Nanoscale Memristor-Based Spike Timing-Dependent Plasticity Learning in a Radix-X Quantized Retinal Neural Network
PHYSICA STATUS SOLIDI A-APPLICATIONS AND MATERIALS SCIENCE(2022)
摘要
The human retina sends visual signals to the brain's visual cortex from photoreceptors (rod and cone cells) through various synaptic pathways and performs crucial early vision processing before signals are passed to higher brain regions. Herein, an artificial retina system implemented based on the leaky integrate-and-fire spiking neuron model is presented. The architecture of the proposed retina system consists of a multilayer convolutional neural network (CNN), and the system uses spike timing-dependent plasticity (STDP) as a feedforward learning rule. In addition, the system integrates a feedback plasticity learning rule to expedite learning convergence. The system weights are implemented using nanoscale memristor arrays, taking on a constrained (radix-X) range of conductance states. The proposed system produces an output image of 25 x 25 pixels, corresponding to the output retina ganglion cells that act as the interface between the retina and the visual cortex, using an input image of 100 x 100 pixels.
更多查看译文
关键词
memristors, retina, spike timing-dependent plasticity, spiking neural models
AI 理解论文
溯源树
样例
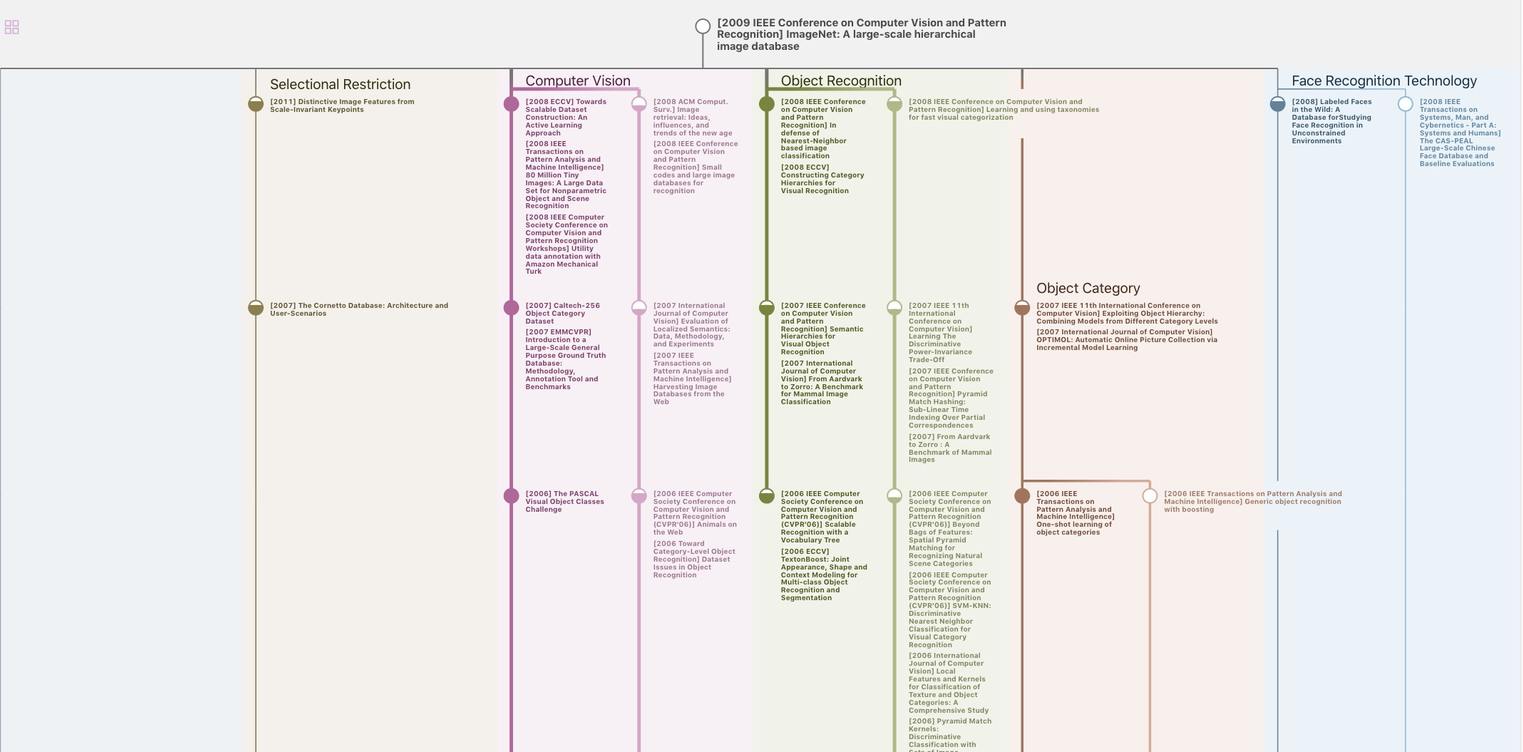
生成溯源树,研究论文发展脉络
Chat Paper
正在生成论文摘要