Self-Consistent Velocity Matching of Probability Flows
CoRR(2023)
摘要
We present a discretization-free scalable framework for solving a large class of mass-conserving partial differential equations (PDEs), including the time-dependent Fokker-Planck equation and the Wasserstein gradient flow. The main observation is that the time-varying velocity field of the PDE solution needs to be self-consistent: it must satisfy a fixed-point equation involving the flow characterized by the same velocity field. By parameterizing the flow as a time-dependent neural network, we propose an end-to-end iterative optimization framework called self-consistent velocity matching to solve this class of PDEs. Compared to existing approaches, our method does not suffer from temporal or spatial discretization, covers a wide range of PDEs, and scales to high dimensions. Experimentally, our method recovers analytical solutions accurately when they are available and achieves comparable or better performance in high dimensions with less training time compared to recent large-scale JKO-based methods that are designed for solving a more restrictive family of PDEs.
更多查看译文
关键词
flows,velocity,self-consistent
AI 理解论文
溯源树
样例
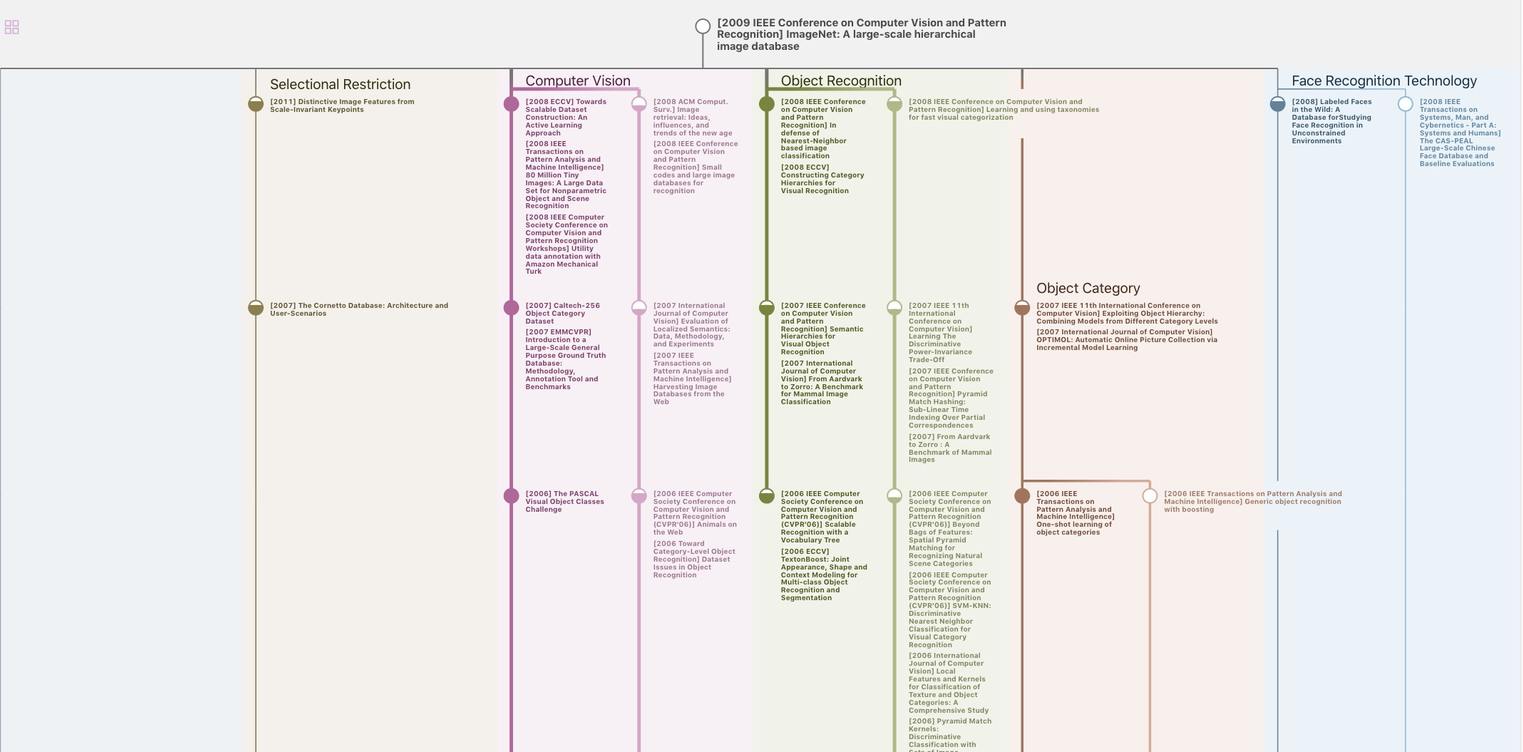
生成溯源树,研究论文发展脉络
Chat Paper
正在生成论文摘要