Domain Adaptation in 3D Object Detection with Gradual Batch Alternation Training
arxiv(2022)
摘要
We consider the problem of domain adaptation in LiDAR-based 3D object detection. Towards this, we propose a simple yet effective training strategy called Gradual Batch Alternation that can adapt from a large labeled source domain to an insufficiently labeled target domain. The idea is to initiate the training with the batch of samples from the source and target domain data in an alternate fashion, but then gradually reduce the amount of the source domain data over time as the training progresses. This way the model slowly shifts towards the target domain and eventually better adapt to it. The domain adaptation experiments for 3D object detection on four benchmark autonomous driving datasets, namely ONCE, PandaSet, Waymo, and nuScenes, demonstrate significant performance gains over prior arts and strong baselines.
更多查看译文
关键词
domain adaptation,3d object detection,object detection
AI 理解论文
溯源树
样例
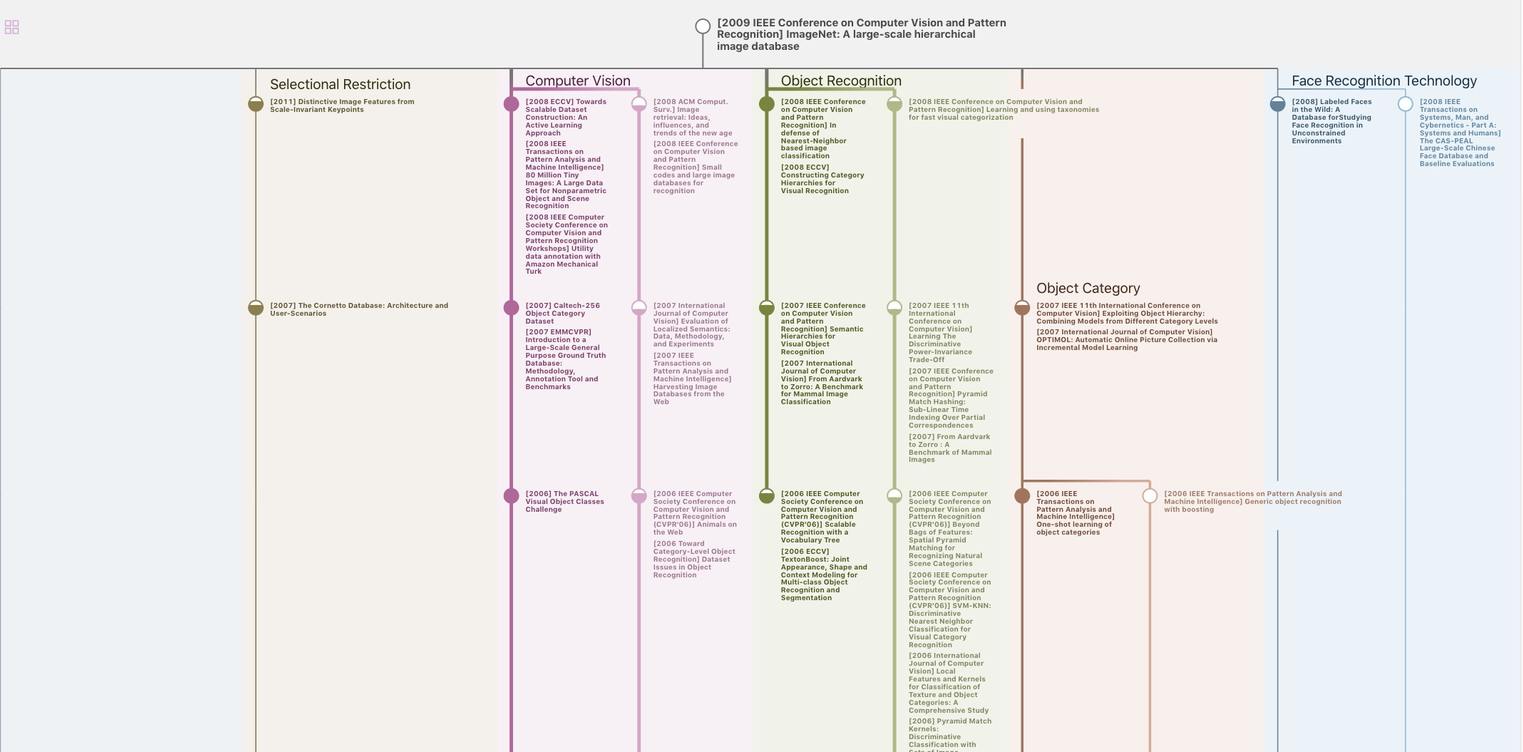
生成溯源树,研究论文发展脉络
Chat Paper
正在生成论文摘要