A deep Koopman operator-based modelling approach for long-term prediction of dynamics with pixel-level measurements
CAAI TRANSACTIONS ON INTELLIGENCE TECHNOLOGY(2024)
摘要
Although previous studies have made some clear leap in learning latent dynamics from high-dimensional representations, the performances in terms of accuracy and inference time of long-term model prediction still need to be improved. In this study, a deep convolutional network based on the Koopman operator (CKNet) is proposed to model non-linear systems with pixel-level measurements for long-term prediction. CKNet adopts an autoencoder network architecture, consisting of an encoder to generate latent states and a linear dynamical model (i.e., the Koopman operator) which evolves in the latent state space spanned by the encoder. The decoder is used to recover images from latent states. According to a multi-step ahead prediction loss function, the system matrices for approximating the Koopman operator are trained synchronously with the autoencoder in a mini-batch manner. In this manner, gradients can be synchronously transmitted to both the system matrices and the autoencoder to help the encoder self-adaptively tune the latent state space in the training process, and the resulting model is time-invariant in the latent space. Therefore, the proposed CKNet has the advantages of less inference time and high accuracy for long-term prediction. Experiments are performed on OpenAI Gym and Mujoco environments, including two and four non-linear forced dynamical systems with continuous action spaces. The experimental results show that CKNet has strong long-term prediction capabilities with sufficient precision.
更多查看译文
关键词
deep neural networks,image motion analysis,image sequences,sequential estimation
AI 理解论文
溯源树
样例
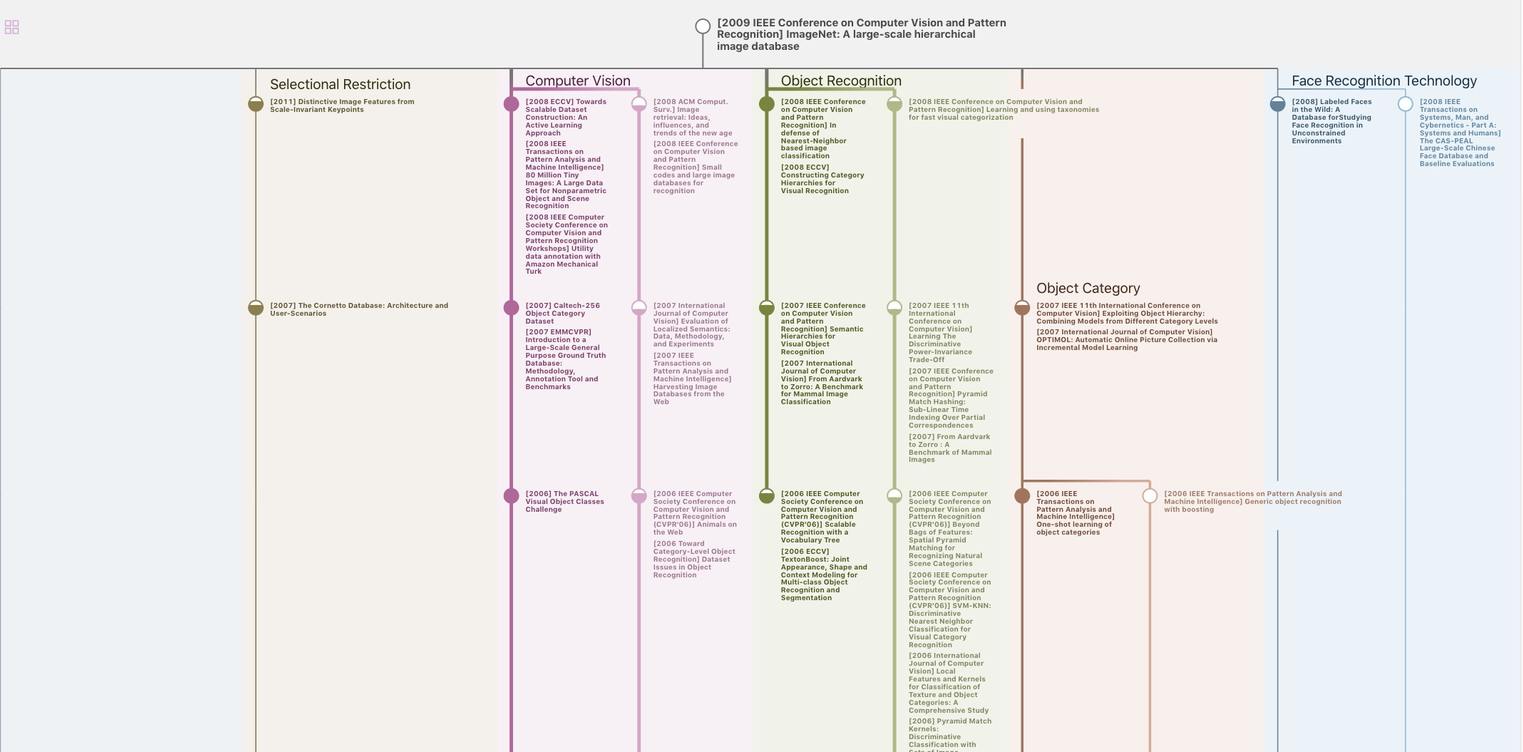
生成溯源树,研究论文发展脉络
Chat Paper
正在生成论文摘要