Colonoscopy Navigation using End-to-End Deep Visuomotor Control: A User Study.
IEEE/RJS International Conference on Intelligent RObots and Systems (IROS)(2022)
摘要
Flexible endoscopes for colonoscopy present several limitations due to their inherent complexity, resulting in patient discomfort and lack of intuitiveness for clinicians. Robotic devices together with autonomous control represent a viable solution to reduce the workload of endoscopists and the training time while improving the overall procedure outcome. Prior works on autonomous endoscope control use heuristic policies that limit their generalisation to the unstructured and highly deformable colon environment and require frequent human intervention. This work proposes an image-based control of the endoscope using Deep Reinforcement Learning, called Deep Visuomotor Control (DVC), to exhibit adaptive behaviour in convoluted sections of the colon tract. DVC learns a mapping between the endoscopic images and the control signal of the endoscope. A first user study of 20 expert gastrointestinal endoscopists was carried out to compare their navigation performance with DVC policies using a realistic virtual simulator. The results indicate that DVC shows equivalent performance on several assessment parameters, being more safer. Moreover, a second user study with 20 novice participants was performed to demonstrate easier human supervision compared to a state-of-the-art heuristic control policy. Seamless supervision of colonoscopy procedures would enable interventionists to focus on the medical decision rather than on the control problem of the endoscope.
更多查看译文
关键词
colonoscopy navigation,colonoscopy procedures,deep reinforcement learning,deep visuomotor control,DVC,FE control signal,flexible endoscopes,gastrointestinal endoscopists,heuristic control policy,highly deformable colon environment,image-based FE control,navigation performance,patient discomfort,robotic FEs
AI 理解论文
溯源树
样例
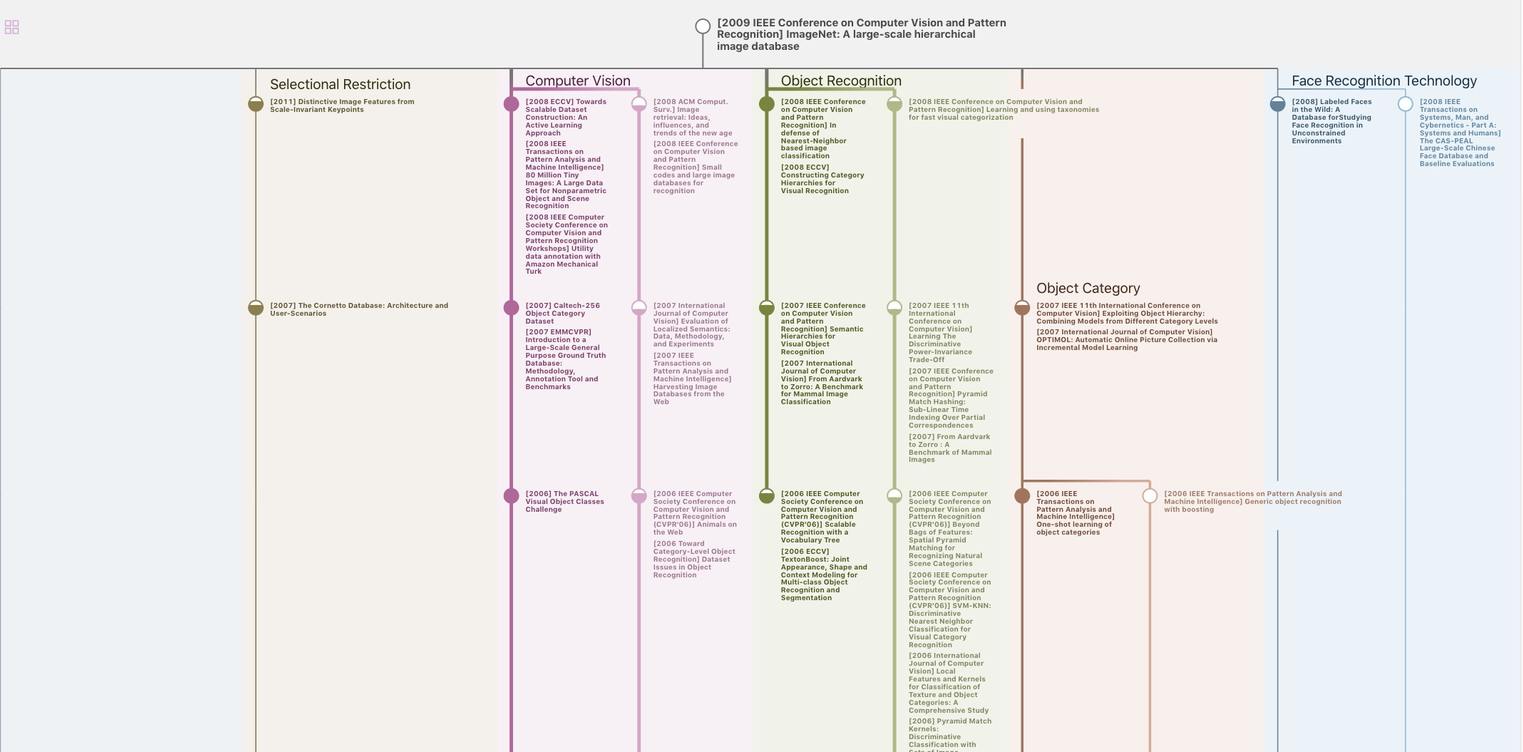
生成溯源树,研究论文发展脉络
Chat Paper
正在生成论文摘要