A Temporal-Aware Relation and Attention Network for Temporal Action Localization
IEEE TRANSACTIONS ON IMAGE PROCESSING(2022)
摘要
Temporal action localization is currently an active research topic in computer vision and machine learning due to its usage in smart surveillance. It is a challenging problem since the categories of the actions must be classified in untrimmed videos and the start and end of the actions need to be accurately found. Although many temporal action localization methods have been proposed, they require substantial amounts of computational resources for the training and inference processes. To solve these issues, in this work, a novel temporal-aware relation and attention network (abbreviated as TRA) is proposed for the temporal action localization task. TRA has an anchor-free and end-to-end architecture that fully uses temporal-aware information. Specifically, a temporal self-attention module is first designed to determine the relationship between different temporal positions, and more weight is given to features within the actions. Then, a multiple temporal aggregation module is constructed to aggregate the temporal domain information. Finally, a graph relation module is designed to obtain the aggregated graph features, which are used to refine the boundaries and classification results. Most importantly, these three modules are jointly explored in a unified framework, and temporal awareness is always fully used. Extensive experiments demonstrate that the proposed method can outperform all state-of-the-art methods on the THUMOS14 dataset with an average mAP that reaches 67.6% and obtain a comparable result on the ActivityNet1.3 dataset with an average mAP that reaches 34.4%. Compared with A2Net (TIP20), PCG-TAL (TIP21), and AFSD (CVPR21) TRA can achieve improvements of 11.7%, 4.4%, and 1.8%, respectively on the THUMOS14 dataset.
更多查看译文
关键词
Proposals, Feature extraction, Task analysis, Location awareness, Network architecture, Convolution, Aggregates, Temporal-aware relation and attention network, temporal self-attention module, multiple temporal aggregation module, graph relation module, temporal action localization
AI 理解论文
溯源树
样例
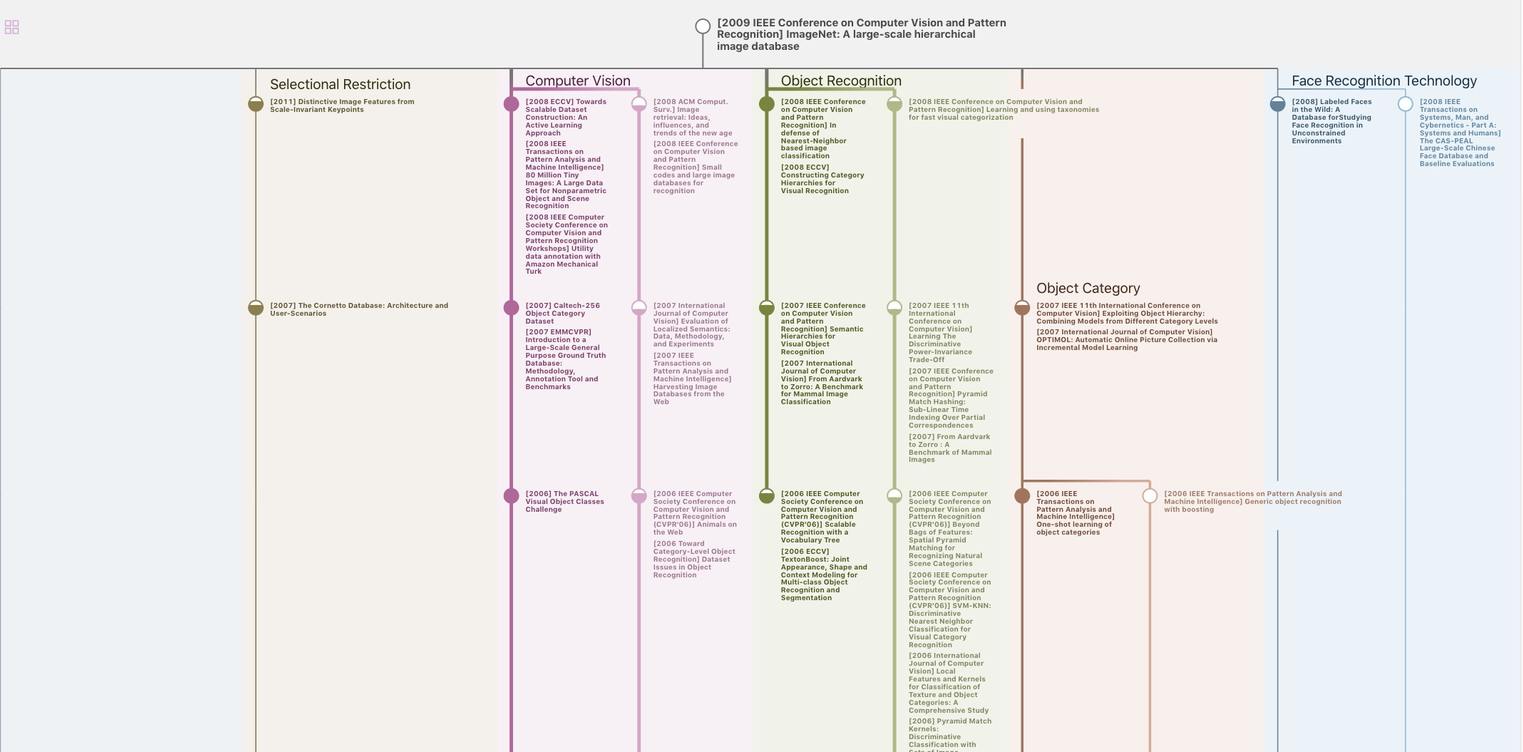
生成溯源树,研究论文发展脉络
Chat Paper
正在生成论文摘要