Explore Adversarial Attack via Black Box Variational Inference
IEEE SIGNAL PROCESSING LETTERS(2022)
摘要
From the perspective of probability, we propose a new method for black-box adversarial attack via black-box variational inference (BBVI), where the knowledge of victim model is unavailable. Instead of obtaining a single point, the proposed method focuses on approximating the probability distribution of adversarial examples. Thus, infinite adversarial examples can be drawn from the inferred distribution. Although the Monte Carlo estimator in BBVI is unbiased, its variance brings unstable gradient estimation, which leads to poor attack performance and low query efficiency. To reduce variance, we improve the BBVI with importance sampling which guided by a surrogate model to obtain a better estimator of gradient, which enhances both success rate and query efficiency. Extensive experiments on ImageNet dataset well demonstrate the outperformance of the proposed method compared with prior arts.
更多查看译文
关键词
Monte Carlo methods,Computational modeling,Probability distribution,Gaussian distribution,Bayes methods,Art,Stochastic processes,Adversarial attack,importance sampling,Bayesian inference
AI 理解论文
溯源树
样例
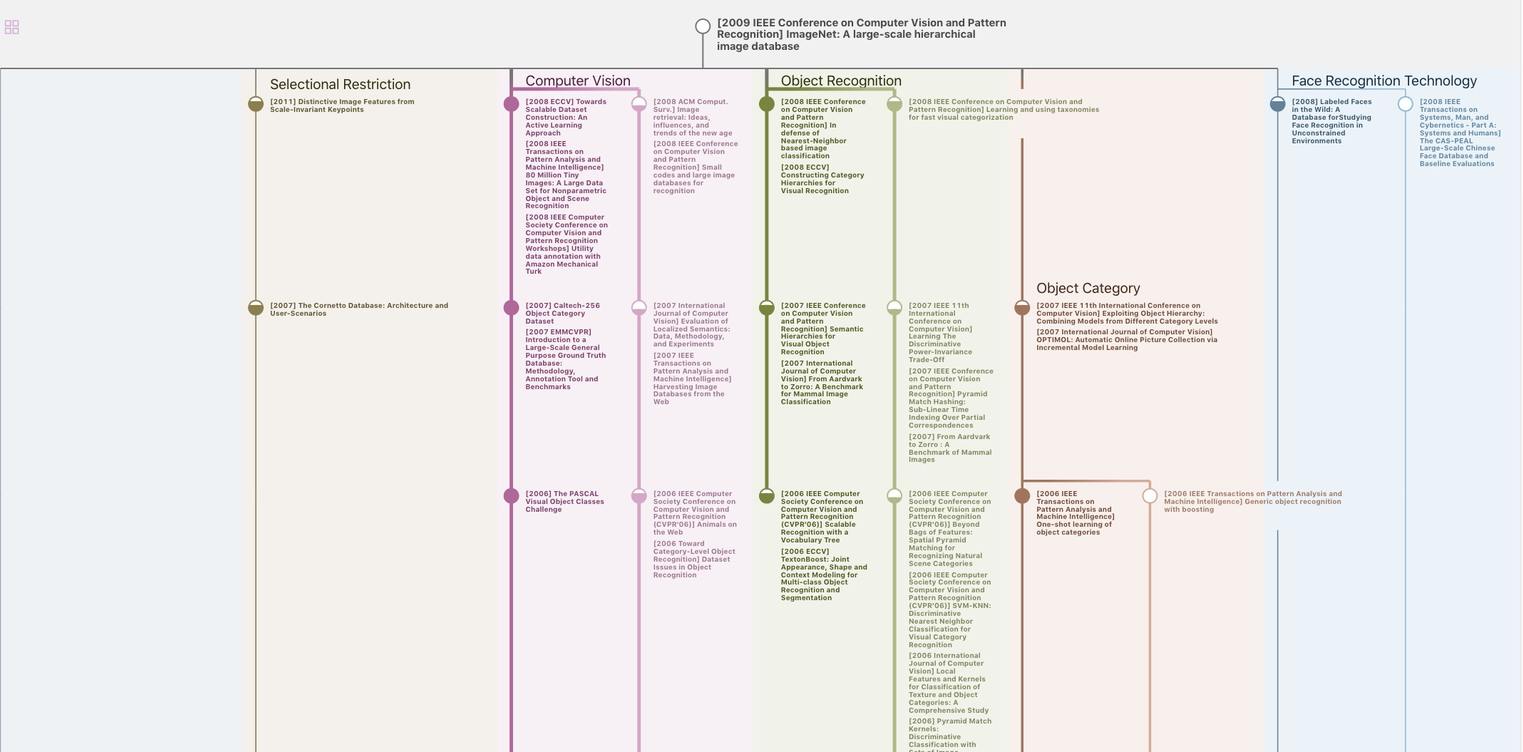
生成溯源树,研究论文发展脉络
Chat Paper
正在生成论文摘要