Towards Unsupervised Time Series Representation Learning: A Decomposition Perspective
ICLR 2023(2023)
摘要
Existing contrastive methods of universal time series representation learning mainly rely on distilling invariant patterns at varying scales and building contrastive loss with the help of negative sampling. However, the invariance assumptions may not hold in real-world time-series data, and the infamous negative sampling could bring in new biases for representation learning. In this work, we propose a novel contrastive learning approach toward time series representation learning on top of trend-seasonality decomposition, namely TS-DC. TS-DC differentiates itself from prior methods in three folds: 1) a time series decomposition approach is devised to distill different aspects/components of a complex time series; 2) a novel component-wise contrastive loss is proposed in which negative sampling is not necessary; 3) the informative signals of time series can be captured comprehensively by means of adaptive contrasting. Extensive experiments on different public benchmark datasets validate the superior performance of our proposed representation learning method.
更多查看译文
关键词
Time Series,Representation Learning,Contrastive Learning
AI 理解论文
溯源树
样例
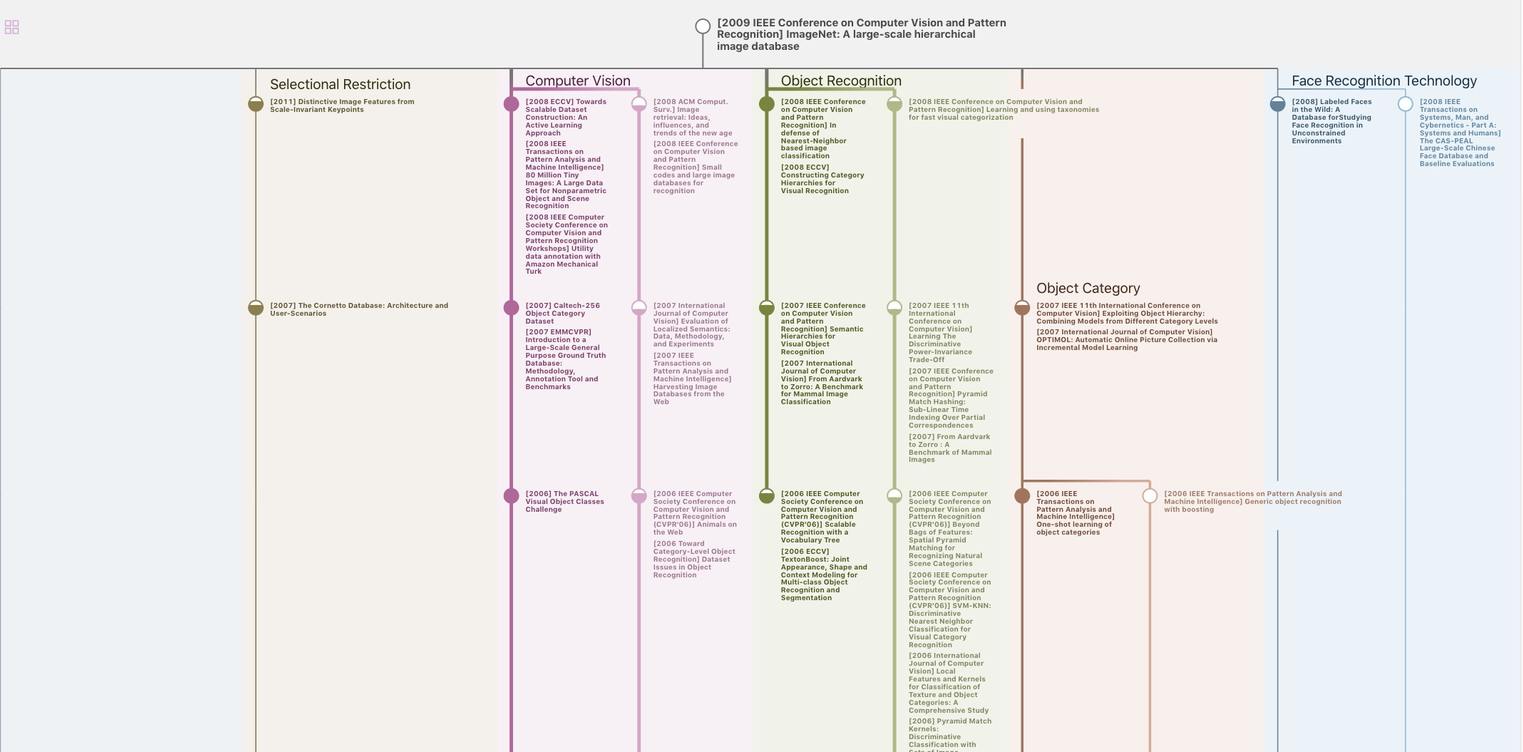
生成溯源树,研究论文发展脉络
Chat Paper
正在生成论文摘要