Medical Symptom Detection in Intelligent Pre-Consultation Using Bi-directional Hard-Negative Noise Contrastive Estimation
KDD '22: Proceedings of the 28th ACM SIGKDD Conference on Knowledge Discovery and Data Mining(2022)
摘要
Leveraging artificial intelligence (AI) techniques in medical applications is helping our world to deal with the shortage of healthcare workers and improve the efficiency and productivity of healthcare delivery. Intelligent pre-consultation (IPC) is a relatively new application deployed on mobile terminals for collecting patient's information before a face-to-face consultation. It takes advantages of state-of-the-art machine learning techniques to assist doctors on clinical decision-making. One of key functions of IPC is to detect medical symptoms from patient queries. By extracting symptoms from patient queries, IPC is able to collect more information on patient's health status by asking symptom-related questions. All collected information will be summarized as a medical record for doctors to make clinical decision. This saves a great deal of time for both doctors and patients. Detecting symptoms from patient's query is challenging, as most patients lack medical background and often tend to use colloquial language to describe their symptoms. In this work, we formulate symptom detection as a retrieval problem and propose a bi-directional hard-negative enforced noise contrastive estimation method (Bi-hardNCE) to tackle the symptom detection problem. Bi-hardNCE has both forward contrastive estimation and backward contrastive estimation, which forces model to distinguish the true symptom from negative symptoms and meanwhile distinguish true query from negative queries. To include more informative negatives, our Bi-hardNCE adopts a hard-negative mining strategy and a false-negative eliminating strategy, which achieved a significant improvement on performance. Our proposed model outperforms commonly used retrieval models by a large margin.
更多查看译文
关键词
contrastive,noise,pre-consultation,bi-directional,hard-negative
AI 理解论文
溯源树
样例
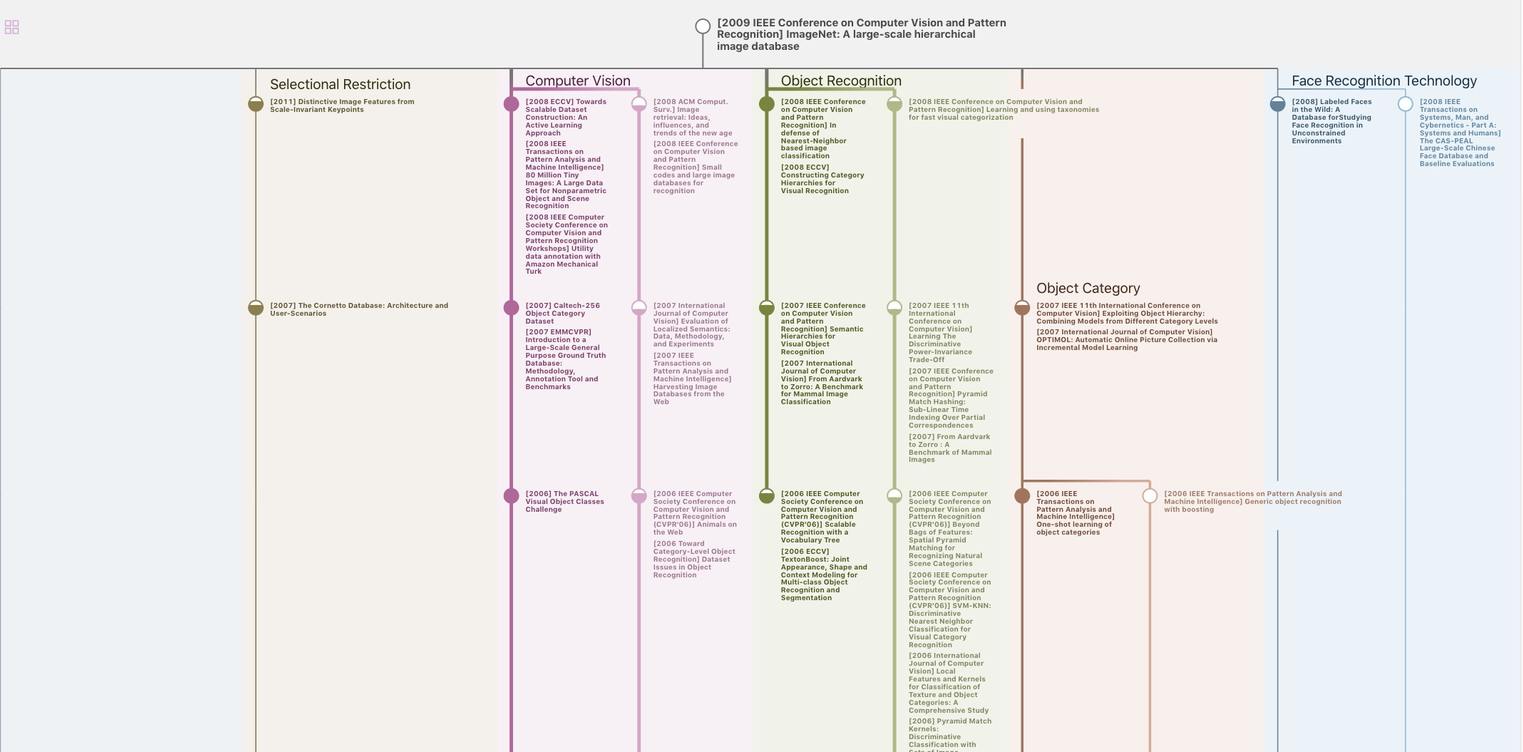
生成溯源树,研究论文发展脉络
Chat Paper
正在生成论文摘要