Causal Explanations for Sequential Decision Making Under Uncertainty
arxiv(2023)
摘要
We introduce a novel framework for causal explanations of stochastic, sequential decision-making systems built on the well-studied structural causal model paradigm for causal reasoning. This single framework can identify multiple, semantically distinct explanations for agent actions -- something not previously possible. In this paper, we establish exact methods and several approximation techniques for causal inference on Markov decision processes using this framework, followed by results on the applicability of the exact methods and some run time bounds. We discuss several scenarios that illustrate the framework's flexibility and the results of experiments with human subjects that confirm the benefits of this approach.
更多查看译文
关键词
sequential decision making,causal,uncertainty,decision making
AI 理解论文
溯源树
样例
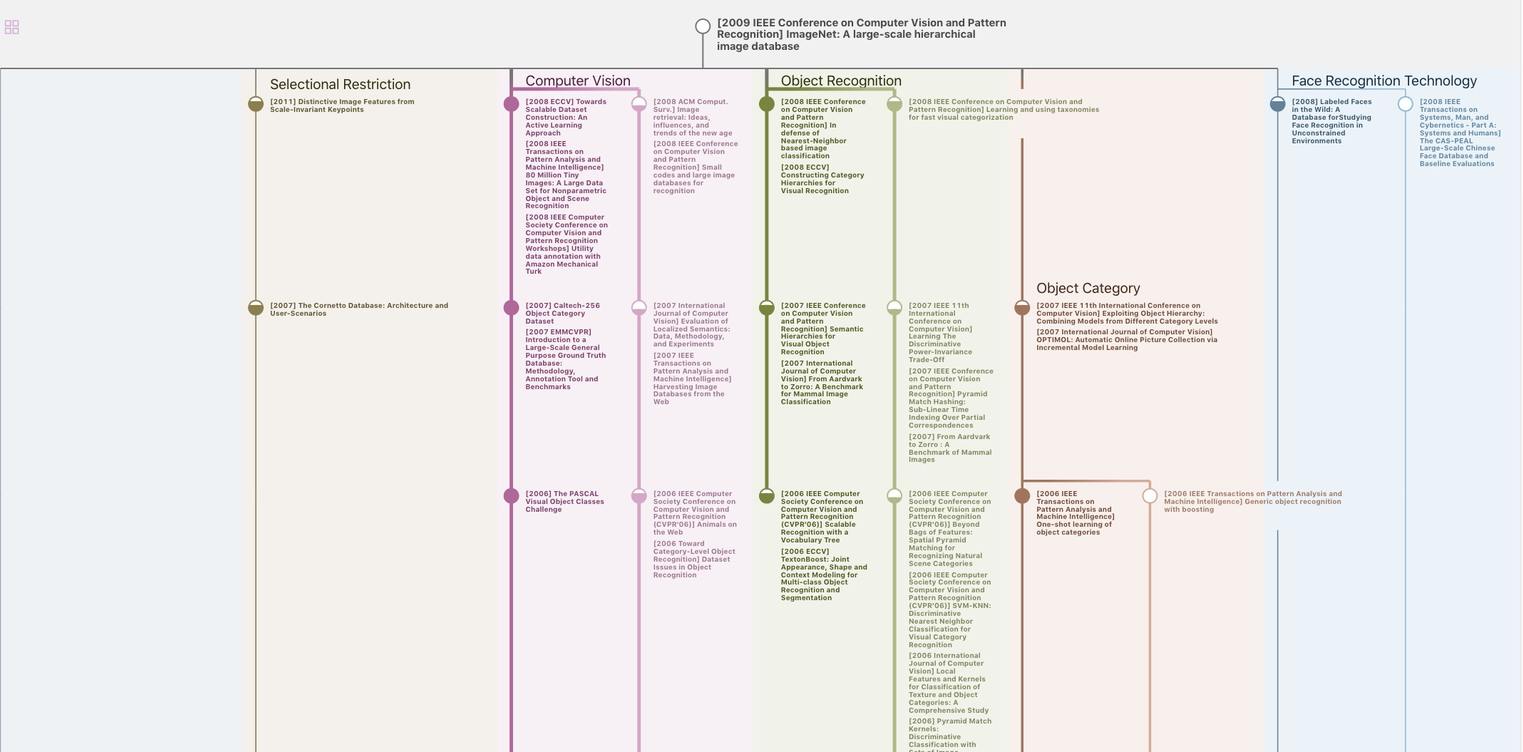
生成溯源树,研究论文发展脉络
Chat Paper
正在生成论文摘要