Area-based breast percentage density estimation in mammograms using weight-adaptive multitask learning
SCIENTIFIC REPORTS(2022)
摘要
Breast density, which is a measure of the relative amount of fibroglandular tissue within the breast area, is one of the most important breast cancer risk factors. Accurate segmentation of fibroglandular tissues and breast area is crucial for computing the breast density. Semiautomatic and fully automatic computer-aided design tools have been developed to estimate the percentage of breast density in mammograms. However, the available approaches are usually limited to specific mammogram views and are inadequate for complete delineation of the pectoral muscle. These tools also perform poorly in cases of data variability and often require an experienced radiologist to adjust the segmentation threshold for fibroglandular tissue within the breast area. This study proposes a new deep learning architecture that automatically estimates the area-based breast percentage density from mammograms using a weight-adaptive multitask learning approach. The proposed approach simultaneously segments the breast and dense tissues and further estimates the breast percentage density. We evaluate the performance of the proposed model in both segmentation and density estimation on an independent evaluation set of 7500 craniocaudal and mediolateral oblique-view mammograms from Kuopio University Hospital, Finland. The proposed multitask segmentation approach outperforms and achieves average relative improvements of 2.88
更多查看译文
关键词
Cancer,Computational biology and bioinformatics,Computer science,Risk factors,Science,Humanities and Social Sciences,multidisciplinary
AI 理解论文
溯源树
样例
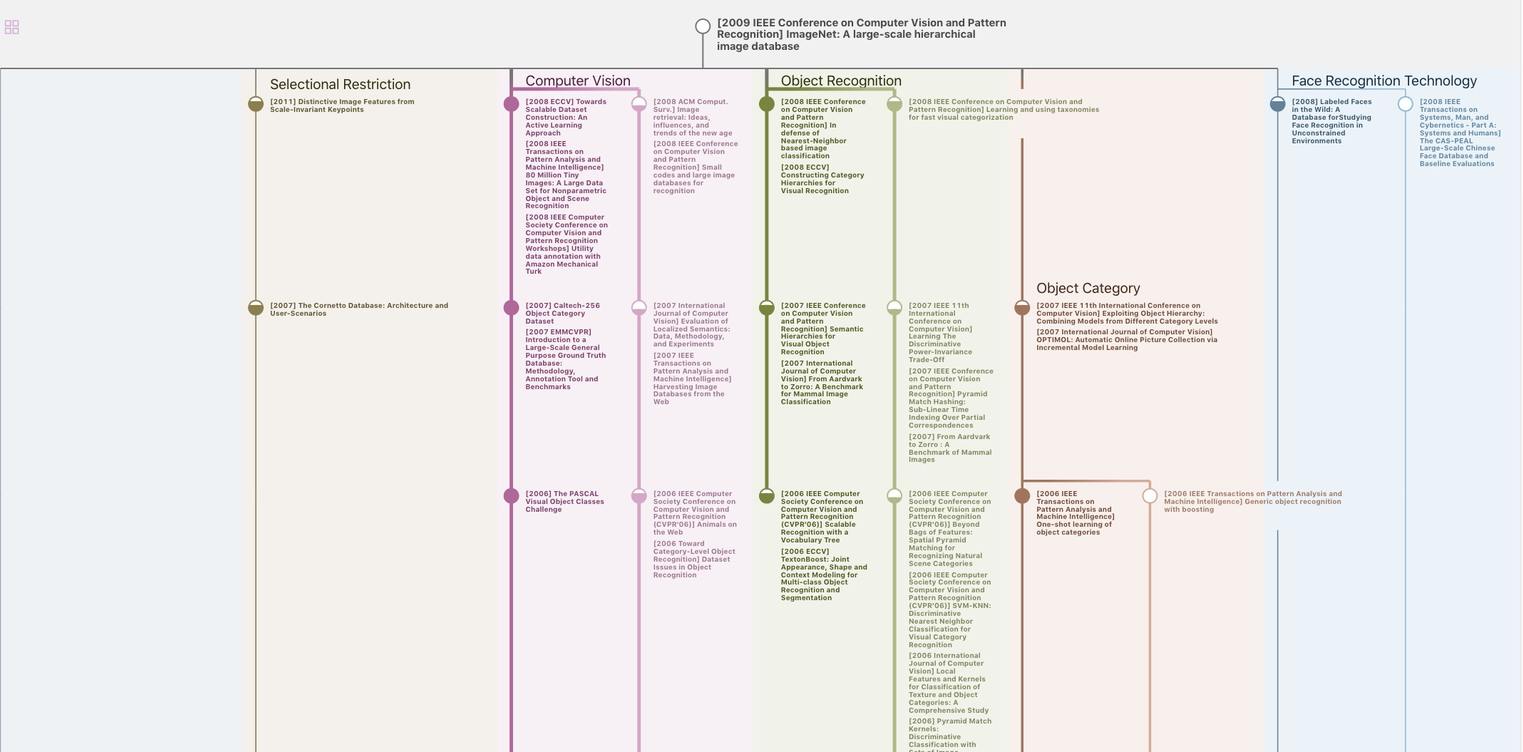
生成溯源树,研究论文发展脉络
Chat Paper
正在生成论文摘要