subspaces (GradOPS) spanned by other task-specific gradients. Our method not only solves all conflicts among the tasks, but can also effectively search for diverse solutions towards different trade-off preferences among the tasks. Theoretical analysis on convergence is provided, and performance of our algorithm is fully testified on multiple benchmarks in various domains. Results demonstrate that our method can effectively find multiple state-of-the-art solutions with different trade-off strategies among the tasks on multiple datasets.
更多查看译文
关键词
multi-task learning,deep learning
AI 理解论文
溯源树
样例
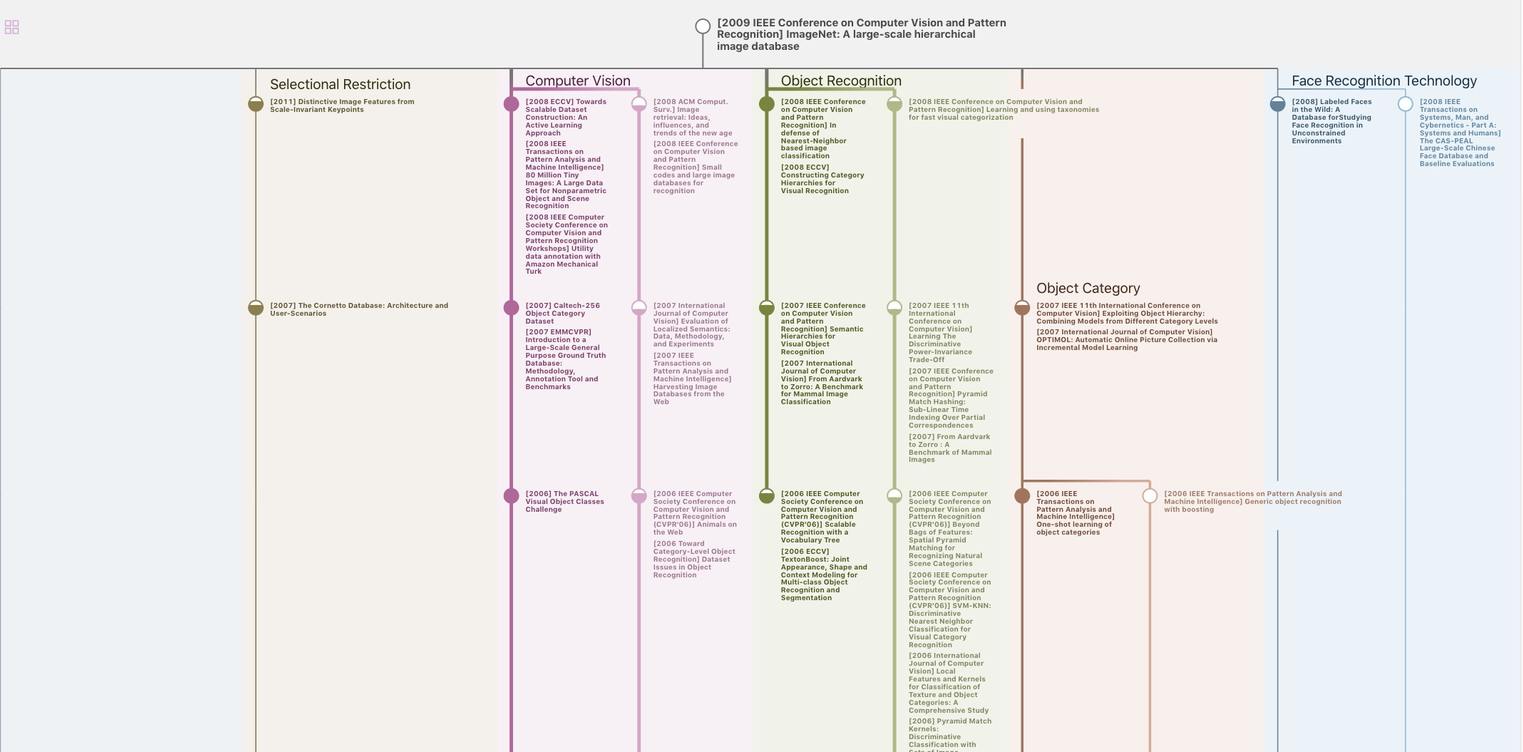
生成溯源树,研究论文发展脉络
Chat Paper
正在生成论文摘要