Feed-Forward Neural Network for Predicting Enantioselectivity of the Asymmetric Negishi Reaction
ACS CENTRAL SCIENCE(2023)
摘要
Density functional theory (DFT) is a powerful tool to model transition state (TS) energies to predict selectivity in chemical synthesis. However, a successful multistep synthesis campaign must navigate energetically narrow differences in pathways that create some limits to rapid and unambiguous application of DFT to these problems. While powerful data science techniques may provide a complementary approach to overcome this problem, doing so with the relatively small data sets that are widespread in organic synthesis presents a significant challenge. Herein, we show that a small data set can be labeled with features from DFT TS calculations to train a feed-forward neural network for predicting enantioselectivity of a Negishi cross-coupling reaction with P-chiral hindered phosphines. This approach to modeling enantioselectivity is compared with conventional approaches, including exclusive use of DFT energies and data science approaches, using features from ligands or ground states with neural network architectures.
更多查看译文
关键词
predicting enantioselectivity,asymmetric negishi reaction,neural network,feed-forward
AI 理解论文
溯源树
样例
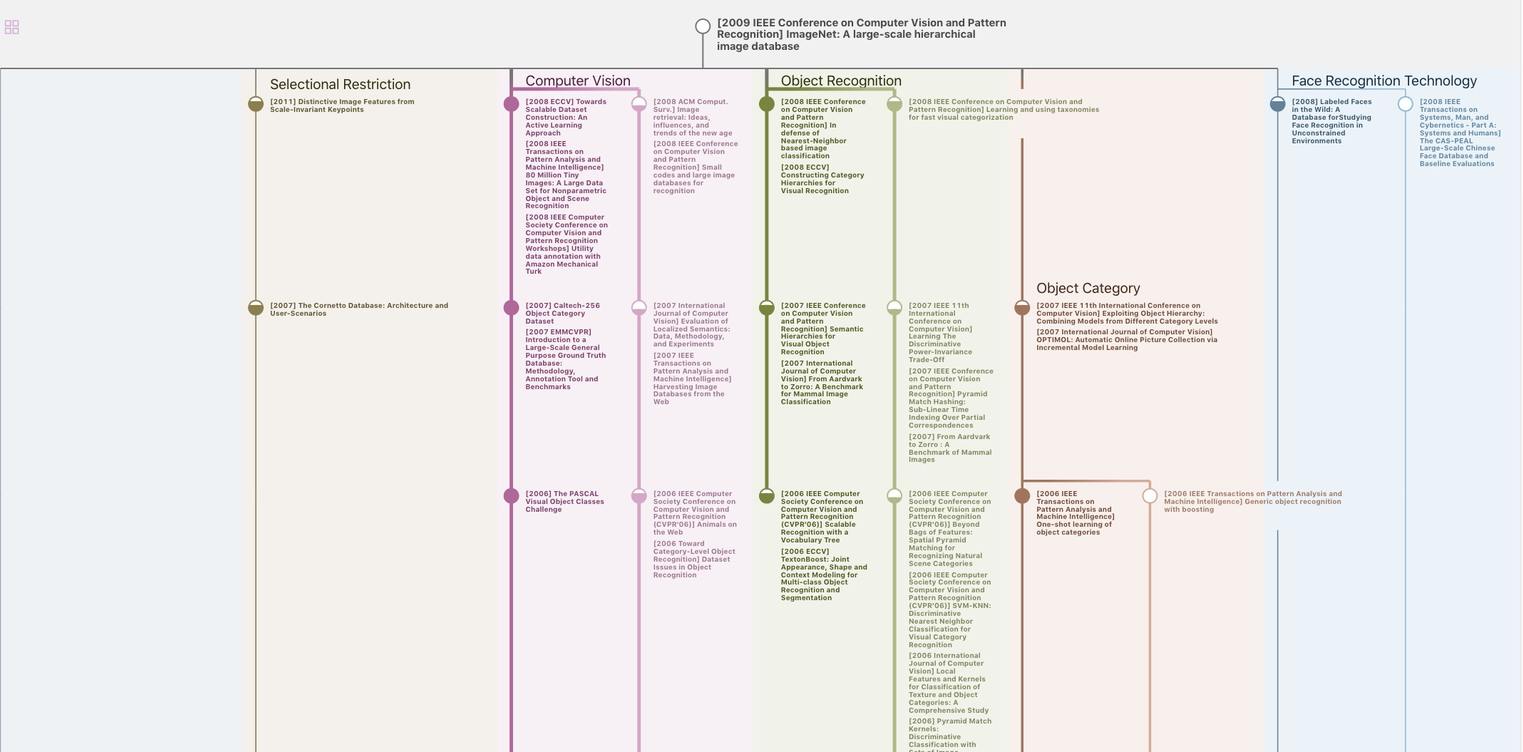
生成溯源树,研究论文发展脉络
Chat Paper
正在生成论文摘要