Neighbor-Anchoring Adversarial Graph Neural Networks (Extended Abstract)
2022 IEEE 38th International Conference on Data Engineering (ICDE)(2022)
摘要
While graph neural networks (GNNs) exhibit strong discriminative power, they often fall short of learning the underlying node distribution for increased robustness. To deal with this, inspired by generative adversarial networks (GANs), we investigate the problem of adversarial learning on graph neural networks, and propose a novel framework named NAGNN (i.e., Neighbor-anchoring Adversarial Graph Neural Networks) for graph representation learning, which trains not only a discriminator but also a generator that compete with each other. In particular, we propose a novel neighbor-anchoring strategy, where the generator produces samples with explicit features and neighborhood structures anchored on a reference real node, so that the discriminator can perform neighborhood aggregation on the fake samples to learn superior representations.
更多查看译文
关键词
Neighbor-anchoring,generative adversarial network,graph neural network
AI 理解论文
溯源树
样例
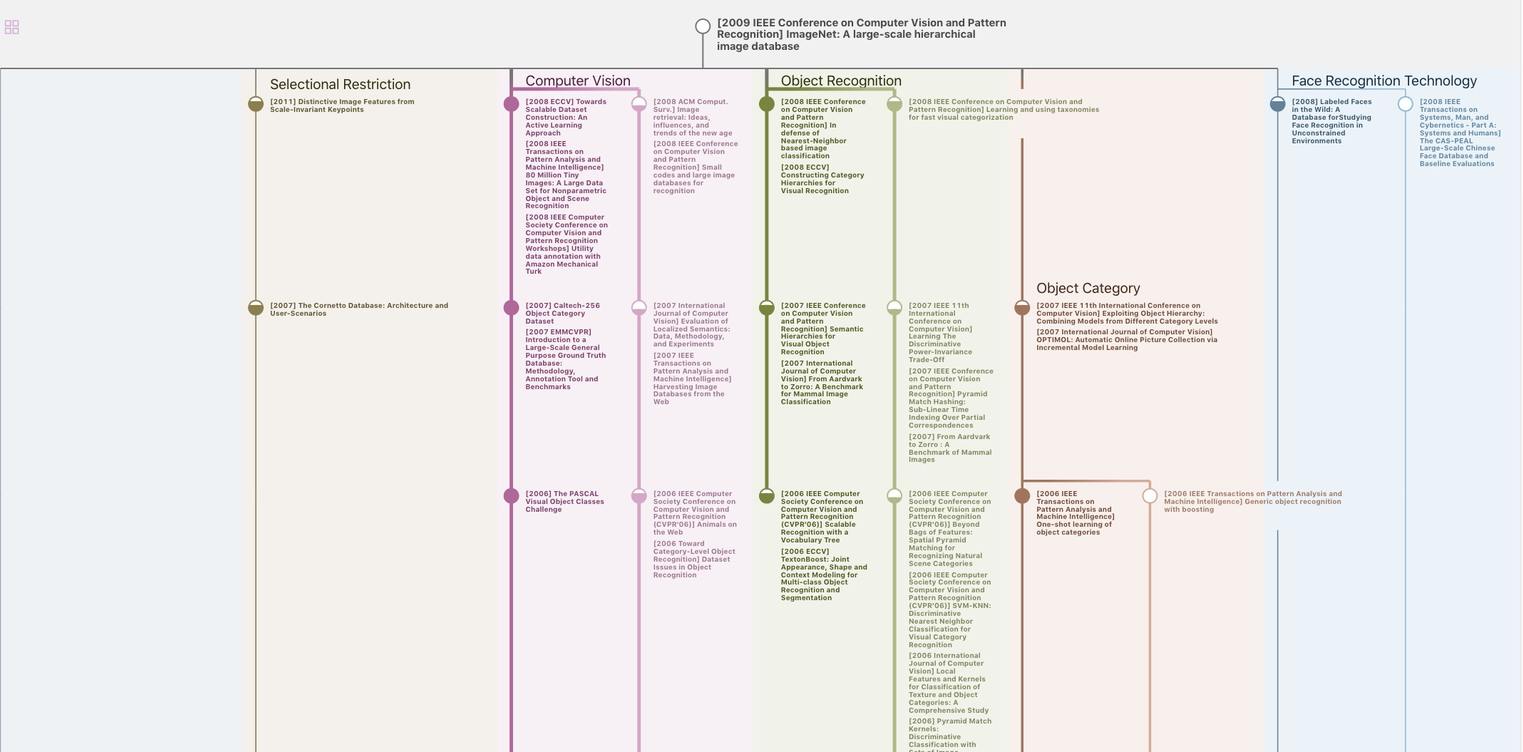
生成溯源树,研究论文发展脉络
Chat Paper
正在生成论文摘要