Granulation of Large Temporal Databases: An Allan Variance Approach
SN Computer Science(2022)
摘要
As the use of Big Data begins to dominate various scientific and engineering applications, the ability to conduct complex data analyses with speed and efficiency has become increasingly important. The availability of large amounts of data results in ever-growing storage requirements and magnifies issues related to query response times. In this work, we propose a novel methodology for granulation and data reduction of large temporal databases that can address both issues simultaneously. While prior data reduction techniques rely on heuristics or may be computationally intensive, our work borrows the concept of Allan Variance (AVAR) from the fields of signal processing and sensor characterization to efficiently and systematically reduce the size of temporal databases. Specifically, we use Allan variance to systematically determine the temporal window length over which data remains relevant. Large temporal databases are then granulated using the AVAR-determined window length. Averaging over the resulting granules produces aggregate information for each granule, resulting in significant data reduction. The query performance and data quality are evaluated using existing standard datasets, as well as for two large datasets that include temporal information for vehicular and weather data. Our results demonstrate that the AVAR-based data reduction approach is efficient and maintains data quality, while leading to an order of magnitude improvement in query execution times compared to three existing clustering-based data reduction methods.
更多查看译文
关键词
Big data,Data reduction,Temporal granulation,Allan variance
AI 理解论文
溯源树
样例
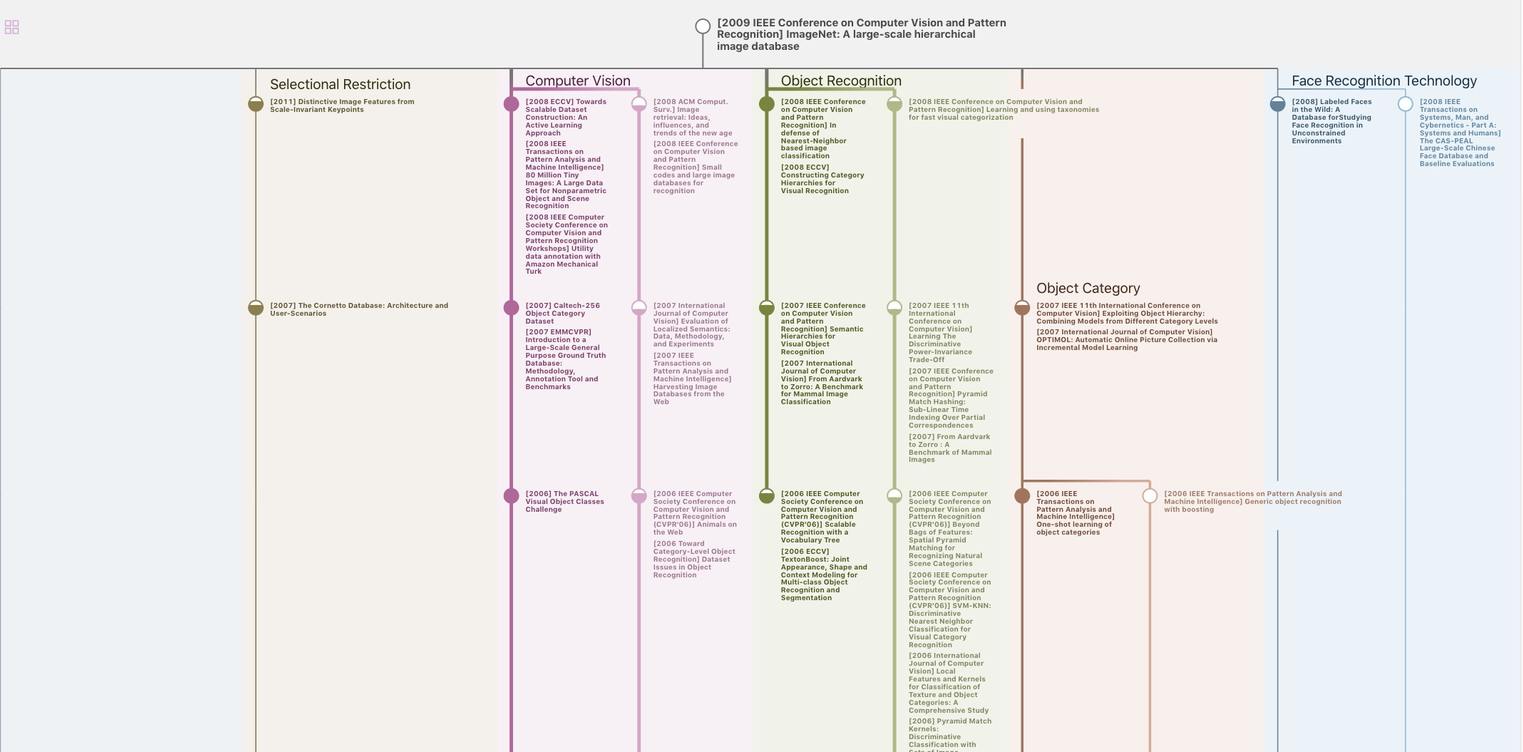
生成溯源树,研究论文发展脉络
Chat Paper
正在生成论文摘要