A Novel Gradient Accumulation Method for Calibration of Named Entity Recognition Models
IEEE International Joint Conference on Neural Network (IJCNN)(2022)
摘要
The adoption of deep learning models has brought significant performance improvements across several research fields, such as computer vision and natural language processing. However, their "black-box" nature yields the downside of poor explainability: in particular, several real-world applications require - to varying extents - reliable confidence scores associated to a model's prediction. The relation between a model's accuracy and confidence is typically referred to as calibration. In this work, we propose a novel calibration method based on gradient accumulation in conjunction with existing loss regularization techniques. Our experiments on the Named Entity Recognition task show an improvement of the performance/calibration ratio compared to the current methods.
更多查看译文
关键词
calibration,ner,uncertainty,noise injection
AI 理解论文
溯源树
样例
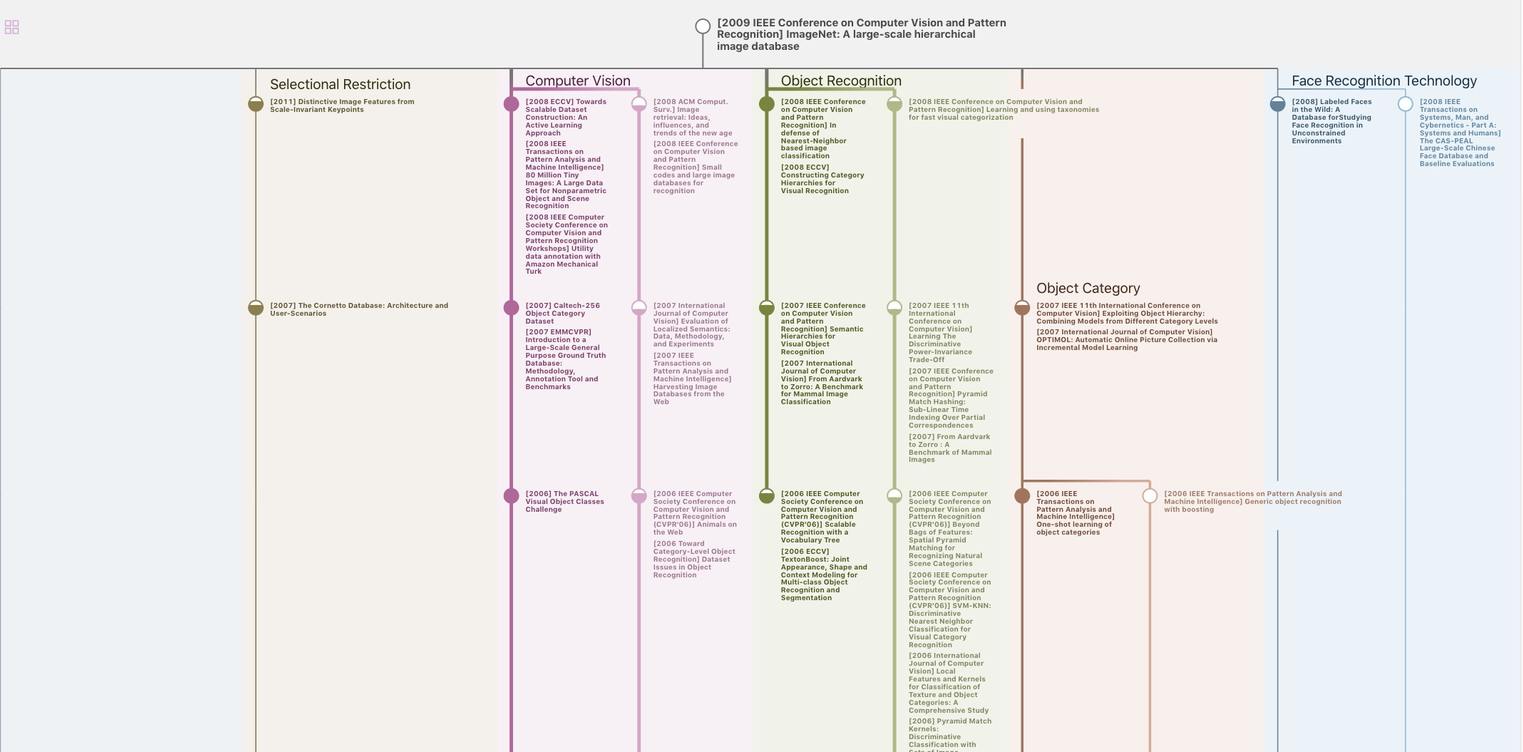
生成溯源树,研究论文发展脉络
Chat Paper
正在生成论文摘要