Toward Interpretability in Fault Diagnosis for Autonomous Vehicles: Interpretation of Sensor Data Anomalies
IEEE Sensors Journal(2023)
摘要
To guarantee the safety and reliability of autonomous driving applications, it is indispensable to construct a proper fault diagnosis framework tailored to autonomous vehicles. Fault diagnosis aims to provide essential information about the system operational status and its interpretation facilitates decision-making and mitigates the potential operation risks. In the present work, interpretability issue in fault diagnosis for autonomous vehicles is discussed from the sensor data analytics perspective. Environmental impact is first evaluated using the noise energy as a measure to interpret the impact on sensor data caused by the environment. A signal quality indicator is proposed and Savitzky–Golay filters are applied for online denoising, acting as a countermeasure to mitigate the impact and to enhance the data quality. Then, the adversarial learned denoising shrinkage autoencoder (ALDSAE), an adversarial learning neural network, is constructed for sensor data anomaly detection. It considers an adversarial training technique to improve the performance of the anomaly detector. A residual explainer specific to the ALDSAE model is employed to calculate the contribution of each input feature to the anomaly score in order to interpret the anomaly detection results. Several experiments with the collected data from an autonomous vehicle in a real test field are implemented to validate the effectiveness of the proposed approaches. Results show that the area under the ROC curve (AUC_ROC) of the proposed ALDSAE is over 20% higher in average than several traditional anomaly detectors, and the mean explanation accuracy of the residual explainer achieves similar performance with the widely employed kernel Shapley additive explanation (SHAP) with more than 99% reduction in mean response time.
更多查看译文
关键词
Autonomous vehicles,environmental impact quantification,fault diagnosis,sensor data anomaly interpretation
AI 理解论文
溯源树
样例
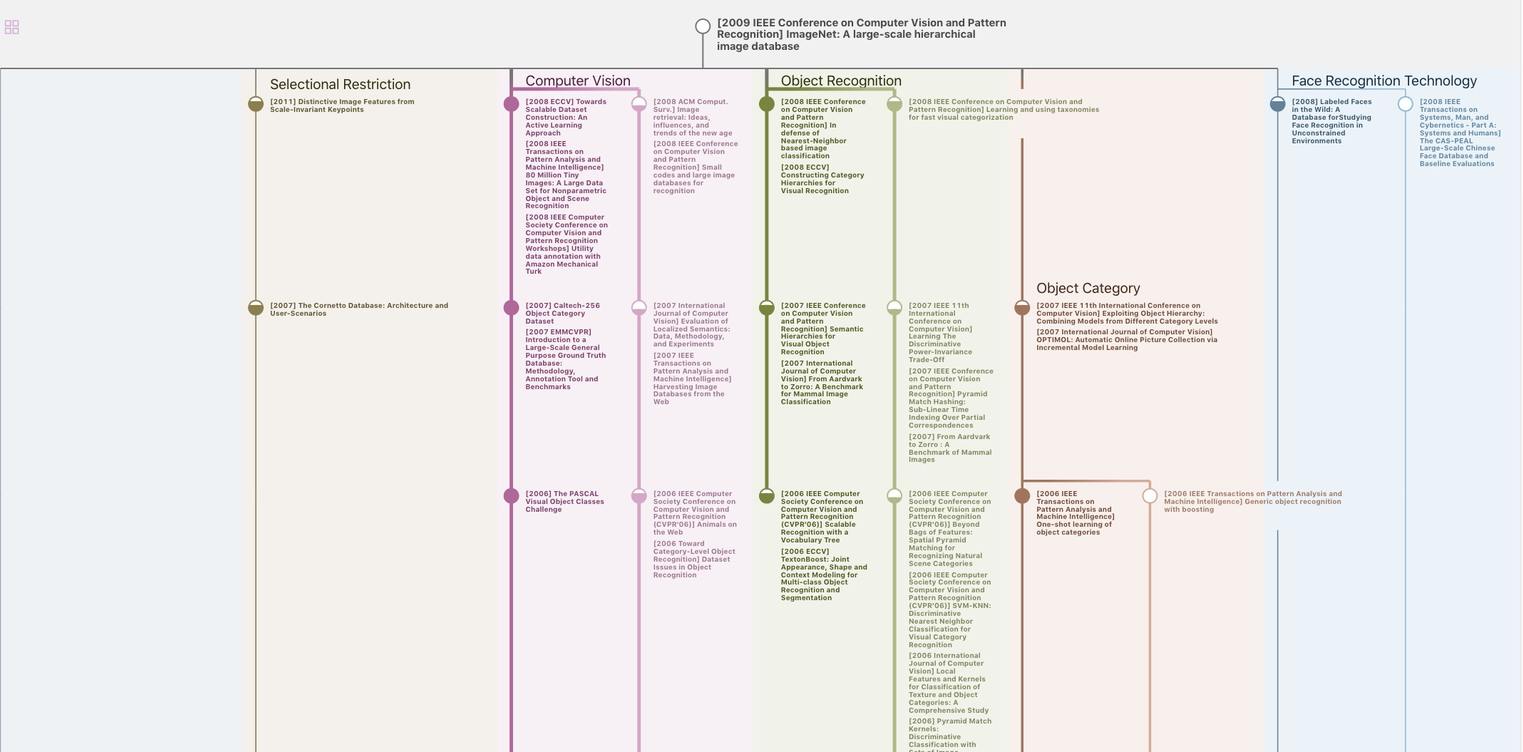
生成溯源树,研究论文发展脉络
Chat Paper
正在生成论文摘要