Is it time we get real? A systematic review of the potential of data-driven technologies to address teachers' implicit biases.
Frontiers in artificial intelligence(2022)
摘要
Data-driven technologies for education, such as artificial intelligence in education (AIEd) systems, learning analytics dashboards, open learner models, and other applications, are often created with an aspiration to help teachers make better, evidence-informed decisions in the classroom. Addressing gender, racial, and other biases inherent to data and algorithms in such applications is seen as a way to increase the responsibility of these systems and has been the focus of much of the research in the field, including systematic reviews. However, implicit biases can also be held by teachers. To the best of our knowledge, this systematic literature review is the first of its kind to investigate what kinds of teacher biases have been impacted by data-driven technologies, how or if these technologies were designed to challenge these biases, and which strategies were most effective at promoting equitable teaching behaviors and decision making. Following PRISMA guidelines, a search of five databases returned = 359 records of which only = 2 studies by a single research team were identified as relevant. The findings show that there is minimal evidence that data-driven technologies have been evaluated in their capacity for supporting teachers to make less biased decisions or promote equitable teaching behaviors, even though this capacity is often used as one of the core arguments for the use of data-driven technologies in education. By examining these two studies in conjunction with related studies that did not meet the eligibility criteria during the full-text review, we reveal the approaches that could play an effective role in mitigating teachers' biases, as well as ones that may perpetuate biases. We conclude by summarizing directions for future research that should seek to directly confront teachers' biases through explicit design strategies within teacher tools, to ensure that the impact of biases of both technology (including data, algorithms, models etc.) teachers are minimized. We propose an extended framework to support future research and design in this area, through motivational, cognitive, and technological debiasing strategies.
更多查看译文
关键词
artificial intelligence in education,bias,decision support systems,equity,learning analytics (LA),teachers
AI 理解论文
溯源树
样例
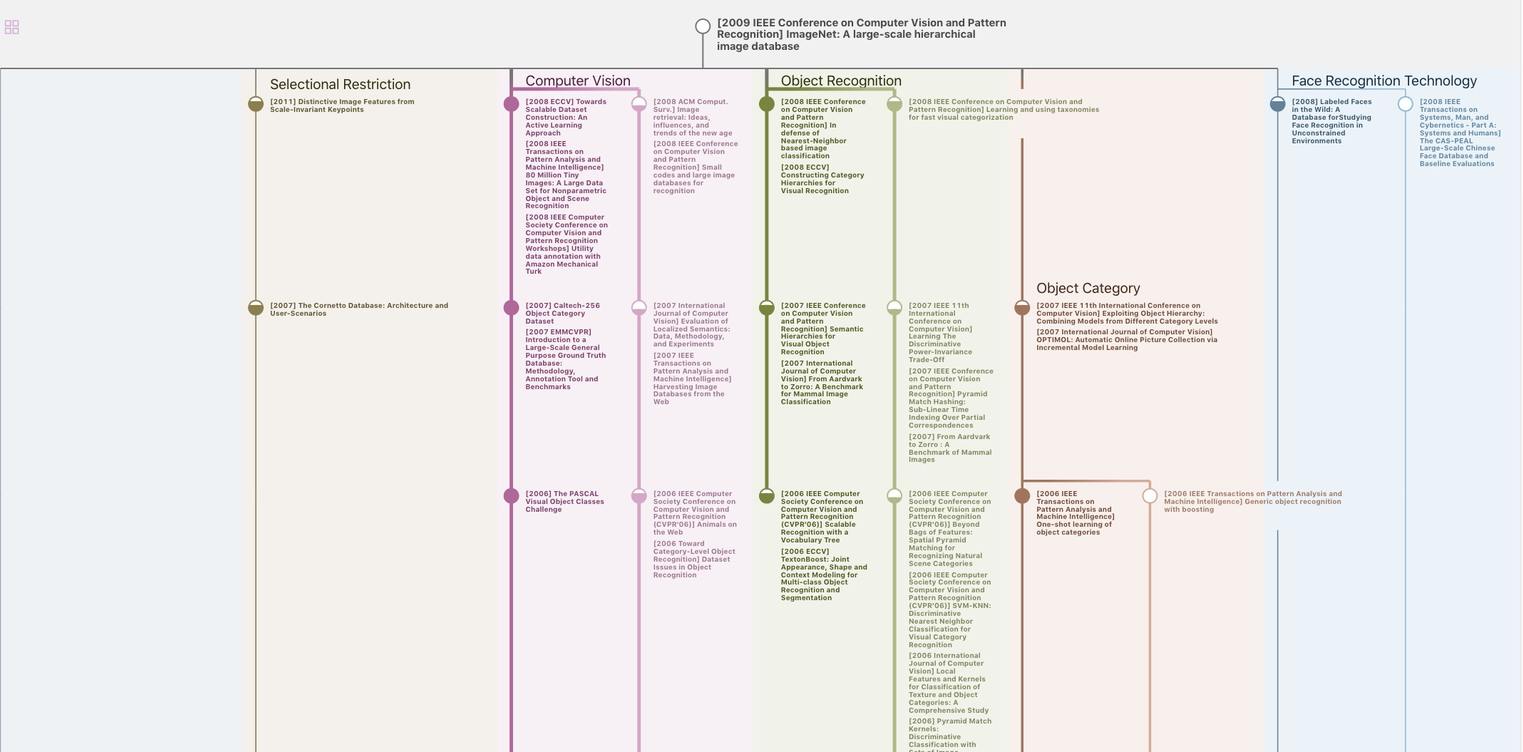
生成溯源树,研究论文发展脉络
Chat Paper
正在生成论文摘要