Deep Learning for Satellites Based Spectrum Sensing Systems: A Low Computational Complexity Perspective.
IEEE Trans. Veh. Technol.(2023)
摘要
We investigate a satellites-based spectrum sensing system in the presence of low signal-to-noise ratio (SNR) conditions. Such a low SNR is also called SNR wall in the energy detection (ED) method. To eliminate the SNR-wall effect, we propose a combined convolutional neural network and long short-term memory (C-CNN-LSTM) aided spectrum-sensing scheme. Specifically, the CNN and the LSTM are concurrently utilized, where the CNN extracts relationships among spectrum-sensing data (SSD) received at different satellites, and the LSTM excavates time-domain relationships among SSD from one satellite. Then, the outputs of the CNN and the LSTM will be combined. Performance evaluations indicate that the C-CNN-LSTM outperforms the CNN and the ED methods in terms of a higher probability of correct detection (P-d) and a lower probability of false alarm (P-f). Moreover, the C-CNN-LSTM can achieve a bit better P-d versus Pf than that of the cooperative detection DetectNets, which requires multiple DetectNets deployed on multiple sensing nodes, and is used for comparison purposes. These beneficial results demonstrate the superiority of the C-CNN-LSTM in terms of a lower implementation and computational complexity having a high Pd and a low P-f.
更多查看译文
关键词
Sensors,Satellites,Convolutional neural networks,Training,Signal to noise ratio,Logic gates,Data models,Deep learning,likelihood ratio,multiple satellites,spectrum sensing
AI 理解论文
溯源树
样例
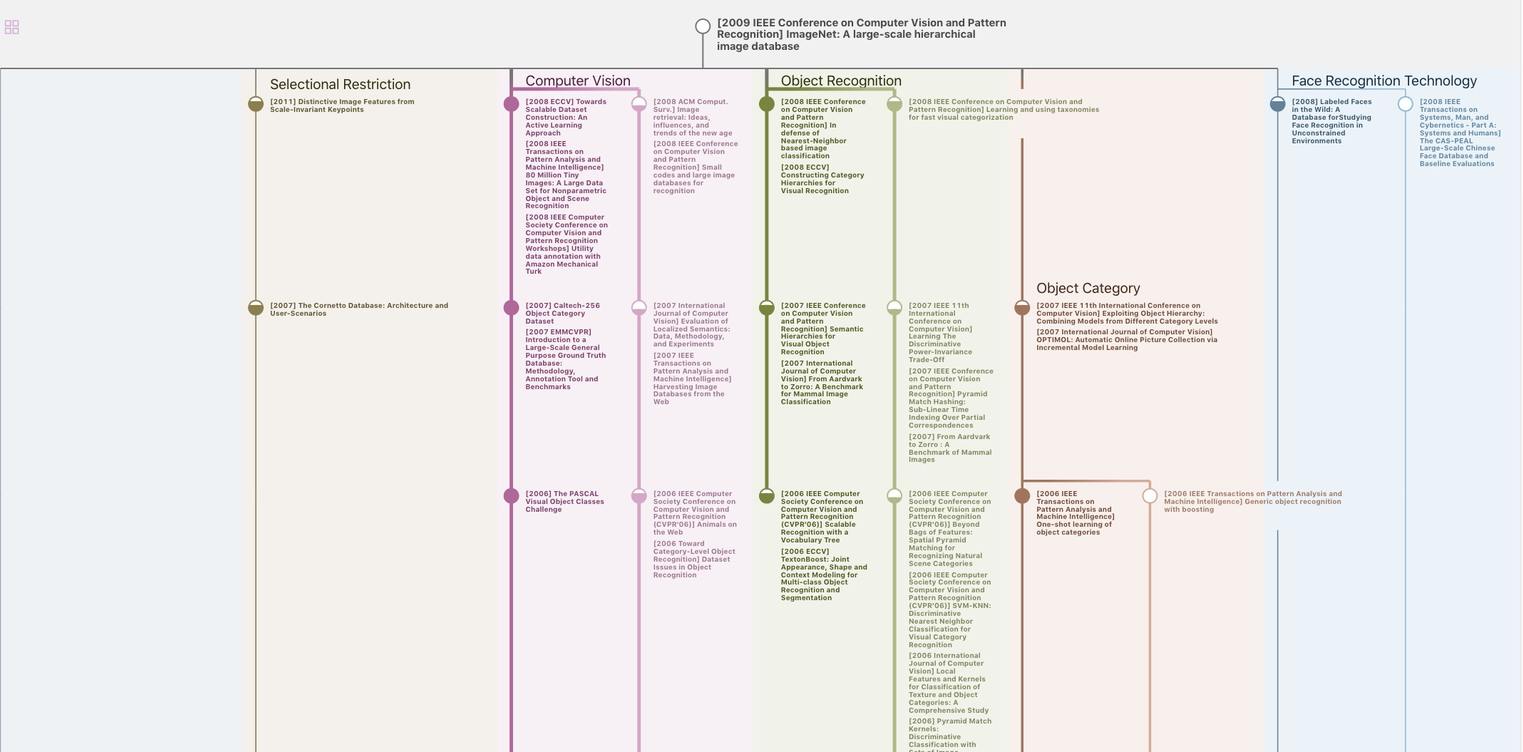
生成溯源树,研究论文发展脉络
Chat Paper
正在生成论文摘要