Hypernetworks for Zero-shot Transfer in Reinforcement Learning
arxiv(2023)
摘要
In this paper, hypernetworks are trained to generate behaviors across a range of unseen task conditions, via a novel TD-based training objective and data from a set of near-optimal RL solutions for training tasks. This work relates to meta RL, contextual RL, and transfer learning, with a particular focus on zero-shot performance at test time, enabled by knowledge of the task parameters (also known as context). Our technical approach is based upon viewing each RL algorithm as a mapping from the MDP specifics to the near-optimal value function and policy and seek to approximate it with a hypernetwork that can generate near-optimal value functions and policies, given the parameters of the MDP. We show that, under certain conditions, this mapping can be considered as a supervised learning problem. We empirically evaluate the effectiveness of our method for zero-shot transfer to new reward and transition dynamics on a series of continuous control tasks from DeepMind Control Suite. Our method demonstrates significant improvements over baselines from multitask and meta RL approaches.
更多查看译文
关键词
learning,reinforcement,transfer,zero-shot
AI 理解论文
溯源树
样例
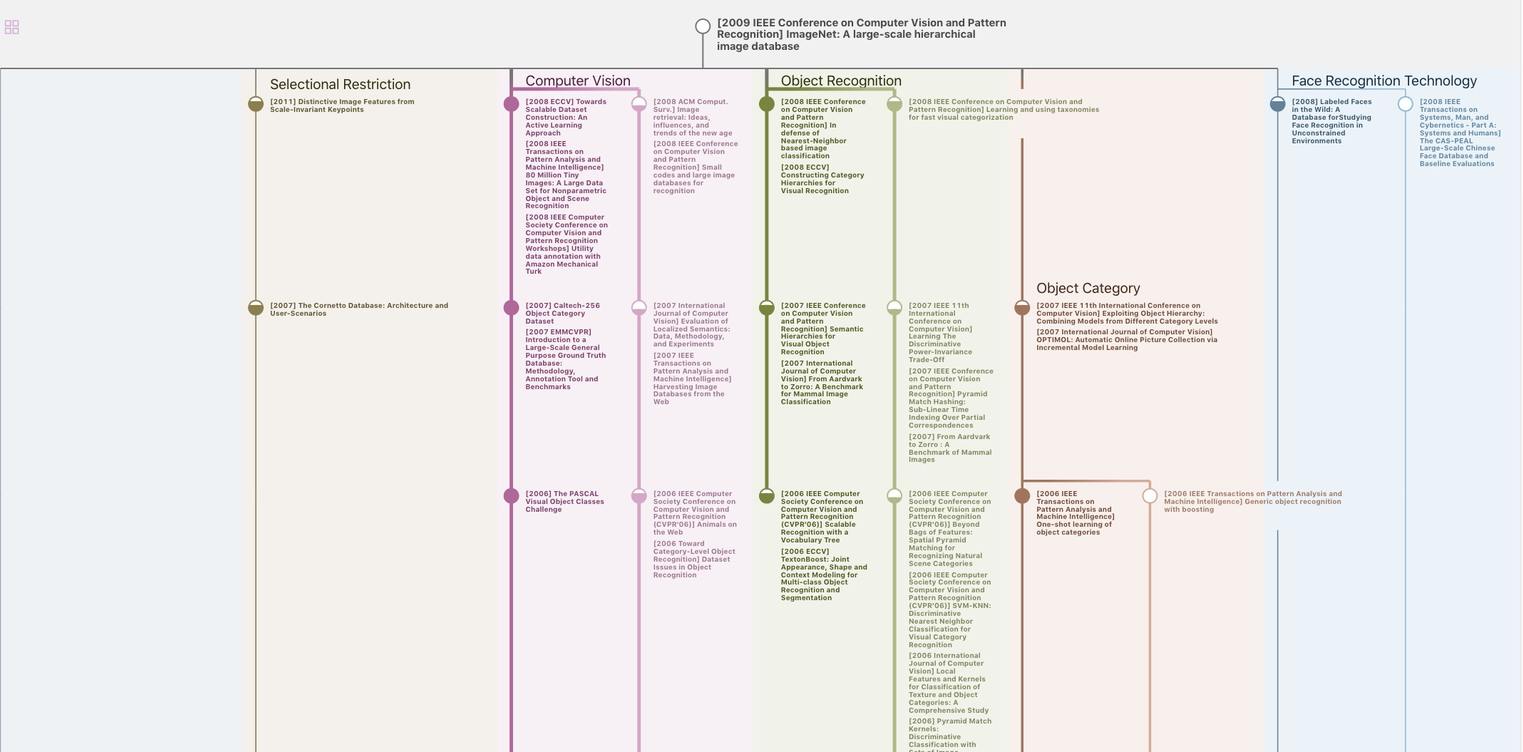
生成溯源树,研究论文发展脉络
Chat Paper
正在生成论文摘要