KCRL: Krasovskii-Constrained Reinforcement Learning with Guaranteed Stability in Nonlinear Dynamical Systems
arxiv(2022)
摘要
Learning a dynamical system requires stabilizing the unknown dynamics to avoid state blow-ups. However, current reinforcement learning (RL) methods lack stabilization guarantees, which limits their applicability for the control of safety-critical systems. We propose a model-based RL framework with formal stability guarantees, Krasovskii Constrained RL (KCRL), that adopts Krasovskii's family of Lyapunov functions as a stability constraint. The proposed method learns the system dynamics up to a confidence interval using feature representation, e.g. Random Fourier Features. It then solves a constrained policy optimization problem with a stability constraint based on Krasovskii's method using a primal-dual approach to recover a stabilizing policy. We show that KCRL is guaranteed to learn a stabilizing policy in a finite number of interactions with the underlying unknown system. We also derive the sample complexity upper bound for stabilization of unknown nonlinear dynamical systems via the KCRL framework.
更多查看译文
关键词
nonlinear dynamical systems,dynamical systems,krasovskii-constrained
AI 理解论文
溯源树
样例
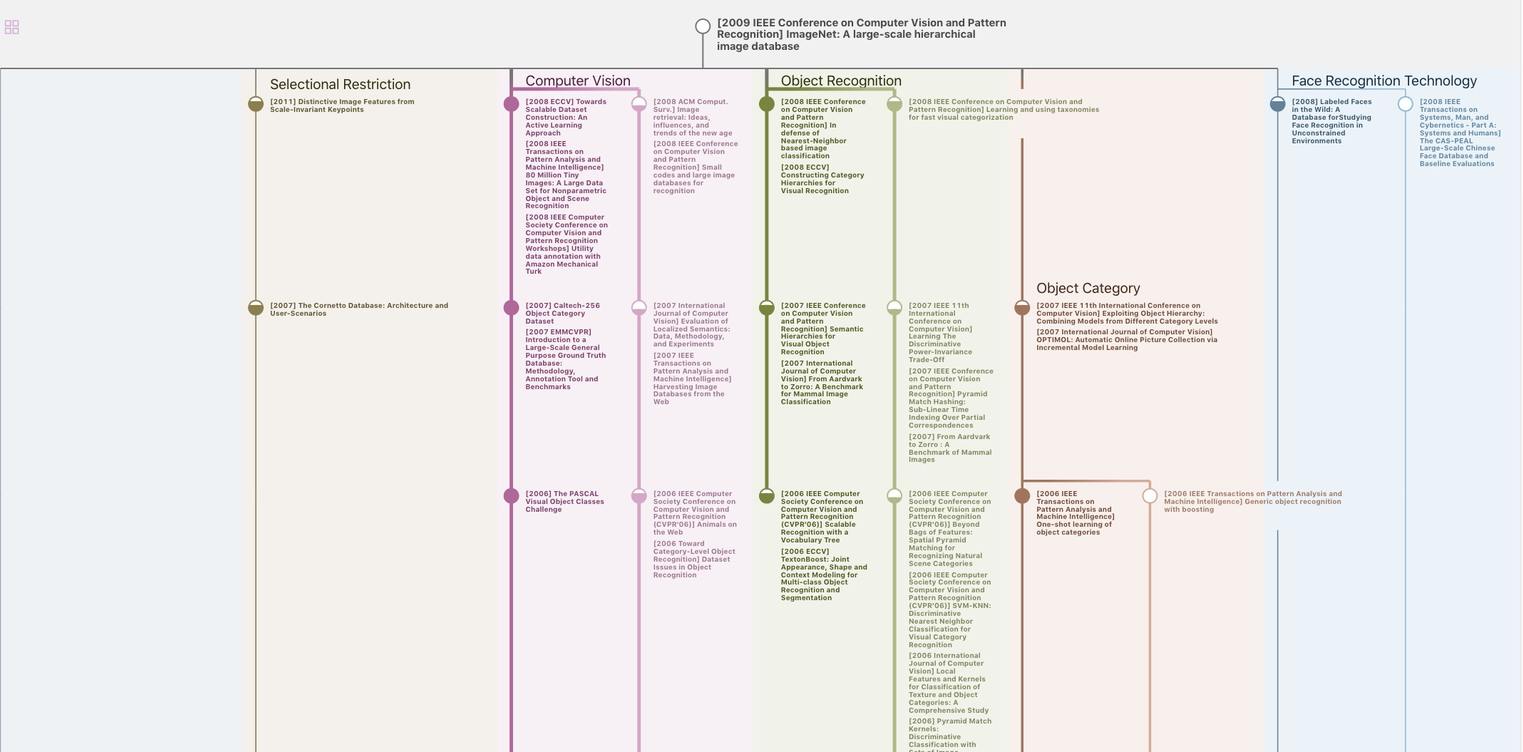
生成溯源树,研究论文发展脉络
Chat Paper
正在生成论文摘要