Guiding Online Reinforcement Learning with Action-Free Offline Pretraining
arxiv(2023)
摘要
Offline RL methods have been shown to reduce the need for environment interaction by training agents using offline collected episodes. However, these methods typically require action information to be logged during data collection, which can be difficult or even impossible in some practical cases. In this paper, we investigate the potential of using action-free offline datasets to improve online reinforcement learning, name this problem Reinforcement Learning with Action-Free Offline Pretraining (AFP-RL). We introduce Action-Free Guide (AF-Guide), a method that guides online training by extracting knowledge from action-free offline datasets. AF-Guide consists of an Action-Free Decision Transformer (AFDT) implementing a variant of Upside-Down Reinforcement Learning. It learns to plan the next states from the offline dataset, and a Guided Soft Actor-Critic (Guided SAC) that learns online with guidance from AFDT. Experimental results show that AF-Guide can improve sample efficiency and performance in online training thanks to the knowledge from the action-free offline dataset. Code is available at https://github.com/Vision-CAIR/AF-Guide.
更多查看译文
关键词
online reinforcement learning,pretraining,action-free
AI 理解论文
溯源树
样例
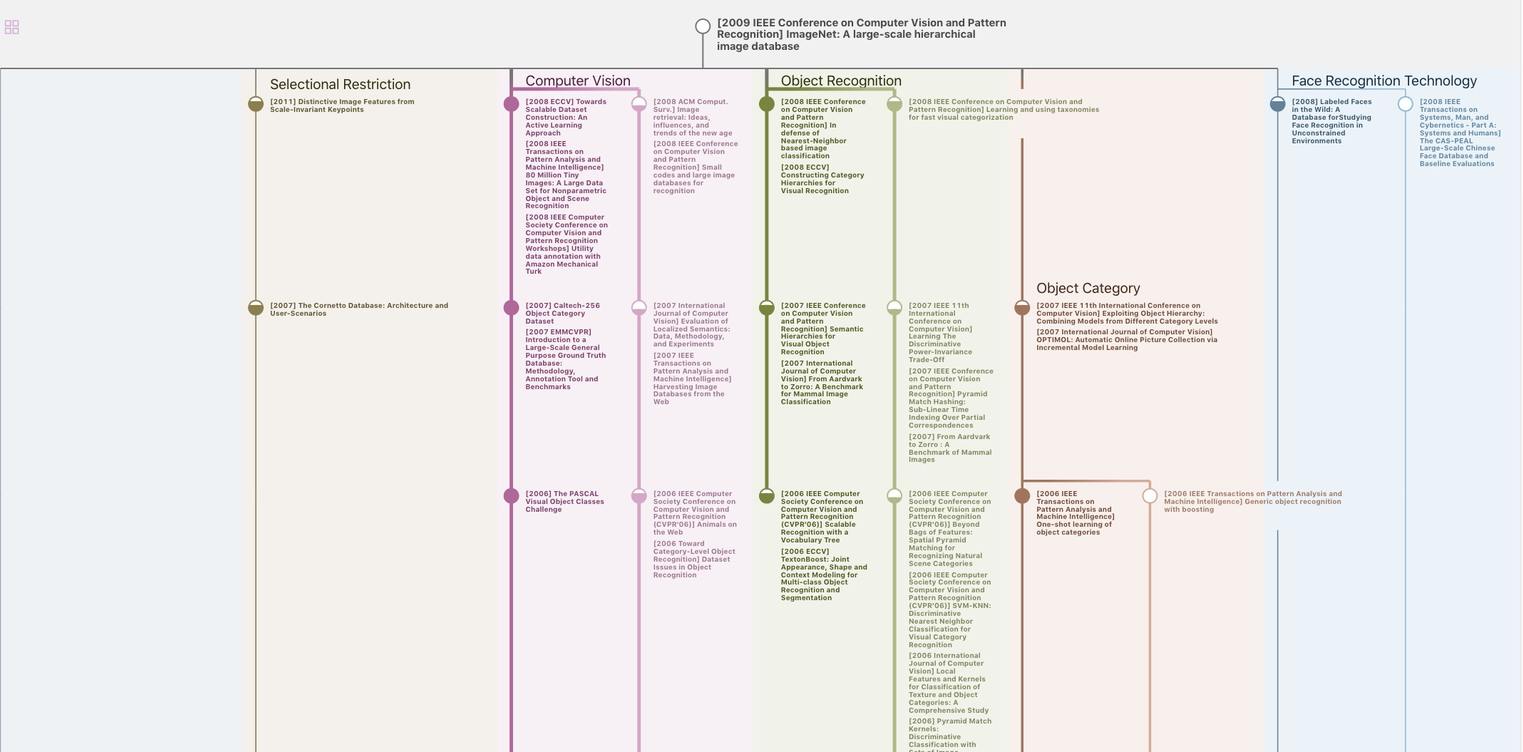
生成溯源树,研究论文发展脉络
Chat Paper
正在生成论文摘要