UFO Depth: Unsupervised learning with flow-based odometry optimization for metric depth estimation
IEEE International Conference on Robotics and Automation(2022)
摘要
We propose an efficient method for unsupervised learning of metric depth estimation from a single image in the context of unconstrained videos captured from UAVs. We combine the accuracy of an analytical solution based on odometry with the power of deep learning. First, we show how to correct the noisy odometric measurements by optimizing the alignment between the derotated optical flow and the projected linear speed in the image. Then, we detail an analytical depth estimation method based on optical flow and corrected camera velocities. Subsequently, the improved depth and camera veloc-ities obtained analytically are used, as additional cost terms, for training our novel unsupervised learning architecture for metric depth estimation. We extensively test on a recent UAV dataset, which we significantly extend by adding completely novel scenes. We outperform by significant margins different kinds of state-of-the-art approaches, ranging from analytical and unsupervised solutions to transformer-based architectures that require heavy computation and pre-training. The resulting algorithm could be deployed on embedded devices, being a good candidate for practical robotics use cases, such as obstacle avoidance and safe landing for UAV s.
更多查看译文
关键词
metric depth estimation,flow-based odometry optimization,analytical solution,deep learning,optical flow,unsupervised learning architecture,UFO depth,camera velocities,embedded devices,transformer-based architectures
AI 理解论文
溯源树
样例
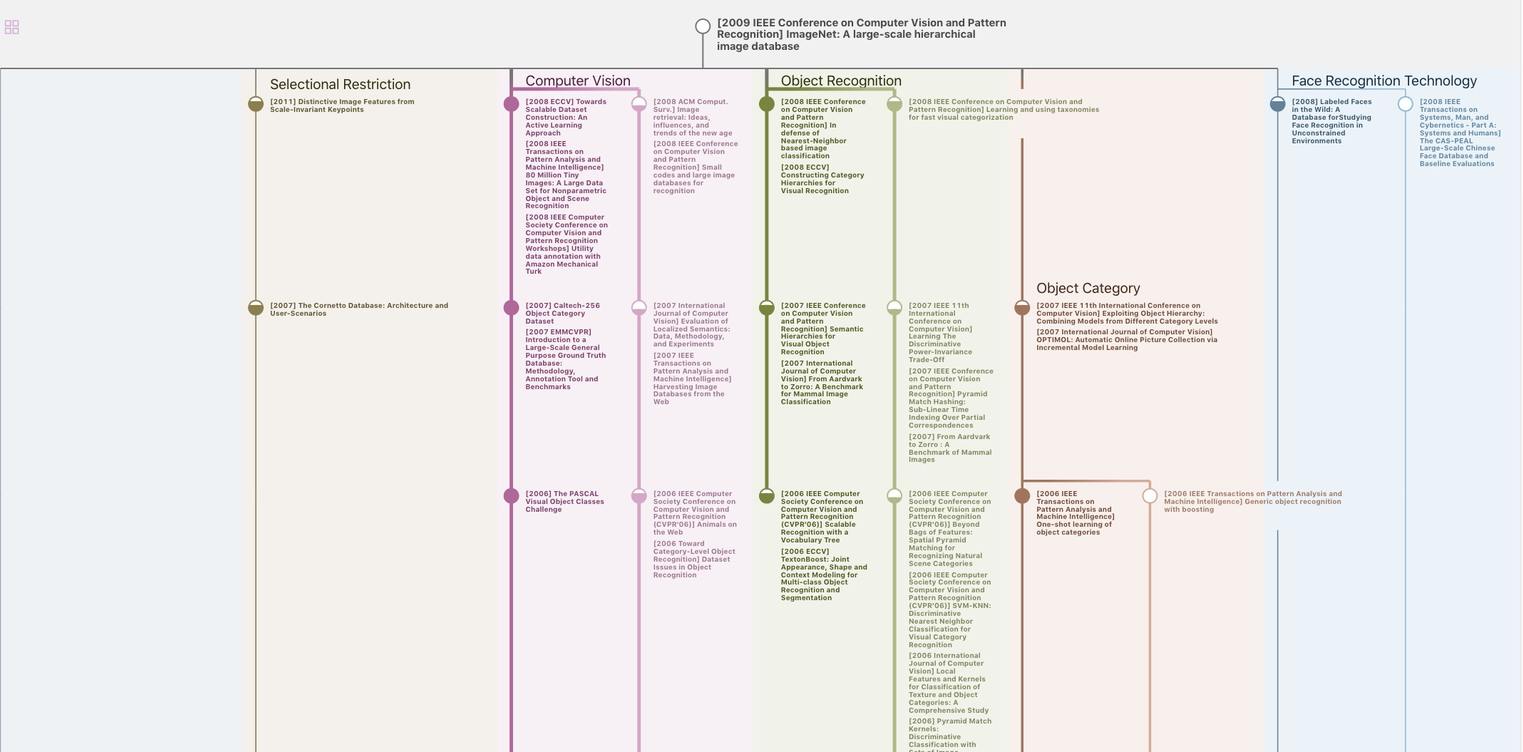
生成溯源树,研究论文发展脉络
Chat Paper
正在生成论文摘要