Impartial Differentiable Automatic Data Augmentation Based on Finite Difference Approximation for Pedestrian Detection
IEEE TRANSACTIONS ON INSTRUMENTATION AND MEASUREMENT(2022)
摘要
Pedestrian detection is a challenging task in practical scenarios. Data augmentation is an effective way to deal with this problem, but designing a suitable data augmentation policy requires a great deal of manual experience and multiple adjustments of parameters. To address this problem, we propose an impartial differentiable automatic data augmentation (IDADA) that automatically searches a suitable augmentation policy for pedestrian detection in a more practical scenario. First, an impartiality loss is proposed to solve the bias of the operations probabilities distribution, so as to improve the impartiality for each operation. Second, the impartiality loss and the original validation loss of the detection model are combined as a target function to update the augmentation policy parameters. Since the target function is not differentiable, the finite difference approximation is applied to provide accurate gradient estimation for the augmentation policy optimization process. Comprehensive experiments performed on two typical datasets of CrowdHuman and CityPersons verify the effectiveness of the proposed approach. Specifically, our approach achieves 40.99% in miss rate (MR) on CrowdHuman, yielding the new state-of-the-art result on this dataset.
更多查看译文
关键词
Optimization,Training,Estimation,Task analysis,Finite difference methods,Search problems,Encoding,Automatic data augmentation,differentiable optimization,pedestrian detection
AI 理解论文
溯源树
样例
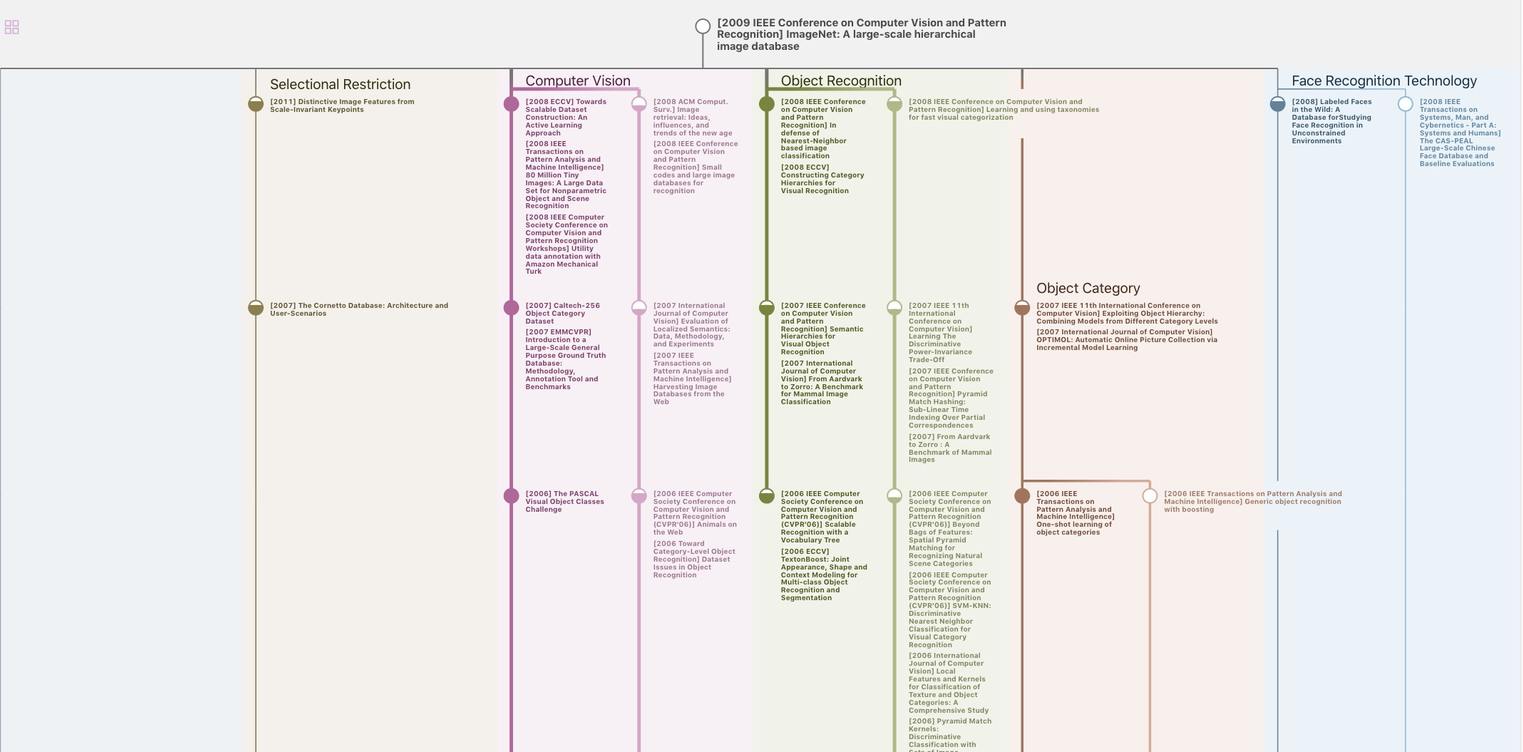
生成溯源树,研究论文发展脉络
Chat Paper
正在生成论文摘要