Remaining useful life prediction of bearings by a new reinforced memory GRU network
Advanced Engineering Informatics(2022)
摘要
The remaining useful life (RUL) prediction of bearings has great significance in the predictive maintenance of mechanical equipment. Owing to the difficulty of collecting abundant lifecycle datasets with correct labels, it is quite necessary to explore a prediction method with high precision and robustness in the case of small samples. It follows that a novel RUL prediction approach is put forward to overcome this problem. First, for reducing the man-made interference and the demand for expert knowledge, an unsupervised health indicator (HI) is constructed by Gaussian mixture model (GMM) and Kullback-Leibler divergence (KLD), which is named as KLD-based HI. Then because of the rapid forgetting of historical trend information in the current RNN-based prediction models, a novel reinforced memory gated recurrent unit (RMGRU) network is proposed by reusing the state information at the previous moment. According to the constructed KLD-based HI vector, the unknown HIs are successively predicted by RMGRU until the predicted HI value exceeds the failure threshold, and then RUL is calculated. The contrast experiment on IEEE 2012PHM bearing datasets shows the superiority of the bearing RUL prediction approach based on RMGRU over the classical time series forecasting methods. It can be concluded that this method has great application potential in bearing RUL prediction.
更多查看译文
关键词
Attention mechanism,Gated recurrent unit,Health indicator,Reinforced memory,RUL prediction
AI 理解论文
溯源树
样例
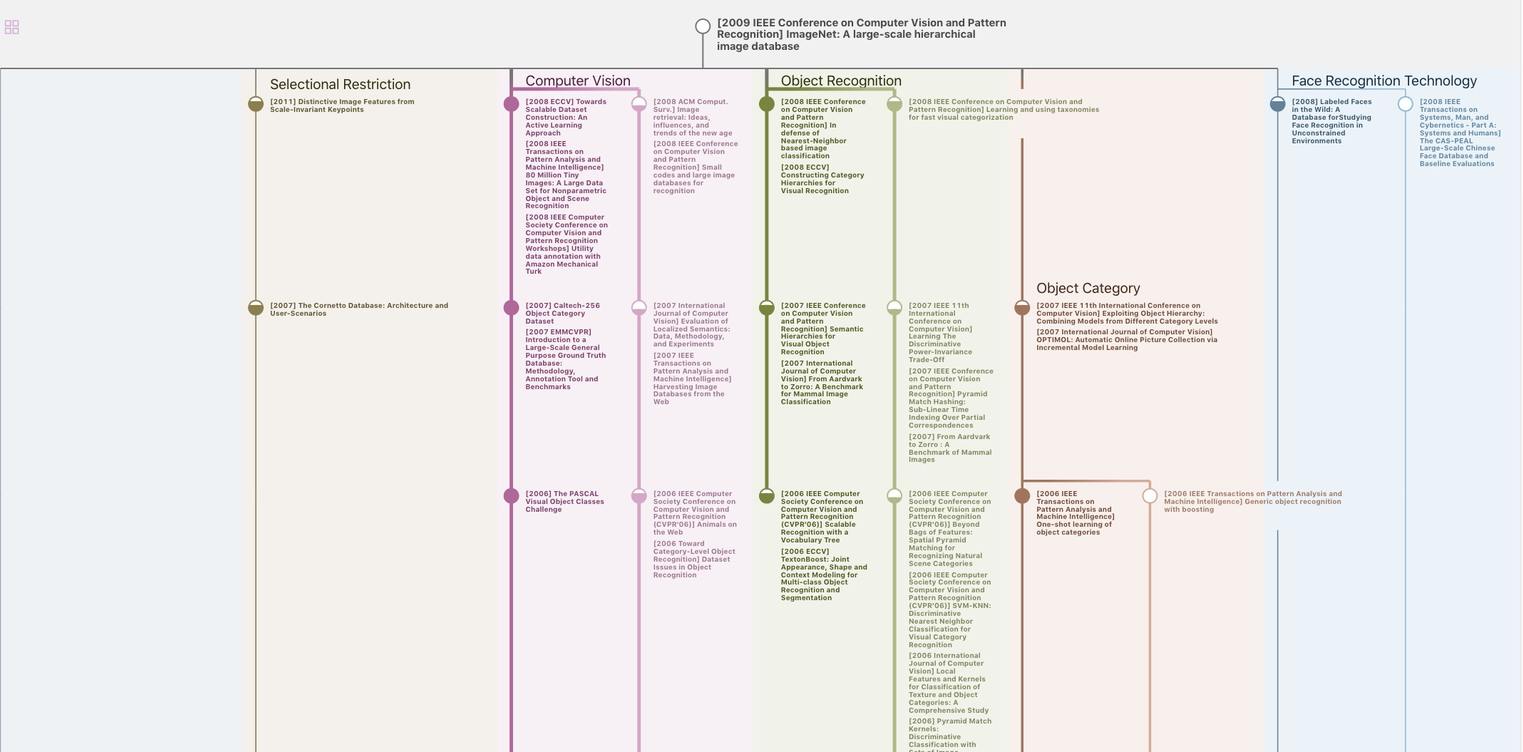
生成溯源树,研究论文发展脉络
Chat Paper
正在生成论文摘要