SHINE-Mapping: Large-Scale 3D Mapping Using Sparse Hierarchical Implicit Neural Representations
arxiv(2023)
摘要
Accurate mapping of large-scale environments is an essential building block of most outdoor autonomous systems. Challenges of traditional mapping methods include the balance between memory consumption and mapping accuracy. This paper addresses the problem of achieving large-scale 3D reconstruction using implicit representations built from 3D LiDAR measurements. We learn and store implicit features through an octree-based, hierarchical structure, which is sparse and extensible. The implicit features can be turned into signed distance values through a shallow neural network. We leverage binary cross entropy loss to optimize the local features with the 3D measurements as supervision. Based on our implicit representation, we design an incremental mapping system with regularization to tackle the issue of forgetting in continual learning. Our experiments show that our 3D reconstructions are more accurate, complete, and memory-efficient than current state-of-the-art 3D mapping methods.
更多查看译文
关键词
sparse hierarchical implicit
AI 理解论文
溯源树
样例
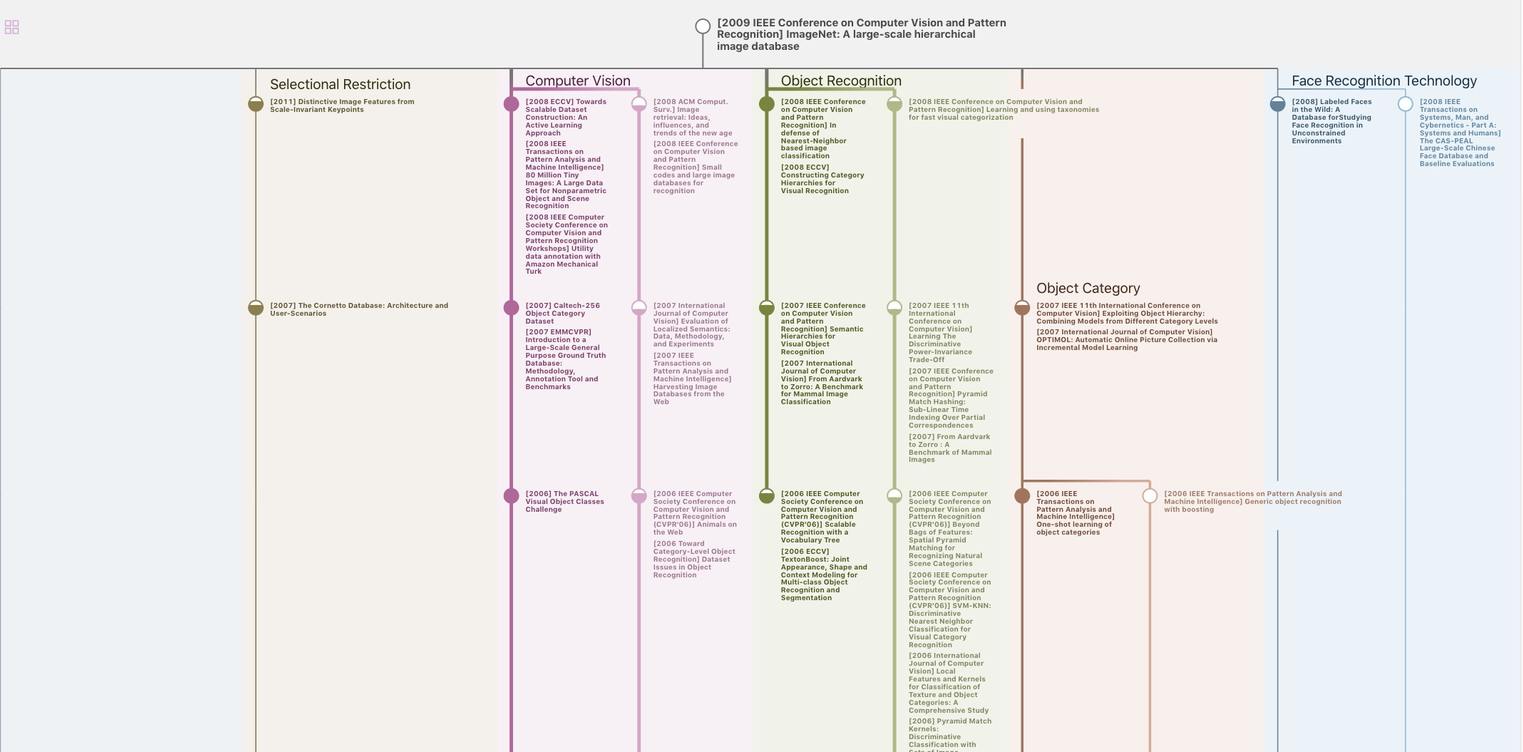
生成溯源树,研究论文发展脉络
Chat Paper
正在生成论文摘要