Universal Smoothed Score Functions for Generative Modeling
arxiv(2023)
摘要
We consider the problem of generative modeling based on smoothing an unknown density of interest in $\mathbb{R}^d$ using factorial kernels with $M$ independent Gaussian channels with equal noise levels introduced by Saremi and Srivastava (2022). First, we fully characterize the time complexity of learning the resulting smoothed density in $\mathbb{R}^{Md}$, called M-density, by deriving a universal form for its parametrization in which the score function is by construction permutation equivariant. Next, we study the time complexity of sampling an M-density by analyzing its condition number for Gaussian distributions. This spectral analysis gives a geometric insight on the "shape" of M-densities as one increases $M$. Finally, we present results on the sample quality in this class of generative models on the CIFAR-10 dataset where we report Fr\'echet inception distances (14.15), notably obtained with a single noise level on long-run fast-mixing MCMC chains.
更多查看译文
关键词
universal smoothed score functions
AI 理解论文
溯源树
样例
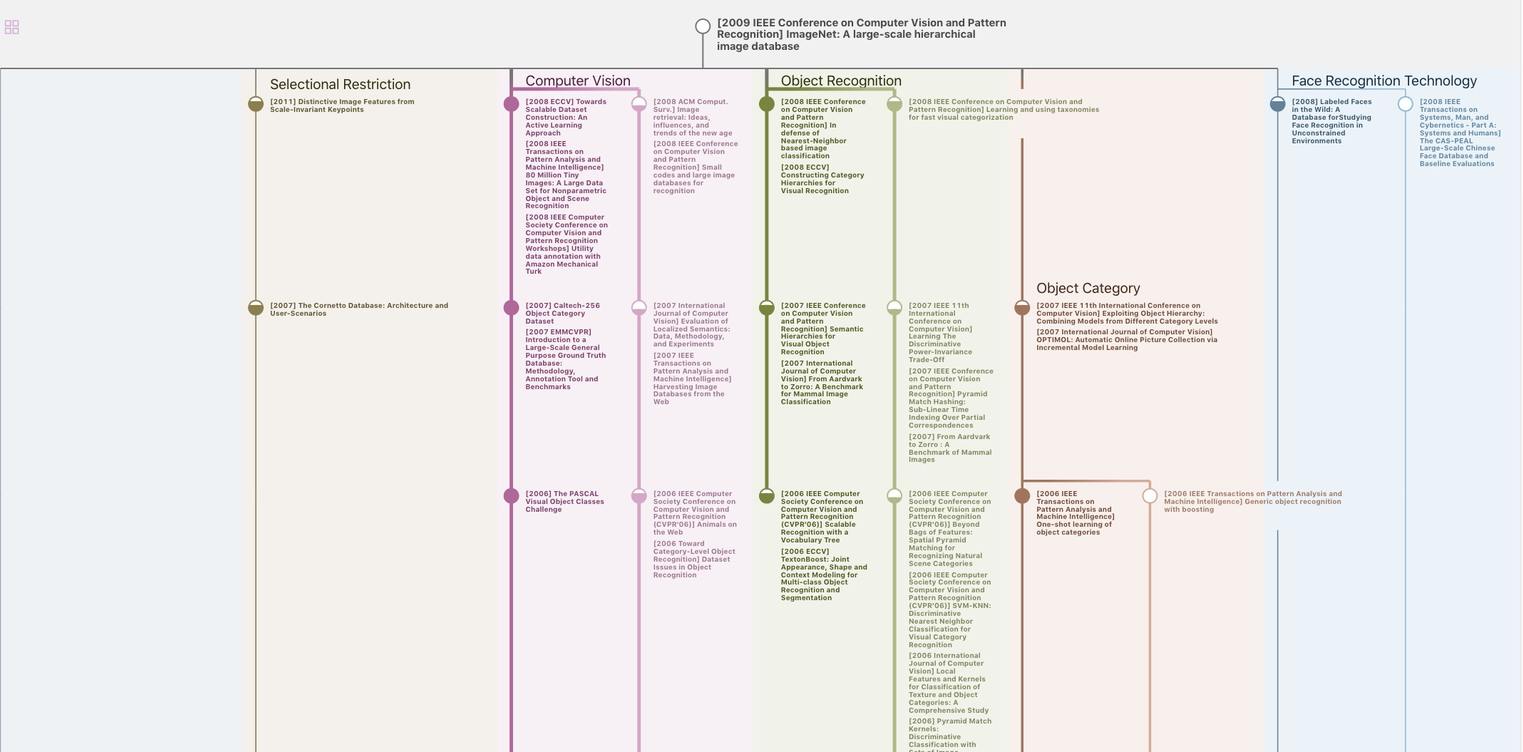
生成溯源树,研究论文发展脉络
Chat Paper
正在生成论文摘要