Fast Trajectory End-Point Prediction with Event Cameras for Reactive Robot Control
arxiv(2023)
摘要
Prediction skills can be crucial for the success of tasks where robots have limited time to act or joints actuation power. In such a scenario, a vision system with a fixed, possibly too low, sampling rate could lead to the loss of informative points, slowing down prediction convergence and reducing the accuracy. In this paper, we propose to exploit the low latency, motion-driven sampling, and data compression properties of event cameras to overcome these issues. As a use-case, we use a Panda robotic arm to intercept a ball bouncing on a table. To predict the interception point, we adopt a Stateful LSTM network, a specific LSTM variant without fixed input length, which perfectly suits the event-driven paradigm and the problem at hand, where the length of the trajectory is not defined. We train the network in simulation to speed up the dataset acquisition and then fine-tune the models on real trajectories. Experimental results demonstrate how using a dense spatial sampling (i.e. event cameras) significantly increases the number of intercepted trajectories as compared to a fixed temporal sampling (i.e. frame-based cameras).
更多查看译文
关键词
event cameras,robot,trajectory,end-point
AI 理解论文
溯源树
样例
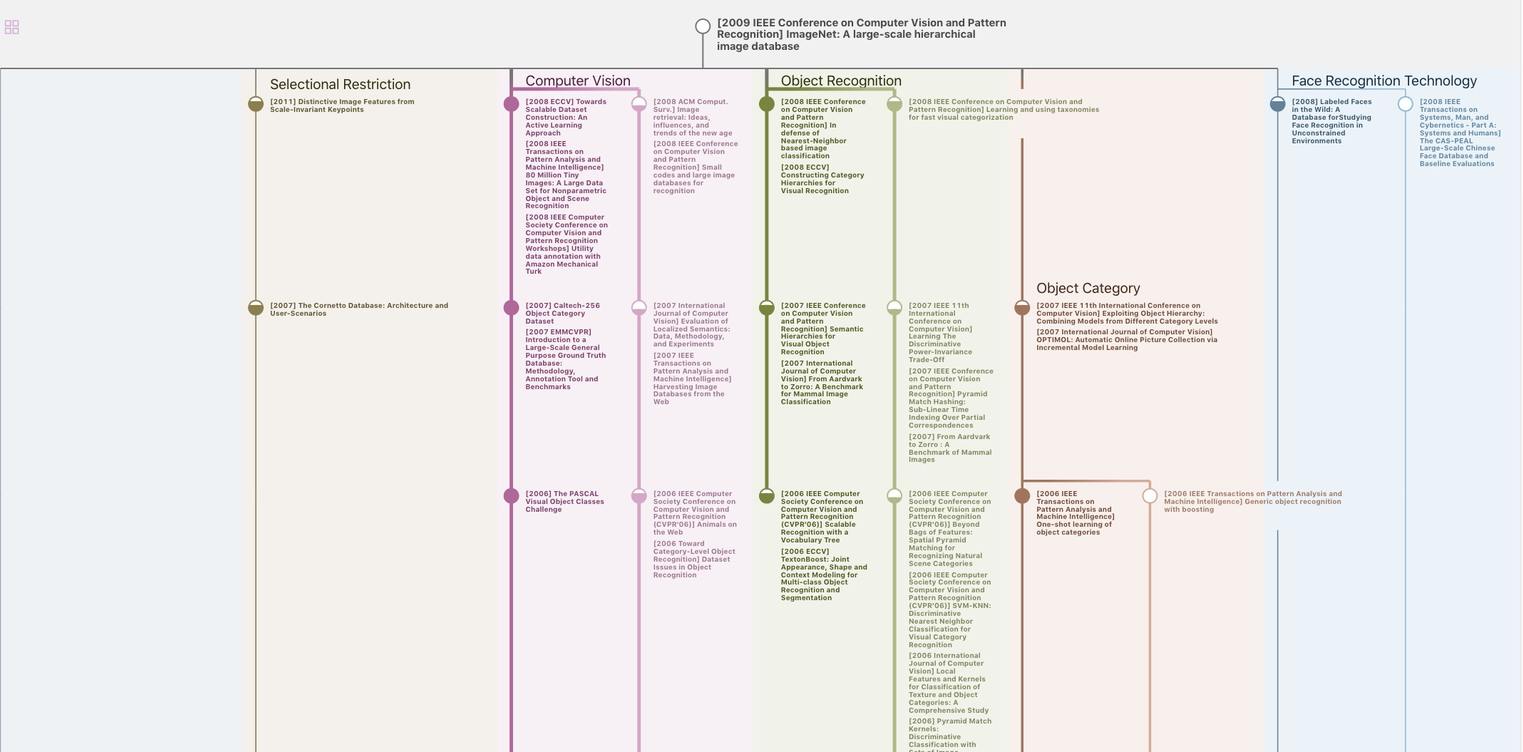
生成溯源树,研究论文发展脉络
Chat Paper
正在生成论文摘要