On Model Transmission Strategies in Federated Learning With Lossy Communications
IEEE Transactions on Parallel and Distributed Systems(2023)
摘要
Recently, federated learning (FL) has received tremendous attention in both academia and industry, in which decentralized clients collaboratively complete model training by exchanging model updates with a parameter server through the Internet. Its distributed nature well utilizes the localized data and preserves clients’ privacy, but also incurs heavy communication overhead. Existing studies on model update have mostly focused on the bandwidth constraint of the communication channels. Today's Internet however is highly unreliable. Simply using Transmission Control Protocol (TCP) would lead to low network utilization under frequent losses. In this paper, we closely examine the optimal transmission strategies in FL over the realistic lossy Internet. We systematically integrate model compression, forward error correction (FEC) and retransmission towards Federated Learning with Lossy Communications (FedLC). We derive the convergence rate of FedLC under non-convex loss with the optimal transmission. We then decompose this non-convex problem and present effective practical solutions. Public datasets are exploited for performance evaluation by varying the packet loss rate from 10% to 50%. In a fixed training time budget, FedLC can improve model accuracy by 3.91% on average or reduce the communication traffic by 34.27%-47.57% in comparison with state-of-the-art baselines.
更多查看译文
关键词
Compression,federated learning,forward error correction,lossy communication,retransmission
AI 理解论文
溯源树
样例
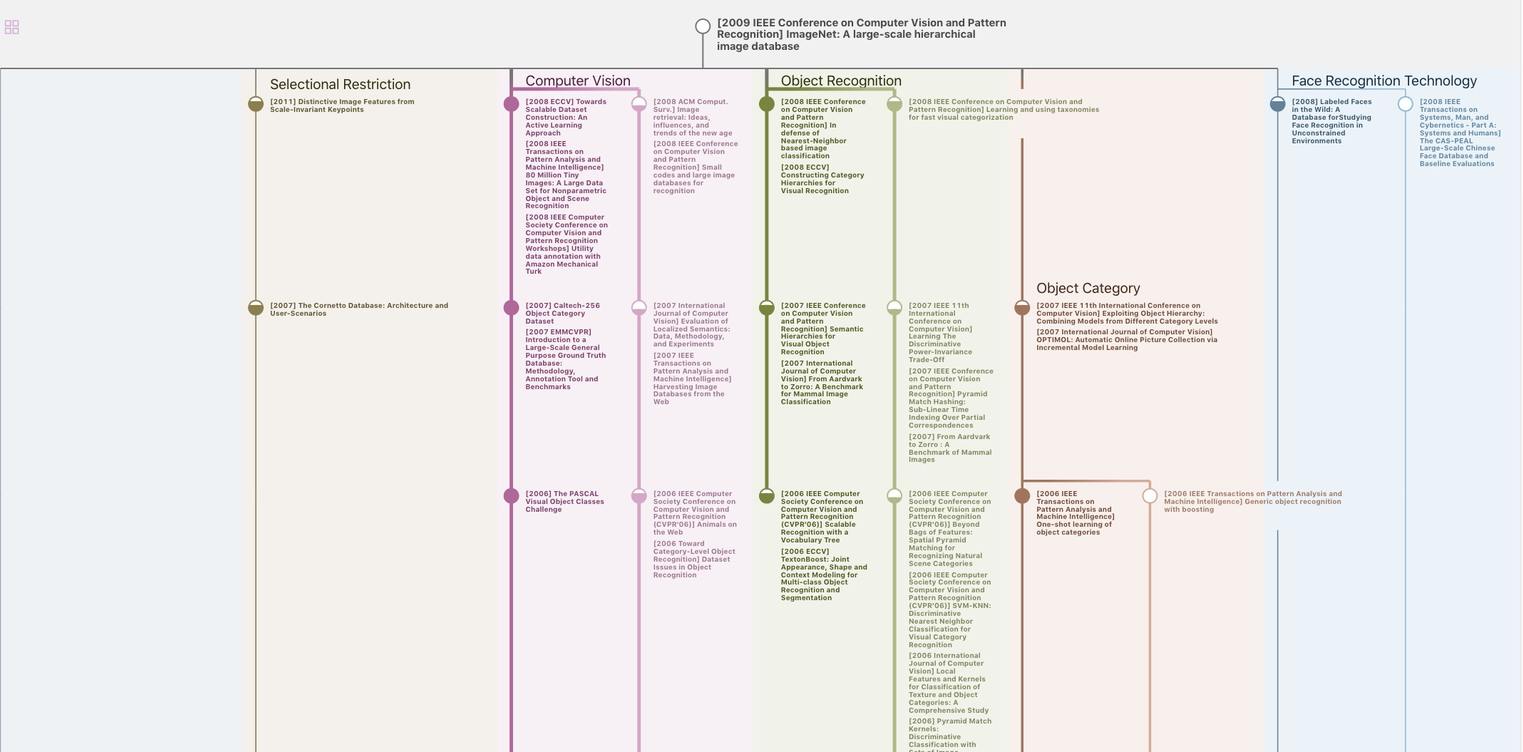
生成溯源树,研究论文发展脉络
Chat Paper
正在生成论文摘要