Non-iterative Conditional Pairwise Estimation for the Rating Scale Model
EDUCATIONAL AND PSYCHOLOGICAL MEASUREMENT(2022)
摘要
We investigate two non-iterative estimation procedures for Rasch models, the pair-wise estimation procedure (PAIR) and the Eigenvector method (EVM), and identify theoretical issues with EVM for rating scale model (RSM) threshold estimation. We develop a new procedure to resolve these issues-the conditional pairwise adjacent thresholds procedure (CPAT)-and test the methods using a large number of simulated datasets to compare the estimates against known generating parameters. We find support for our hypotheses, in particular that EVM threshold estimates suffer from theoretical issues which lead to biased estimates and that CPAT represents a means of resolving these issues. These findings are both statistically significant (p < .001) and of a large effect size. We conclude that CPAT deserves serious consideration as a conditional, computationally efficient approach to Rasch parameter estimation for the RSM. CPAT has particular potential for use in contexts where computational load may be an issue, such as systems with multiple online algorithms and large test banks with sparse data designs.
更多查看译文
关键词
Rasch, rating scale model, estimation, pairwise, simulation
AI 理解论文
溯源树
样例
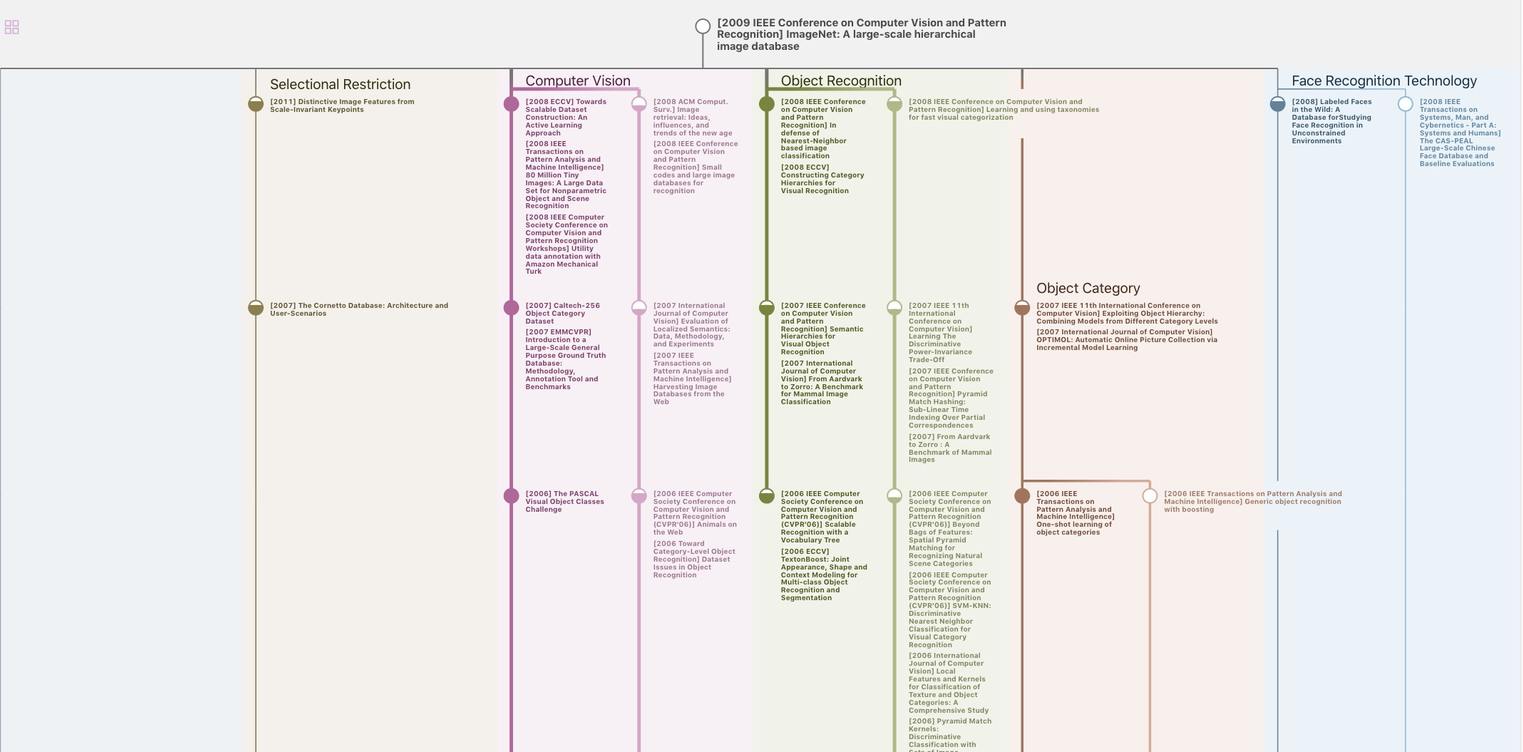
生成溯源树,研究论文发展脉络
Chat Paper
正在生成论文摘要