StyleMorph: Disentangling Shape, Pose and Appearance through 3D Morphable Image and Geometry Generation
ICLR 2023(2023)
摘要
We introduce StyleMorph, a 3D generative model that relies on the 3D morphable model paradigm to disentangle shape, pose, object and scene texture for high quality image synthesis. We represent 3D shape variability through 3D deformation fields with respect to a canonical object template. Both the deformations and the template are expressed as implicit networks and learned in an unsupervised manner only from 2D image supervision. We connect 3D morphable modelling with deferred neural rendering by performing an implicit surface rendering of “Template Object Coordinates” (TOCS), thereby constructing a purely geometric, deformation-equivariant 2D signal that reflects the compounded geometric effects of non-rigid shape, pose, and perspective projection. We use TOCS maps in tandem with object and background appearance codes to condition a StyleGAN-based deferred neural rendering (DNR) network for high-resolution image synthesis. We show competitive photorrealistic image synthesis results on 4 datasets (FFHQ faces, AFHQ Cats, Dogs, Wild), while achieving the joint disentanglement of shape, pose, object and scene texture.
更多查看译文
关键词
3D-aware GAN,Template-based,Morphable,Disentanglement,Photorealistic,Neural Radiance Field,StyleGAN
AI 理解论文
溯源树
样例
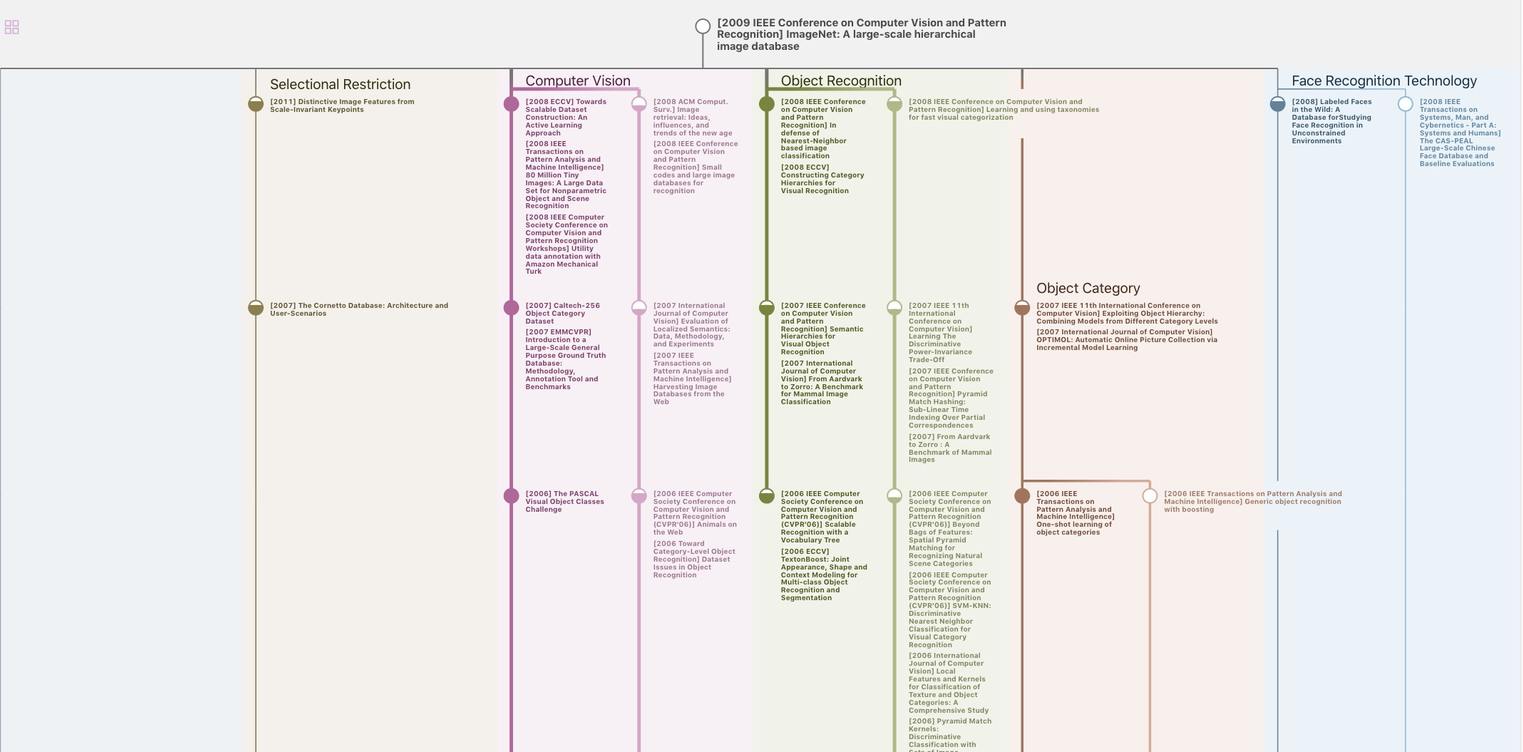
生成溯源树,研究论文发展脉络
Chat Paper
正在生成论文摘要