A deep learning approach utilizing clinical and molecular data for identifying prognostic biomarkers in patients treated with immune checkpoint inhibitors: An ORIEN pan-cancer study.
Journal of Clinical Oncology(2022)
摘要
2619 Background: Immune checkpoint inhibitors (ICIs) have made significant improvements in the treatment of cancer patients (pts), but many continue to experience primary or secondary resistance. Here, we leveraged clinical and genomic data to identify prognostic biomarkers in pts treated with ICIs utilizing a pan-cancer approach. Methods: Pts were enrolled to the Total Cancer Care protocol across 18 cancer centers within the Oncology Research Information Exchange Network (ORIEN). RNA-seq was performed on tumors following the RSEM pipeline and gene expressions were quantified as Transcript Per Million (TPM) and were logarithmically normalized. An Auto-Encoder Survival Deep Network (AE-SDN) architecture was developed that combined the reconstruction loss of AE with Cox regression for modeling time to event. For comparison, immunoscore for each pt was calculated based on the estimated densities of tumor CD3+ and CD8+ T cells (Galon, 2020) utilizing CIBERSORTx. The quality of overall survival (OS) predictions was assessed using Harrell’s concordance index (C-index). Log-rank test was used to assess stratified group differences (by ICI or cancer histology) along with Kaplan-Meier (KM) survival analysis of AE-SDN and immunoscore. Results: Pts (n=522) with 4 cancer types including melanoma (n=125), renal cell carcinoma (n=149), non-small cell lung cancer (n=128) and head and neck cancer (n=120) treated with 6 ICI regimens were included in this analysis. ICI regimens were nivolumab (n=219), pembrolizumab (n=202), ipilimumab+nivolumab (n=69), ipilimumab (n=30), avelumab (n=1) and cemiplimab (n=1). The Table summarizes the overall C-index and associated 95% CIs and log-rank P values for the entire cohort (regardless of histology) resulting from our proposed AE-SDN model and the separate estimated immunoscore categorization. AE-SDN top selected genes were mostly related to immunity, carcinogenesis and tumor suppression. The corresponding KM plots showed significantly wider separations of the survival curves in favor of our proposed AE-SDN model relative to the immunoscore with more than 20% improvement in prediction power. Conclusions: Deep network machine learning analysis is a promising approach to identifying relevant prognostic biomarkers in cancer pts treated with ICI. This may lead to novel therapeutic predictive signatures and identification of mechanisms of ICI resistance. Our AE-SDN gene expression signature was significantly prognostic and outperformed the estimated CD3+, CD8+ T Cell immunoscore. Further refinements to our prediction power are ongoing along with more advanced neural network architectures to elucidate related functional pathways. [Table: see text]
更多查看译文
AI 理解论文
溯源树
样例
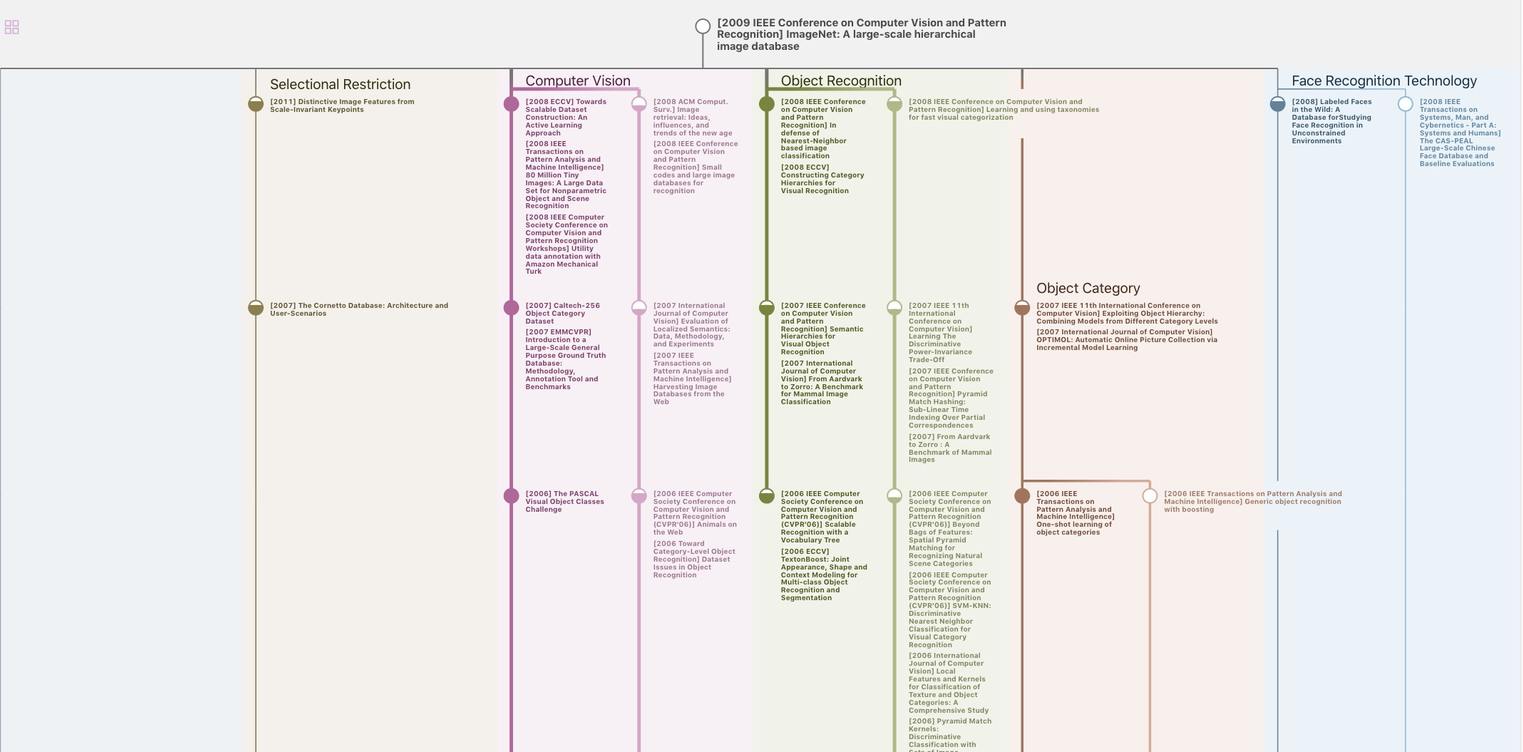
生成溯源树,研究论文发展脉络
Chat Paper
正在生成论文摘要