StyleFace: Towards Identity-Disentangled Face Generation on Megapixels.
European Conference on Computer Vision(2022)
摘要
Identity swapping and de-identification are two essential applications of identity-disentangled face image generation. Although sharing a similar problem definition, the two tasks have been long studied separately, and identity-disentangled face generation on megapixels is still under exploration. In this work, we propose StyleFace, a unified framework for 1024(2) resolution high-fidelity identity swapping and de-identification. To encode real identity while supporting virtual identity generation, we represent identity as a latent variable and further utilize contrastive learning for latent space regularization. Besides, we utilize StyleGAN2 to improve the generation quality on megapixels and devise an Adaptive Attribute Extractor, which adaptively preserves the identity-irrelevant attributes in a simple yet effective way. Extensive experiments demonstrate the state-of-the-art performance of StyleFace in high-resolution identity swapping and de-identification.
更多查看译文
AI 理解论文
溯源树
样例
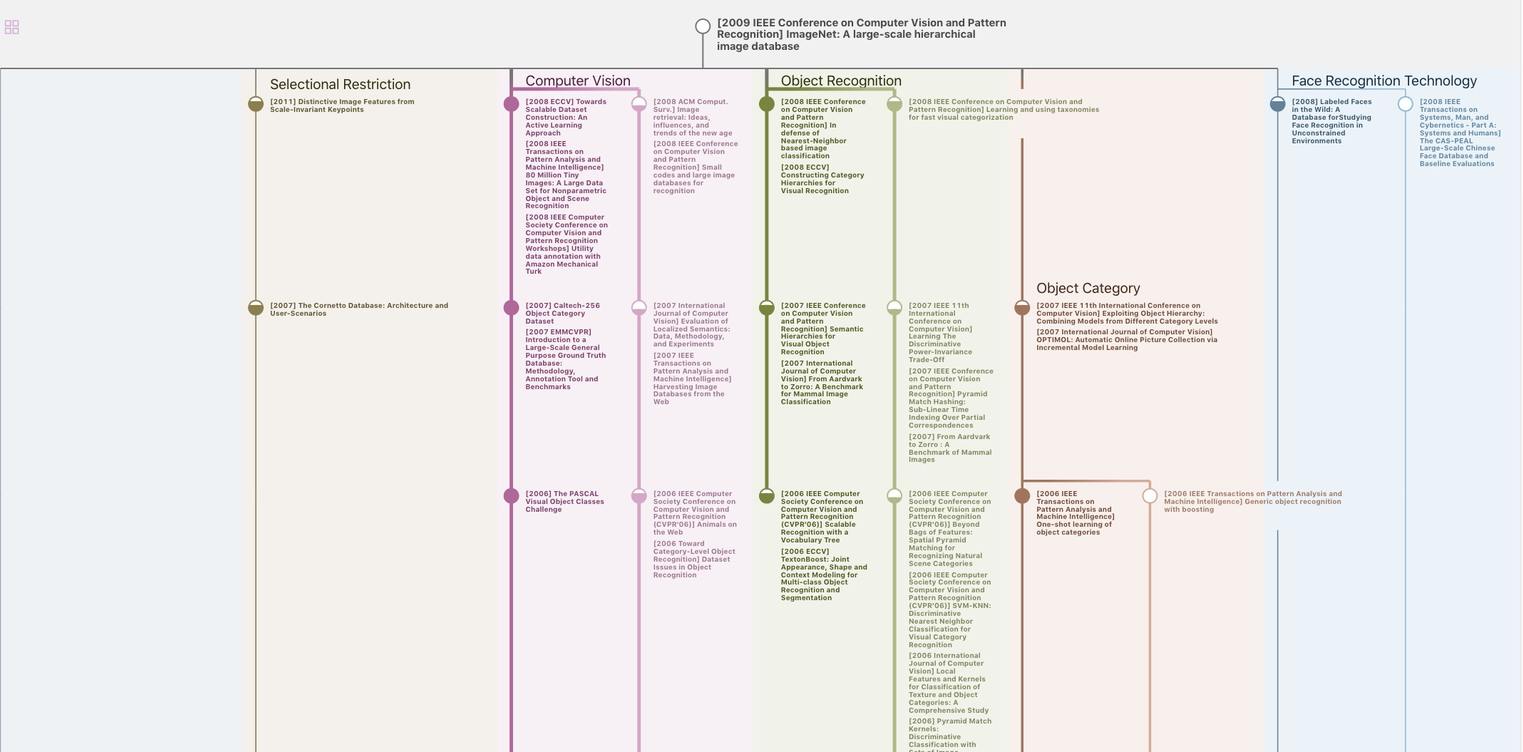
生成溯源树,研究论文发展脉络
Chat Paper
正在生成论文摘要