SpeechFormer++: A Hierarchical Efficient Framework for Paralinguistic Speech Processing
IEEE/ACM Transactions on Audio, Speech, and Language Processing(2023)
摘要
Paralinguistic speech processing is important in addressing many issues, such as sentiment and neurocognitive disorder analyses. Recently, Transformer has achieved remarkable success in the natural language processing field and has demonstrated its adaptation to speech. However, previous works on Transformer in the speech field have not incorporated the properties of speech, leaving the full potential of Transformer unexplored. In this paper, we consider the characteristics of speech and propose a general structure-based framework, called SpeechFormer++, for paralinguistic speech processing. More concretely, following the component relationship in the speech signal, we design a unit encoder to model the intra- and inter-unit information (
i.e.
, frames, phones, and words) efficiently. According to the hierarchical relationship, we utilize merging blocks to generate features at different granularities, which is consistent with the structural pattern in the speech signal. Moreover, a word encoder is introduced to integrate word-grained features into each unit encoder, which effectively balances fine-grained and coarse-grained information. SpeechFormer++ is evaluated on the speech emotion recognition (IEMOCAP & MELD), depression classification (DAIC-WOZ) and Alzheimer's disease detection (Pitt) tasks. The results show that SpeechFormer++ outperforms the standard Transformer while greatly reducing the computational cost. Furthermore, it delivers superior results compared to the state-of-the-art approaches.
更多查看译文
关键词
Neurocognitive disorder detection,paralinguistic speech processing,speech emotion recognition,transformer
AI 理解论文
溯源树
样例
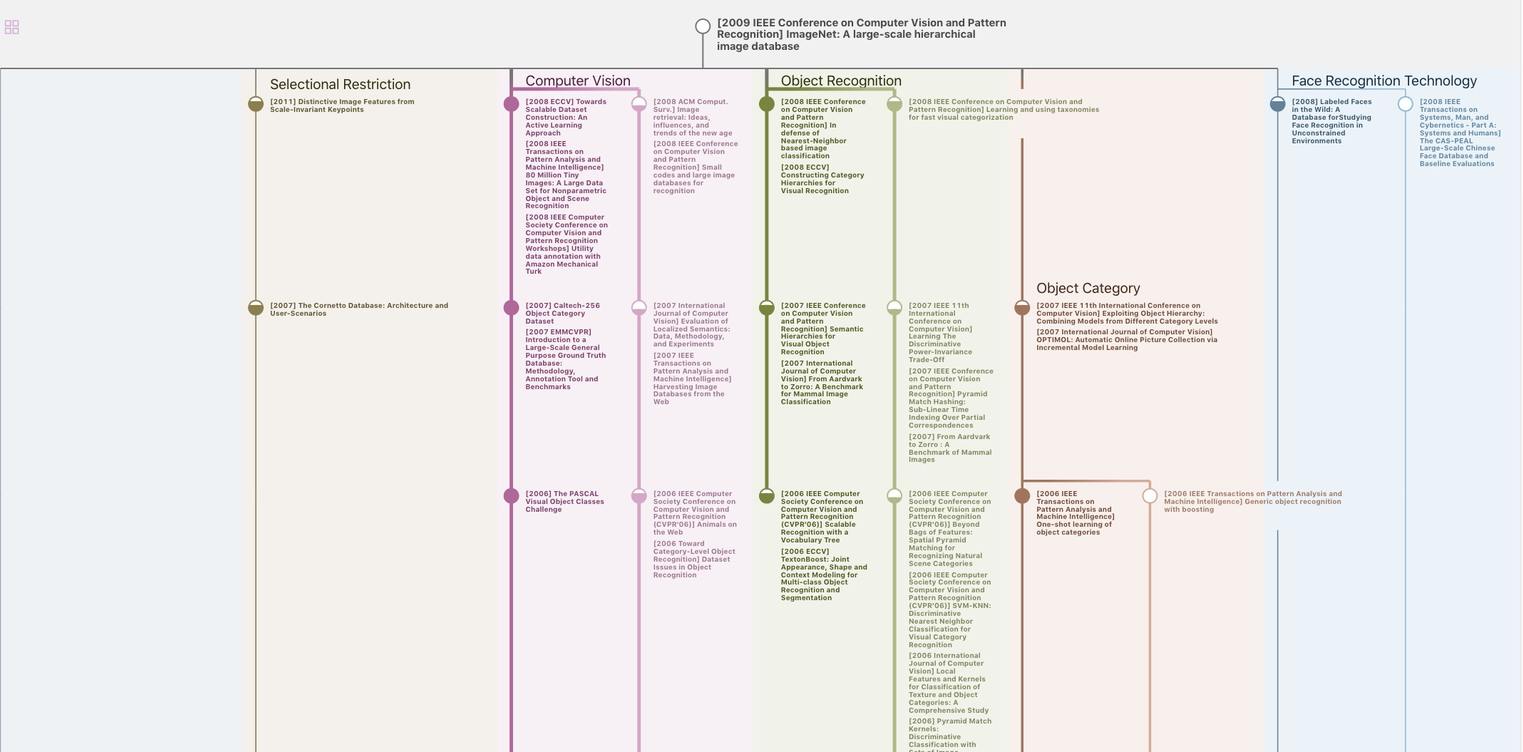
生成溯源树,研究论文发展脉络
Chat Paper
正在生成论文摘要