Measuring Noticeability: Multi-scale Context Aggregation for Prioritizing Video Anomalies.
IEEE International Joint Conference on Neural Network (IJCNN)(2022)
摘要
Determining how impactful an anomaly is on the viewing experience of an audience is important to production studios, content creators, and content distributors. However, judging the impact of each anomaly is a highly manual and subjective task. To support automation of this, we propose the use of noticeability and introduce a method of prediction. We employed a psychophysical experimental method to capture the impact of various anomalies across various images. We then developed a multi-scale context aggregation model, trained on that data, to predict the noticeability of anomalies on novel images. This noticeability prediction can then be used to prioritize anomalies and ensure that remediation efforts are spent where it would most benefit the audience experience.
更多查看译文
关键词
Video Quality Control,Just Noticeable Difference (JND),Local Sensitivity,Deep Learning
AI 理解论文
溯源树
样例
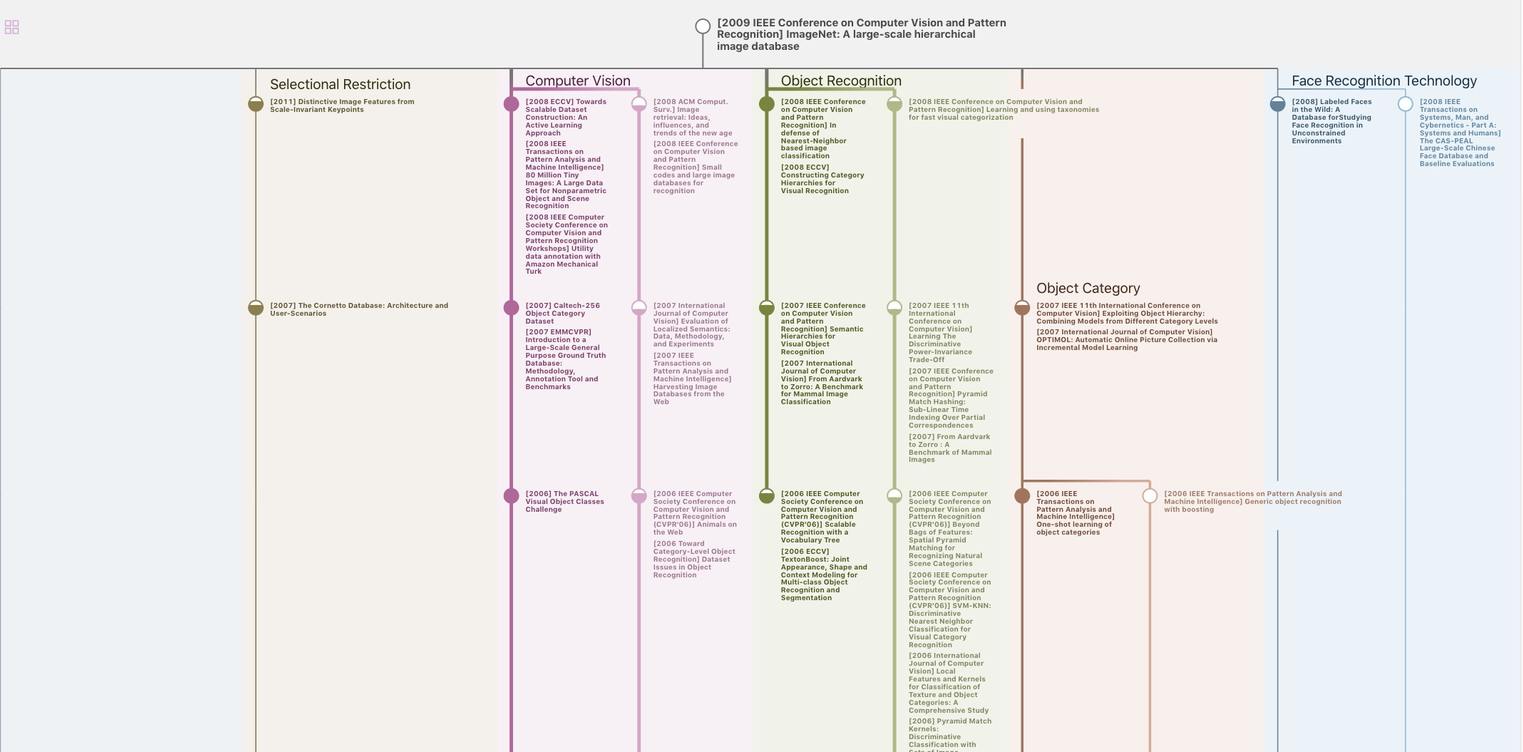
生成溯源树,研究论文发展脉络
Chat Paper
正在生成论文摘要