A Pipe Ultrasonic Guided Wave Signal Generation Network Suitable for Data Enhancement in Deep Learning: US-WGAN
ENERGIES(2022)
摘要
A network ultrasonic Wasserstein generative adversarial network (US-WGAN), which can generate ultrasonic guided wave signals, is proposed herein to solve the problem of insufficient datasets for pipe ultrasonic nondestructive testing based on deep neural networks. This network was trained with pre-enhanced and US-WGAN-enhanced datasets with 3000 epochs; the ultrasound signals generated by the US-WGAN were proved to be of high quality (peak signal-to-noise ratio scores in the range of 30-50 dB) and belong to the same population distribution as the original dataset. To verify the effectiveness of the US-WGAN, a fully connected neural network with seven layers was established, and the performances of the network after data enhancement using the US-WGAN and popular virtual defects were verified for the same network parameters and structures. The results show that adoption of the US-WGAN effectively suppresses the overfitting phenomenon while training the network and increases the dataset size, thereby improving the training and testing accuracies (>97%). Additionally, we noted that a simple, fully connected shallow neural network was sufficient for achieving high-accuracy defect classification using the US-WGAN data enhancement method.
更多查看译文
关键词
EMAT, Wasserstein generative adversarial network, data enhancement, ultrasonic guided wave testing, deep learning, defect classification
AI 理解论文
溯源树
样例
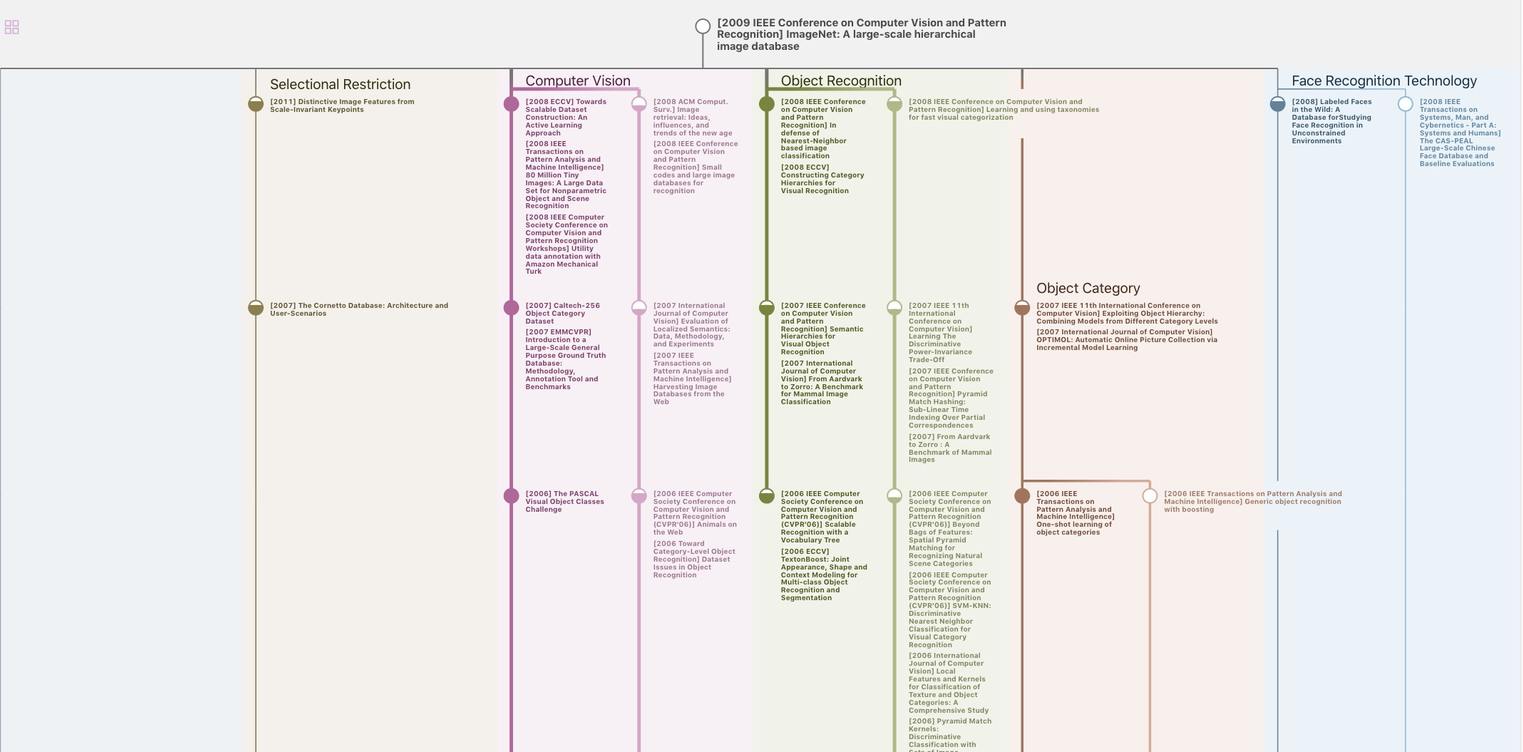
生成溯源树,研究论文发展脉络
Chat Paper
正在生成论文摘要