The Gibbs-Rand Model
PROCEEDINGS OF THE 41ST ACM SIGMOD-SIGACT-SIGAI SYMPOSIUM ON PRINCIPLES OF DATABASE SYSTEMS (PODS '22)(2022)
摘要
Due to its many applications, the clustering ensemble problem has been subject of intense algorithmic study over the last two decades. The input to this problem is a set of clusterings; its goal is to output a clustering that minimizes the average distance to the input clusterings. In this paper, we propose, to the best of our knowledge, the first generative model for this problem. Our Gibbs-like model is parameterized by a center clustering, and by a scale; the probability of a particular clustering decays exponentially with its scaled Rand distance to the center clustering. For our new model, we give polynomial-time algorithms for center dot sampling, when the center clustering has a constant number of clusters and center dot reconstruction, when the scale parameter is small. En route, we establish several interesting properties of our model. Our work shows that the combinatorial structure of a Gibbs-like model for clusterings is more intricate and challenging than the corresponding and well-studied (Mallows) model for permutations.
更多查看译文
关键词
Clustering, Gibbs model, Rand distance
AI 理解论文
溯源树
样例
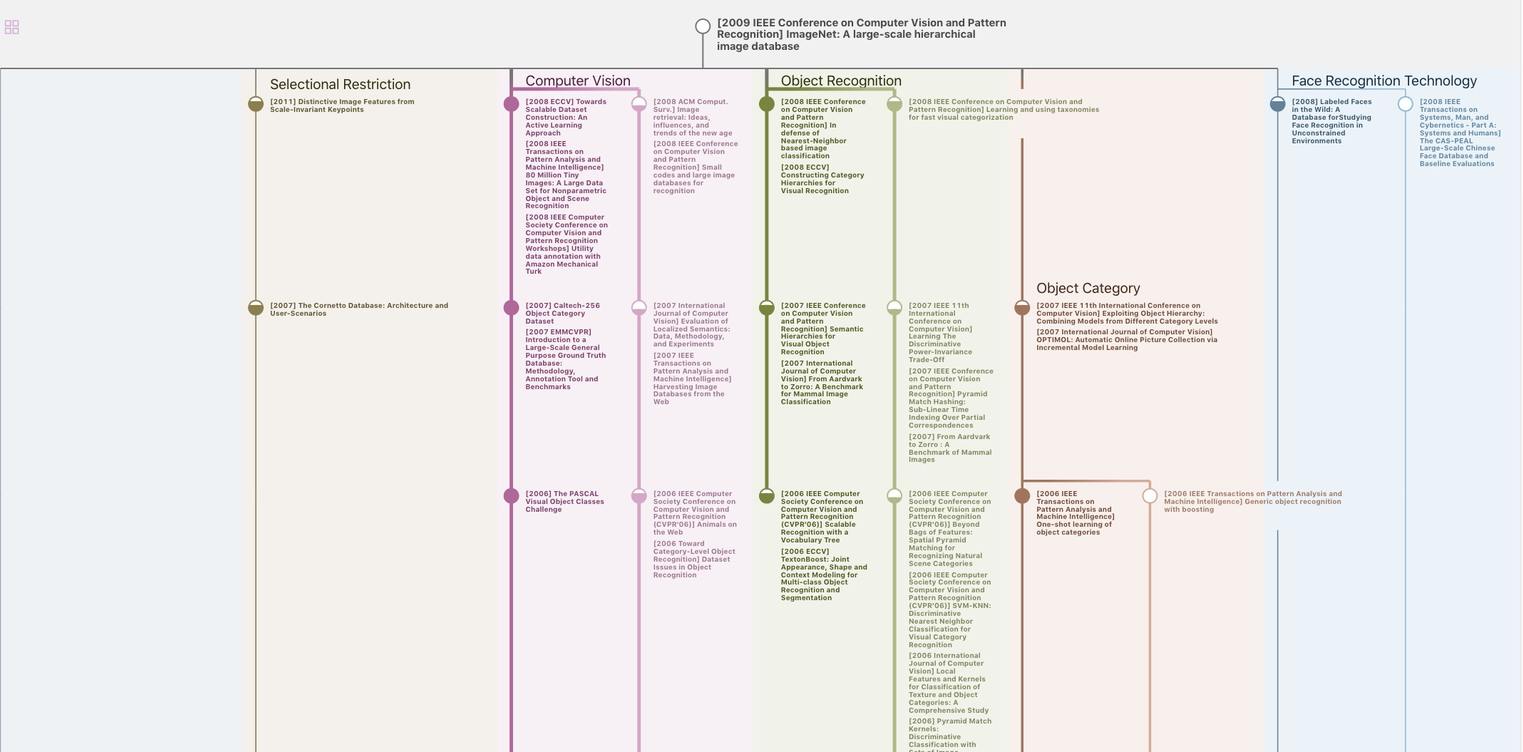
生成溯源树,研究论文发展脉络
Chat Paper
正在生成论文摘要